Abstract 1750: Decoding breast cancer: Unraveling subtype and model differences through multi-model single-cell RNA sequencing data integration
Cancer Research(2024)
摘要
Abstract Breast cancer's complex transcriptional landscape requires a deep understanding of sample and cell diversity to identify effective treatments. In this study, we amalgamate single-cell RNA sequencing data from breast cancer patient-derived xenografts (PDX), organoids, cell lines, patient tumors and reduction mammoplasties resulting in a comprehensive dataset of 117 samples with 506,719 total cells. These samples encompass hormone receptor positive (HR+), human epidermal growth factor receptor 2 enriched (HER2E), and triple-negative breast cancer (TNBC) subtypes. We aimed to delineate similarities and distinctions across model systems and patient samples while also exploring stratification of therapeutic drug efficacy based on subtype proportions within tumors. Mammary tumor PDXs, organoids, and established cell lines exhibited higher proliferation and lower heterogeneity observed via UMAP dimensionality reduction compared to most patient tumors or normal breast epithelium. TNBCs had elevated proliferative and pro-metastatic signatures compared to HR+ and HER2E samples. Interestingly, compared to matched PDX tumors, organoids from these same models were found to exhibit stark differences in gene expression, including upregulation of metabolically active aldo-keto reductase family genes, highlighting differences in the model systems with implications for pre-clinical drug testing. Single-cell tumor subtyping analyses with scSubtype and TNBCtype methods found that therapeutically treated samples had shifts in the proportions of cell-wise subtype annotations when compared to matched untreated samples. Similarly, patient lymph node metastasis when compared with matched primary tumors were significantly linked to decreases in Basal-like and HER2-enriched cell-wise annotations in untreated ER+ samples. In vitro assessment of anti-cancer compounds on PDX cells showed significant correlation of subtype proportion with cell viability following treatment with targeted therapeutic agents. This subtyping methodology offers a powerful tool to monitor the evolving gene expression landscape within samples and predict responses to therapeutic agents. We present here a dynamic approach to cell-wise sample annotation and a substantial multi-model dataset for use facilitating informed decision-making in preclinical research and therapeutic development. Citation Format: Julia E. Altman, Carson J. Walker, Emily K. Zboril, Nicole S. Hairr, Rachel K. Myrick, David C. Boyd, Jennifer E. Koblenski, Madhavi Puchalapalli, Bin Hu, Mikhail G. Dozmorov, Xi Chen, Yunshun Chen, Charles M. Perou, Brian D. Lehmann, Jane E. Visvader, Amy L. Olex, J. Chuck Harrell. Decoding breast cancer: Unraveling subtype and model differences through multi-model single-cell RNA sequencing data integration [abstract]. In: Proceedings of the American Association for Cancer Research Annual Meeting 2024; Part 1 (Regular Abstracts); 2024 Apr 5-10; San Diego, CA. Philadelphia (PA): AACR; Cancer Res 2024;84(6_Suppl):Abstract nr 1750.
更多查看译文
AI 理解论文
溯源树
样例
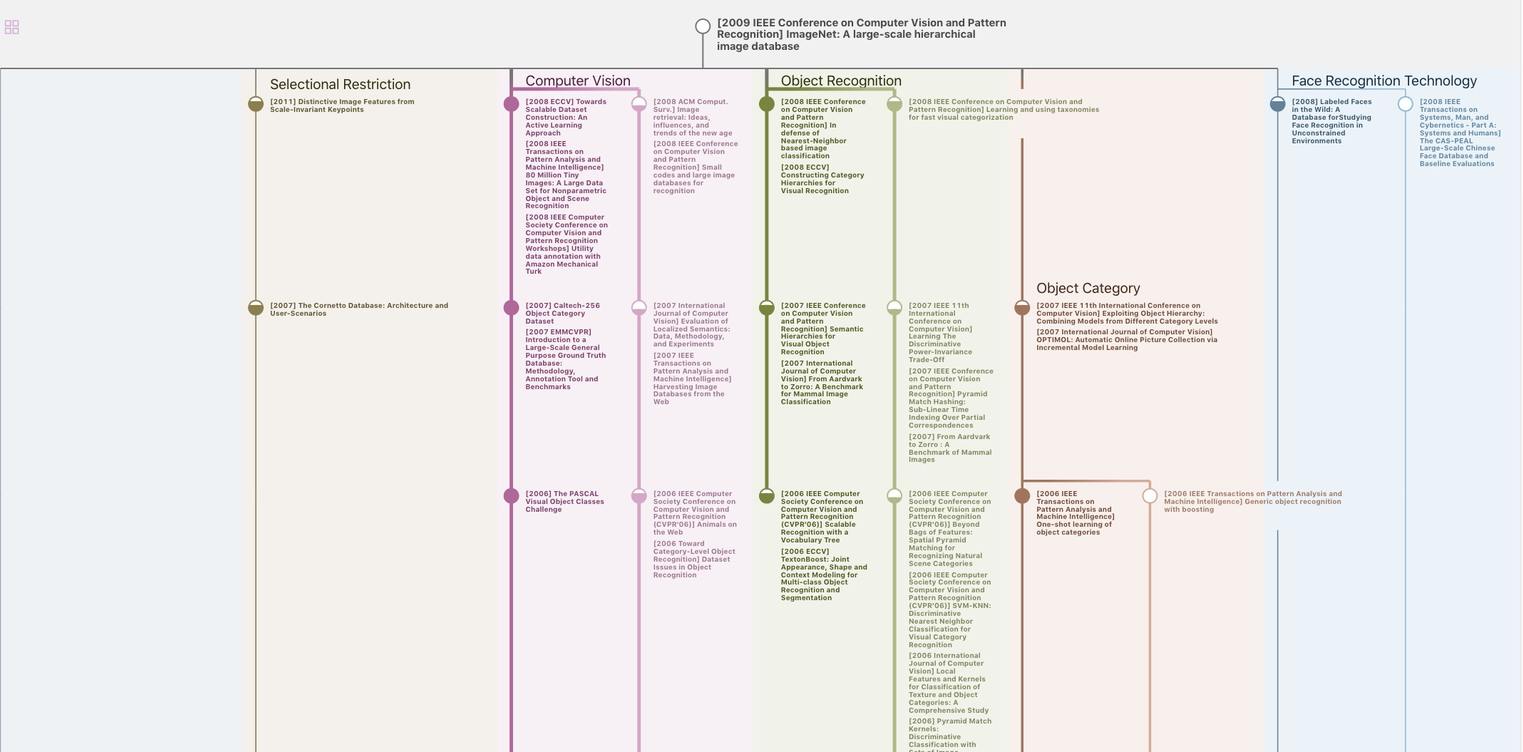
生成溯源树,研究论文发展脉络
Chat Paper
正在生成论文摘要