Creation of a structured solar cell material dataset and performance prediction using large language models
Patterns(2024)
摘要
Materials scientists usually collect experimental data to summarize experiences and predict improved materials. However, a crucial issue is how to proficiently utilize unstructured data to update existing structured data, particularly in applied disciplines. This study introduces a new natural language processing (NLP) task called structured information inference (SII) to address this problem. We propose an end-to-end approach to summarize and organize the multi-layered device-level information from the literature into structured data. After comparing different methods, we fine-tuned LLaMA with an F1 score of 87.14% to update an existing perovskite solar cell dataset with articles published since its release, allowing its direct use in subsequent data analysis. Using structured information, we developed regression tasks to predict the electrical performance of solar cells. Our results demonstrate comparable performance to traditional machine-learning methods without feature selection and highlight the potential of large language models for scientific knowledge acquisition and material development.
更多查看译文
关键词
AI for science,materials science,theory and computation,renewable energy,large language model,text mining,scientific data,automated data annotation,material discovery,device performance prediction,perovskite solar cells
AI 理解论文
溯源树
样例
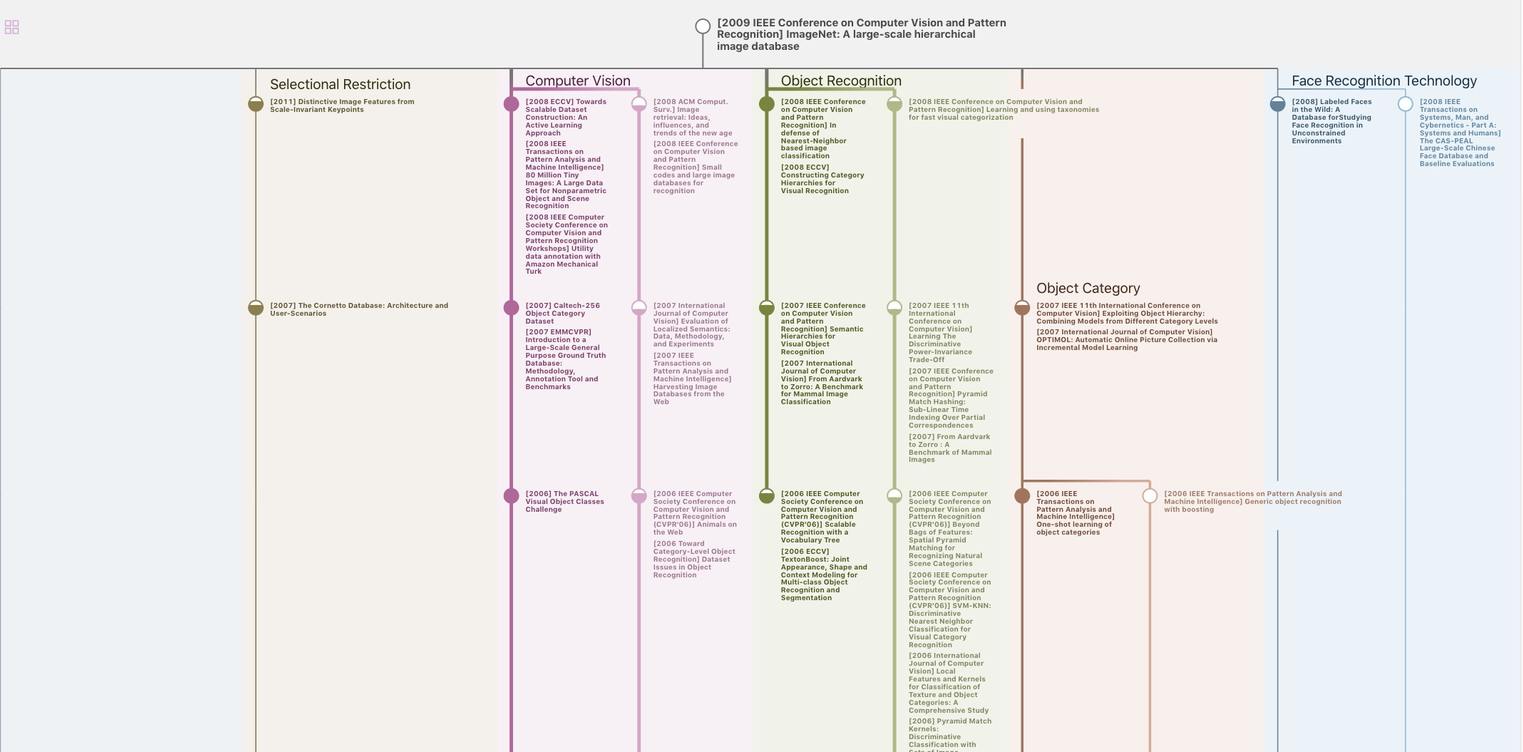
生成溯源树,研究论文发展脉络
Chat Paper
正在生成论文摘要