Abstract 2329: Cancer region definition using spatial gene expression patterns by super resolution reconstruction algorithm for spatial transcriptomics data
Cancer Research(2024)
摘要
Abstract Background: Spatially resolved transcriptomics (ST) has enabled a variety of cancer research on the heterogeneity of tumor microenvironment. However, when identifying cancer boundaries based on ST, the limited resolution of ST such as Visium hinders the accurate identification of cancerous regions. To address these issues, we have developed a novel approach for accurately classifying regions of the tumor by using deep image prior (DIP) algorithm to convert Visium data into high-resolution images. Methods: We proposed SuperST, which utilizes deep image prior to transform low-resolution ST data into high-resolution images, providing more accurate spatial marker expression patterns. This method was used to define cancer regions by combining with a pre-trained deep learning algorithm that recognizes spatial patterns. ST data of hepatocellular carcinoma (HCC) patients were used to apply SuperST. Firstly, 400 spatially variable genes were identified. SuperST leverages the deep image prior (DIP) algorithm and a U-Net neural network to transform ST data into high-resolution ones without needing ground-truth high-resolution data. The final high-resolution images of gene expression data were used to extract 512-dimensional feature vectors for each gene via a pre-trained convolutional neural network model (VGG16). Subsequently, we apply K-means clustering to these features to identify transcriptionally distinct tissue regions considering spatial information and compared with the human-labeled cancer regions. Results: The cancer region detection using spatial gene expression data and our algorithm yielded results that substantially align with the human annotations on the H&E images. Furthermore, compared to the cancer definition obtained using the CopyKat and SPACET algorithms, our results were more spatially consistent for cancerous regions as well as providing high-resolution image-level definition of cancer region margin. This indicates that our algorithm better utilizes spatial information and more effectively overcomes the low resolution of ST data. Conclusion: This research demonstrates the effective application of our SuperST algorithm to the challenging problem of cancer region detection only using spatial gene expression data. It suggests that our algorithm could be utilized for a variety of problems in spatial biology in cancer research. Citation Format: Jeongbin Park, Seungho Cook, Dongjoo Lee, Jinyeong Choi, Seongjin Yoo, Hyung-Jun Im, Daeseung Lee, Hongyoon Choi. Cancer region definition using spatial gene expression patterns by super resolution reconstruction algorithm for spatial transcriptomics data [abstract]. In: Proceedings of the American Association for Cancer Research Annual Meeting 2024; Part 1 (Regular Abstracts); 2024 Apr 5-10; San Diego, CA. Philadelphia (PA): AACR; Cancer Res 2024;84(6_Suppl):Abstract nr 2329.
更多查看译文
AI 理解论文
溯源树
样例
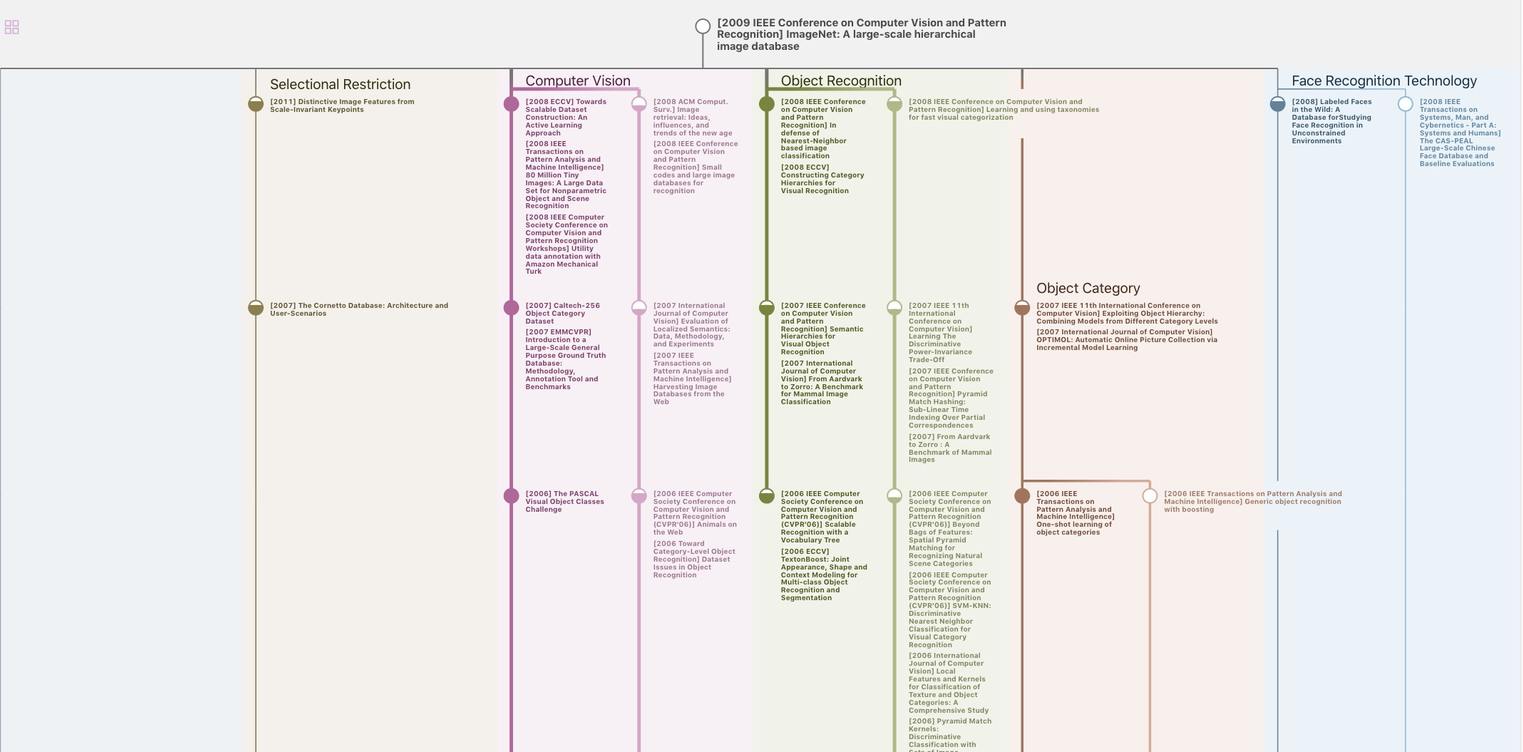
生成溯源树,研究论文发展脉络
Chat Paper
正在生成论文摘要