Abstract 3560: Quantified kinematics and wearable sensors to predict serious adverse events in patients undergoing cancer therapy trials
Cancer Research(2024)
摘要
Abstract Background: Serious adverse events (SAEs) are a critical determinant of drug toxicity in clinical trials. However the drivers of toxicity may be related to patient factors as much or more than drug related factors. Prior studies have shown that time in non-sedentary activity predicted toxicity in cancer patients receiving highly emetogenic chemotherapy. SAEs are a critical determinant of drug toxicity in clinical trials. However, predicting SAEs using conventional PS scales measurements, such as ECOG is challenging because measurements can vary among observers. Prior studies have used activity trackers to measure physical activity and showed that time spent in non-sedentary activity predicted toxicity in cancer patients receiving highly emetogenic chemotherapy. Here, we sought to extend these findings to early phase clinical trial participants by examining data collected in two single-arm, observational, prospective studies at USC Keck and MD Anderson centers. We explored the use of out-of-clinic wearable sensors as an objective metric of frailty so as to identify patients at risk for therapy complications. Methods: Adult patients diagnosed with cancer and enrolled in early stage clinical trials across USC Keck and MD Anderson centers were included in parallel single-arm, observational, prospective studies. Patients used a consumer grade wearable activity tracker (Fitbit) and the study examined associations between the number of severe adverse events to activity levels measured by metabolic equivalents (METs) and get-up-go times across the first 30 days. Trends were assessed using t-tests. Results: 36 eligible patients were included in METs activity analysis. There was a total of 16 recorded incidences of SAEs within the first 30 study days, with 10 patients experiencing at least one SAE. The median non-sedentary time for patients with no SAEs was 27.5 hours above 1.5 METs per week, compared to 21.5 hours for those with at least one SAE occurrence (p-value=0.0146, r2=0.1629). The median get-up-go times for those without SAEs was 10.0 seconds (n=26) and with SAEs was 9.8 seconds (n=10), (p-value=0.828, r2=0.0014). Conclusions: Non-sedentary time, as an objective measure of activity, can be used to identify those patients who are at greatest risk for SAEs in clinical trials. Widely available and inexpensive consumer grade sensors can be used as a stratification tool. Findings should be confirmed in larger patient cohorts across a wider variety of trial settings. Figure 1: Weekly non-sedentary hours vs. presence of SAE in first 30 days of trial enrollment Citation Format: Eli Tran, Luciano Nocera, Anand Kolatkar, Anthony El-Khoueiry, Jacob Thomas, Peter Kuhn, Andrew Zeng, Megumi Kai, Meghan Karuturi, Jorge Nieva. Quantified kinematics and wearable sensors to predict serious adverse events in patients undergoing cancer therapy trials [abstract]. In: Proceedings of the American Association for Cancer Research Annual Meeting 2024; Part 1 (Regular Abstracts); 2024 Apr 5-10; San Diego, CA. Philadelphia (PA): AACR; Cancer Res 2024;84(6_Suppl):Abstract nr 3560.
更多查看译文
AI 理解论文
溯源树
样例
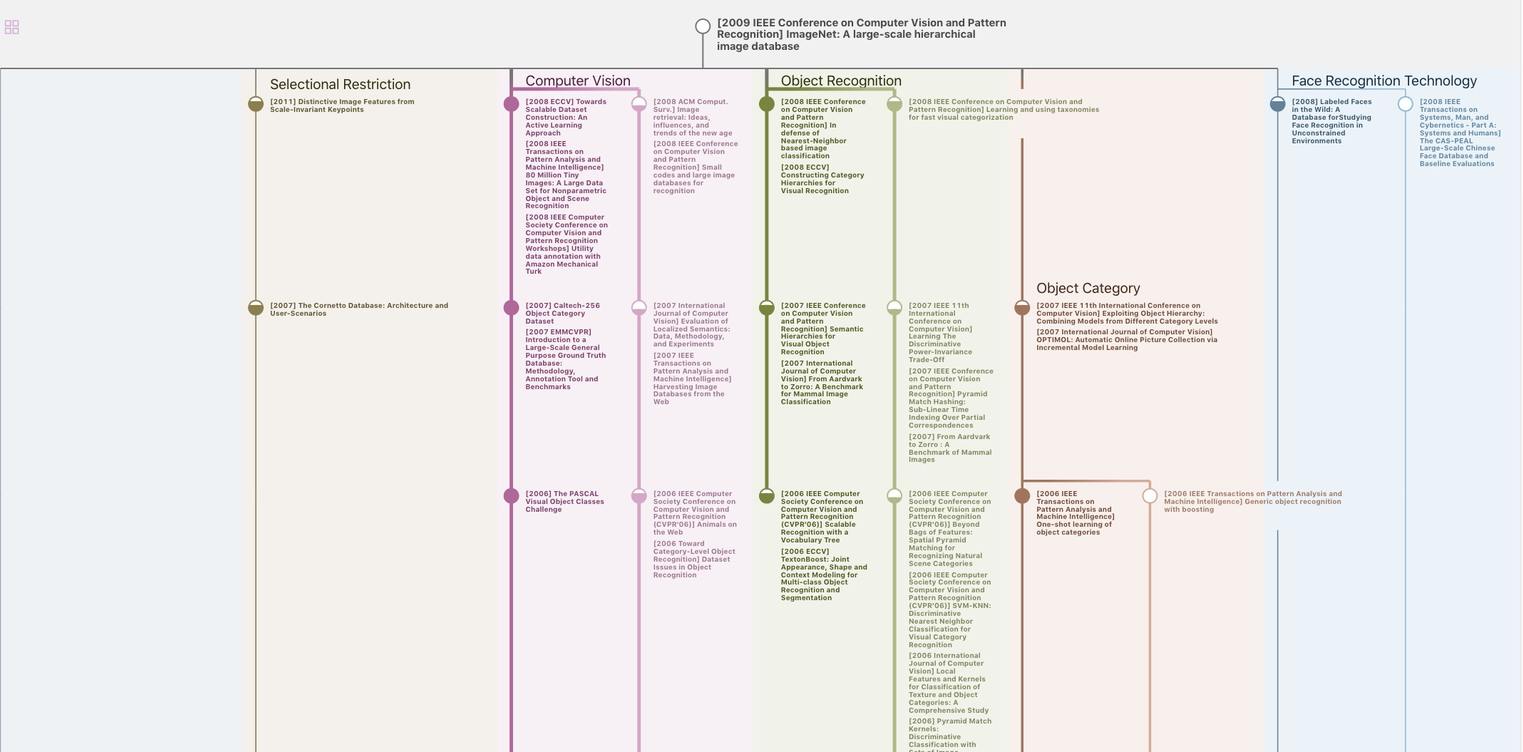
生成溯源树,研究论文发展脉络
Chat Paper
正在生成论文摘要