A Machine Learning Initializer for Newton-Raphson AC Power Flow Convergence
2024 IEEE Texas Power and Energy Conference (TPEC)(2024)
摘要
Power flow computations are fundamental to many power system studies. Obtaining a converged power flow case is not a trivial task especially in large power grids due to the non-linear nature of the power flow equations. One key challenge is that the widely used Newton based power flow methods are sensitive to the initial voltage magnitude and angle estimates, and a bad initial estimate would lead to non-convergence. This paper addresses this challenge by developing a random-forest (RF) machine learning model to provide better initial voltage magnitude and angle estimates towards achieving power flow convergence. This method was implemented on a real ERCOT 6102 bus system under various operating conditions. By providing better Newton-Raphson initialization, the RF model precipitated the solution of 2,106 cases out of 3,899 non-converging dispatches. These cases could not be solved from flat start or by initialization with the voltage solution of a reference case. Results obtained from the RF initializer performed better when compared with DC power flow initialization, Linear regression, and Decision Trees.
更多查看译文
关键词
Machine Learning,Newton Raphson,Power flow convergence,Power flow initialization,Random Forest
AI 理解论文
溯源树
样例
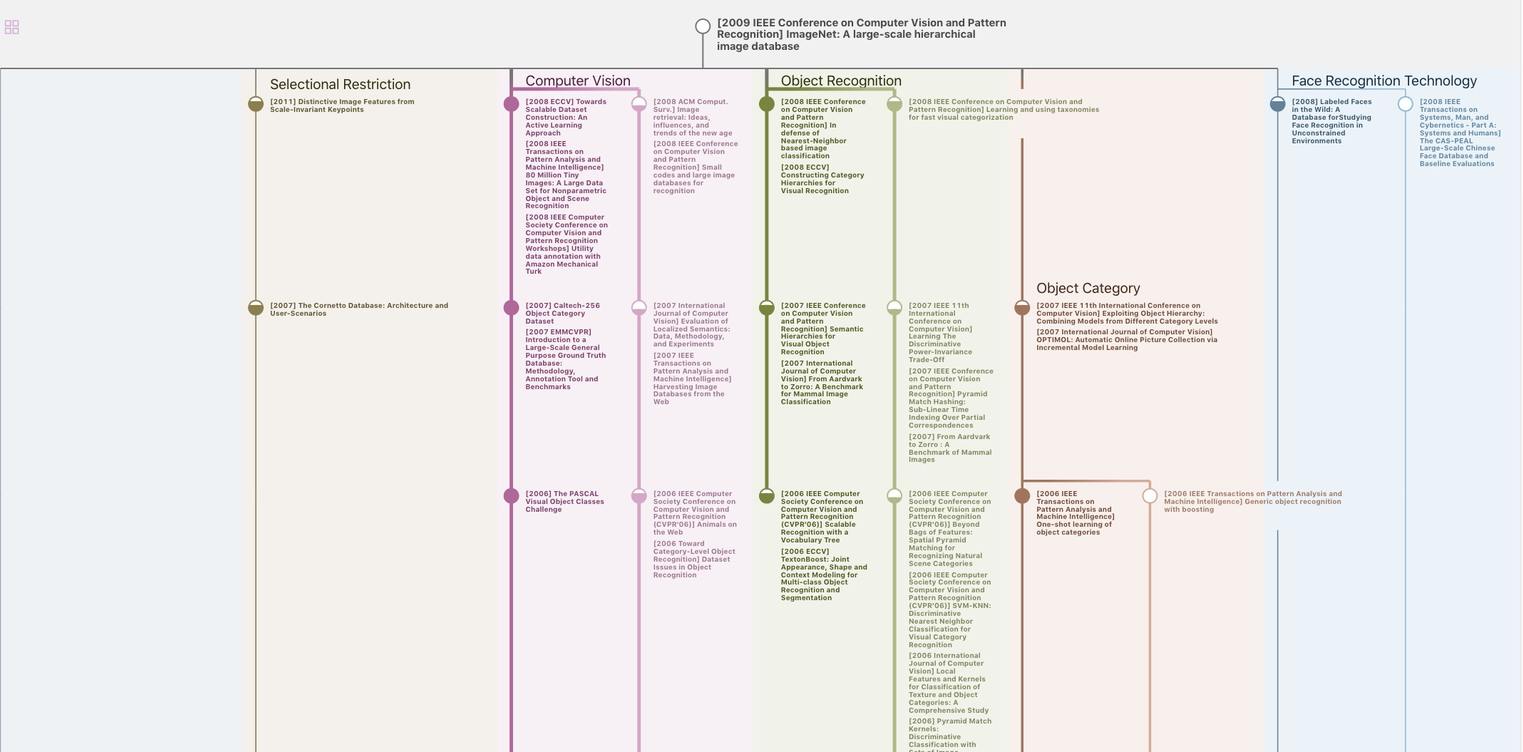
生成溯源树,研究论文发展脉络
Chat Paper
正在生成论文摘要