Abstract 2933: Use of artificial intelligence to facilitate reporting of comprehensive genomic profiling tests
Cancer Research(2024)
摘要
Abstract Background: Comprehensive genomic profiling (CGP) tests have been nationally reimbursed in Japan since 2019, and all test results must be discussed by molecular tumor boards (MTBs). More than 60,000 patients have taken the CGP tests at over 200 government-designated hospitals by November 2023. Streamlining decision-making by MTBs and standardizing interpretation of test results is important. We evaluated the performance of Chrovis, an annotation algorithm for reporting CGP tests. Methods: We retrospectively reviewed the reporting process of all approved CGP tests done at The University of Tokyo Hospital and its 12 affiliated hospitals between December 2019 and November 2023. Chrovis referenced several public knowledge databases and used natural language processing to annotate each genetic variant and generated reports using the automated program. The MTB reviewed all reports with the addition of clinical information and made any necessary changes Recommendations on disclosure of germline findings were made in accordance with the national guideline. Results: Among the 2,819 Chrovis reports created, 1,927 were tumor-only FoundationOne CDx (F1CDx), 483 were tumor/normal paired NCC Oncopanel (NOP), and 409 were FoundationOne Liquid CDx (F1Liquid) panels. Among them, 603 changes in 496 reports (17.6% of all reports) were made by the MTB. The most common type of change was germline disclosure with 208 changes (7.4%), followed by clinical trial information (196 changes, 7.0%) and pathogenicity (107 changes, 3.8%). Gene alterations that led to most changes in germline disclosure were TP53, SMAD4, CDKN2A, TERT, and NF2 (42, 22, 16, 13, and 13 changes, respectively). Changes in clinical trial information were frequently observed in TP53, NF1, BRAF, ERBB2, and KRAS (25, 20, 19, 16, and 16 changes, respectively). F1CDx required the greatest number of changes (404/1927, 21.0%) followed by F1Liquid (53/409, 13.0%) and NOP (39/483, 8.1%) (p < 0.001). Changes to germline disclosure were made in 9.1% of F1CDx reports, 5.4% of F1Liquid reports, and 2.1% of NOP reports (p < 0.001). Changes to clinical trial information were made in 8.3%, 4.4%, and 3.9%, respectively (p < 0.001). Conclusions: Chrovis-generated CGP reports did not require any changes in 82%s, suggesting that artificial intelligence reporting may lessen the burden of MTBs while contributing to its standardization. Recommendation for germline disclosure requires manual supervision, and improvements in clinical trial databases are required to correctly recommend genomically matched trials to patients. Citation Format: Hidenori Kage, Takashi Aoki, Aya Shinozaki-Ushiku, Kousuke Watanabe, Nana Akiyama, Hideaki Isago, Miho Ogawa, Kazunaga Ishigaki, Hiroshi Kobayashi, Takuya Miyagawa, Jun Omatsu, Yuki Saito, Kazuhito Sasaki, Yasuyoshi Sato, Yusuke Sato, Nobumi Suzuki, Shota Tanaka, Motohiro Kato, Masahiko Tanabe, Kenji Tatsuno, Tetsuo Ushiku, Kunihiro Nishimura, Hiroyuki Aburatani, Katsutoshi Oda. Use of artificial intelligence to facilitate reporting of comprehensive genomic profiling tests [abstract]. In: Proceedings of the American Association for Cancer Research Annual Meeting 2024; Part 1 (Regular Abstracts); 2024 Apr 5-10; San Diego, CA. Philadelphia (PA): AACR; Cancer Res 2024;84(6_Suppl):Abstract nr 2933.
更多查看译文
AI 理解论文
溯源树
样例
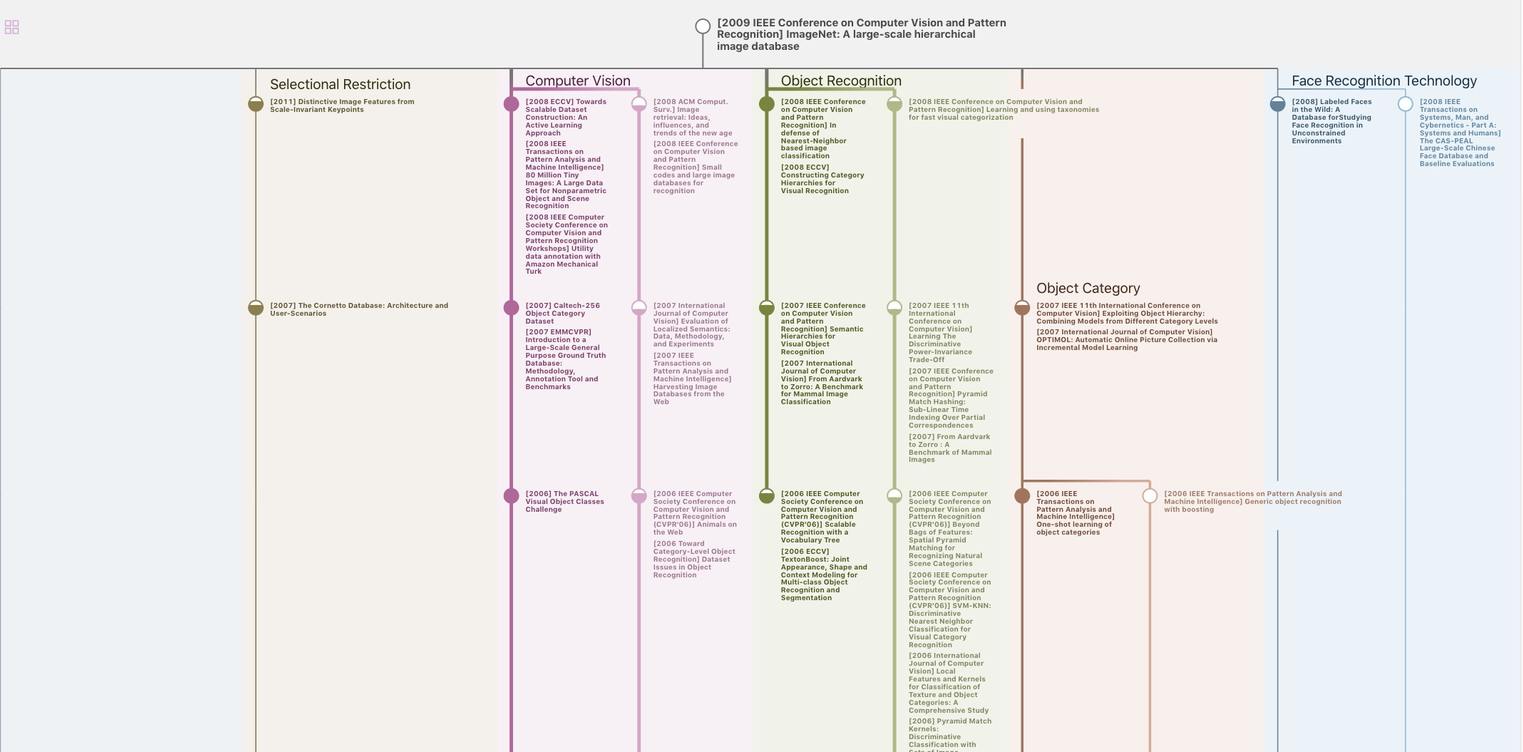
生成溯源树,研究论文发展脉络
Chat Paper
正在生成论文摘要