A Framework to Construct Financial Causality Knowledge Graph from Text.
IEEE International Conference on Semantic Computing(2024)
摘要
Causality analysis holds a prominent role in finance, and the presentation of causality could offer valuable insights for risk mitigation, investment decisions, and portfolio optimization. Recent research has extensively investigated the identification of causality from text, yet there is still a significant deficiency in providing a comprehensive causality presentation from those textual discoveries. In this paper, we present an end-to-end framework to automatically construct Financial Causality Knowledge Graph (FinCaKG) from text, which allows us to visualize the captured causality from a holistic perspective. This framework involves three distinct tasks, including causality sentence detection, cause-effect span identification, and causal dependency representation. To examine the adaptability of FinCaKG framework, we generate, compare, and analyze the distinct FinCaKGs created from different corpus. The results show that this framework has the capability to not only capture the confidential causality but also represent them in a highly detailed manner in the resulting knowledge graph - FinCaKG. We perform a comparative study with ConceptNet, revealing the notable contributions of FinCaKGs in terms of domain coverage and the density of its causal knowledge.
更多查看译文
关键词
Knowledge representation,Causality,Finance
AI 理解论文
溯源树
样例
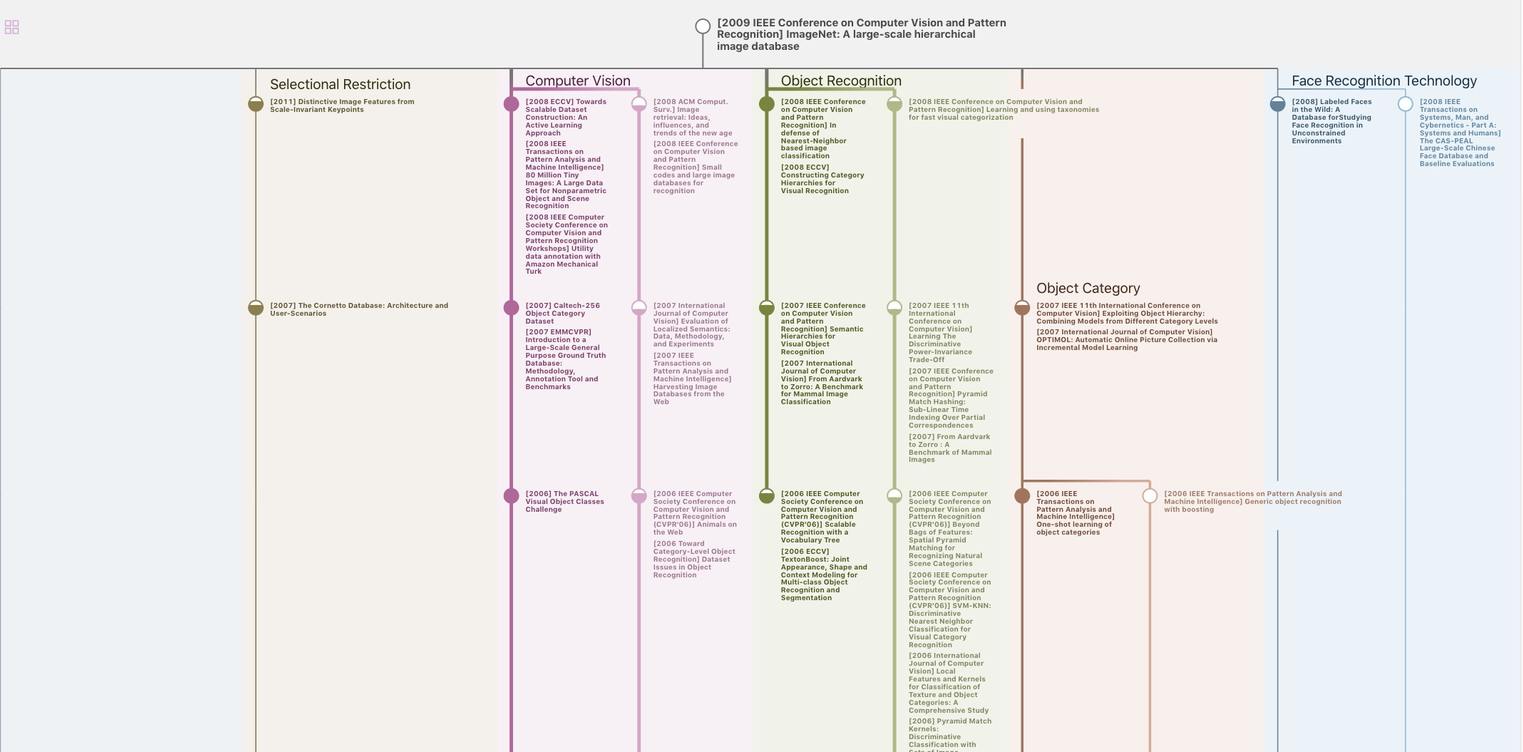
生成溯源树,研究论文发展脉络
Chat Paper
正在生成论文摘要