Power data quality assessment and verification governance based on knowledge graph
Intelligent Decision Technologies(2024)
摘要
In addressing the challenges of scattered data and limited professional knowledge in traditional power data quality assessment and verification governance, our approach leveraged natural language processing (NLP) technology for text preprocessing, incorporating power system research findings and case analyses. Named entity recognition enhanced entity identification accuracy, while relationship weighting technology facilitated entity classification and relationship weight assignment. The resulting power system knowledge graph was seamlessly integrated into a graph database for real-time updates. Through the synergistic use of relationship weighting technology and graph convolutional networks (GCN), our method achieved precise representation and modeling of power system knowledge graphs. The research outcomes underscored the method’s exceptional performance in real-time anomaly detection, maintaining an anomaly detection rate between 1% and 10% and accuracy fluctuating from 80% to 98%. This method stands as a testament to its efficacy in processing power data and validating its robustness in power system assessment and verification.
更多查看译文
AI 理解论文
溯源树
样例
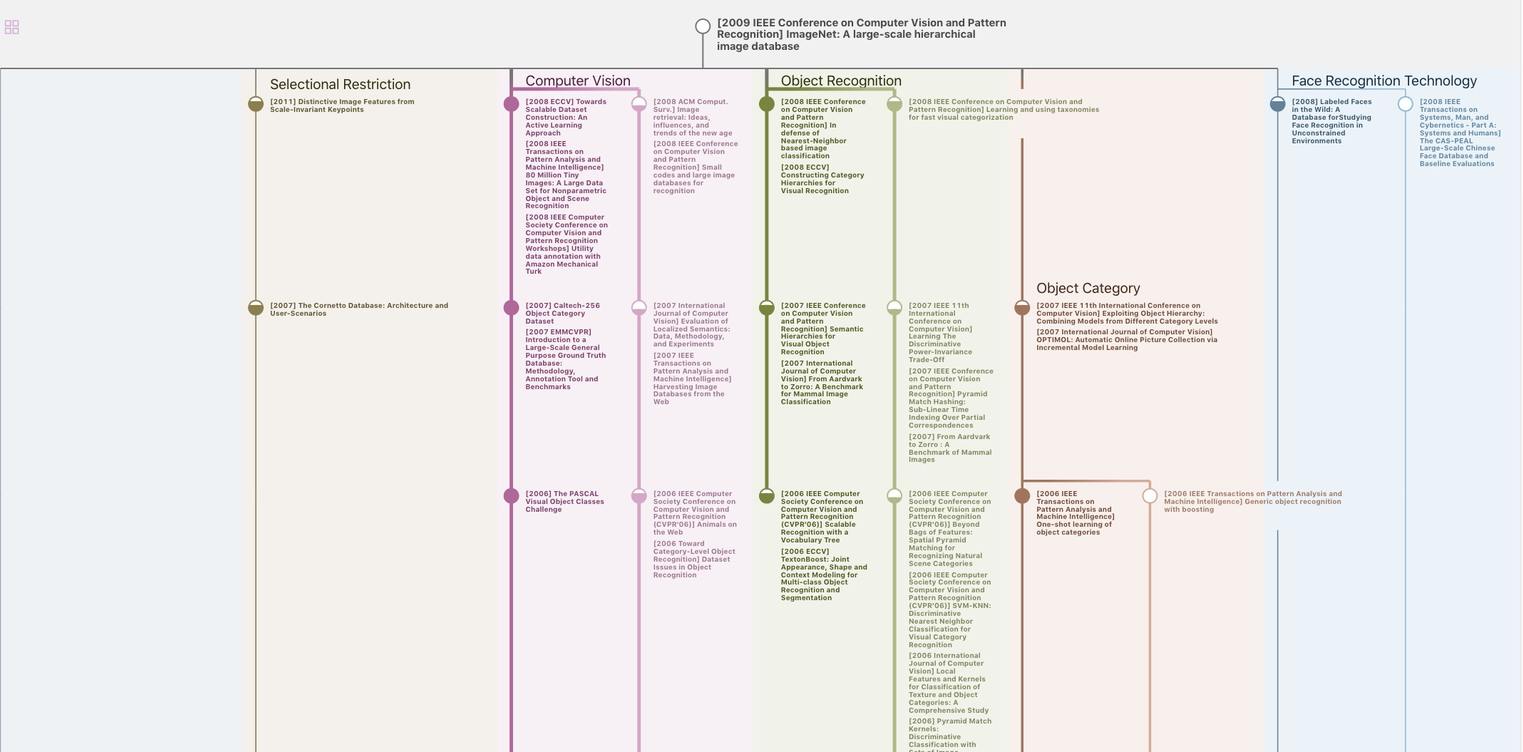
生成溯源树,研究论文发展脉络
Chat Paper
正在生成论文摘要