Abstract 907: ScreenDL: A transfer learning framework integrating tumor omics and functional drug screening for personalized clinical drug response prediction
Cancer Research(2024)
摘要
Abstract Precision oncology hinges on accurate prediction of patient-specific treatment response from tumor molecular inputs. While deep learning (DL) models achieve state-of-the-art response prediction in cell lines, existing methods do not readily translate to the clinic where training data is limited. Here, we have developed and validated ScreenDL, a novel DL framework designed explicitly for use in clinical precision oncology applications. The underlying architecture of ScreenDL consists of two fully connected branches dedicated to learning rich embeddings of a drug’s chemical structure and a tumor’s transcriptomic profile respectively. These tumor omic and drug molecular embeddings are fed into a shared response prediction subnetwork which outputs predicted ln(IC50) values. The ScreenDL training schema incorporates three phases, each designed to improve clinical response prediction: (1) an initial pretraining phase leverages large-scale cell line pharmacogenomic datasets to extract patterns/relationships linking tumor omics and drug response; (2) subsequent transfer learning integrates pharmacogenomic data from patient-derived models of cancer, adapting the pretrained model to a more patient-relevant context; (3) patient-level omics and drug screening data (e.g., from matched patient-derived organoid (PDO) models) is integrated through patient-specific fine-tuning, generating a patient-specific response prediction model. Importantly, biomaterial from surgical tumor resection is often available for organoid establishment and patient-specific drug screening in practical clinical scenarios. To assess the utility of our ScreenDL framework for clinical response prediction, we applied ScreenDL to predict treatment response in 50 advanced/metastatic triple negative breast cancer (TNBC) patient-derived xenograft models (PDX). We leveraged tumor omics profiles for each PDX and drug screening data from matched PDX-derived organoids (PDxOs), mirroring the combination of patient-level tumor omic characterization and functional drug screening in matched PDOs, a protocol currently being piloted in Functional Precision Oncology trials at the University of Utah Huntsman Cancer Institute. After cell line pretraining, ScreenDL achieves a modest median Pearson correlation per drug of 0.15 relative to 0.03 for the leading compared model. However, transfer learning and subsequent PDX-specific fine-tuning significantly improve performance, producing median Pearson correlations per drug of 0.39 and 0.51, respectively. These results demonstrate the ability of our ScreenDL framework to dramatically improve response prediction in clinically relevant domains, bringing DL-based precision treatment selection closer to clinical application. Citation Format: Casey Sederman, Tonya Di Sera, Yi Qiao, Xiaomeng Huang, Bryan E. Welm, Alana L. Welm, Gabor Marth. ScreenDL: A transfer learning framework integrating tumor omics and functional drug screening for personalized clinical drug response prediction [abstract]. In: Proceedings of the American Association for Cancer Research Annual Meeting 2024; Part 1 (Regular Abstracts); 2024 Apr 5-10; San Diego, CA. Philadelphia (PA): AACR; Cancer Res 2024;84(6_Suppl):Abstract nr 907.
更多查看译文
AI 理解论文
溯源树
样例
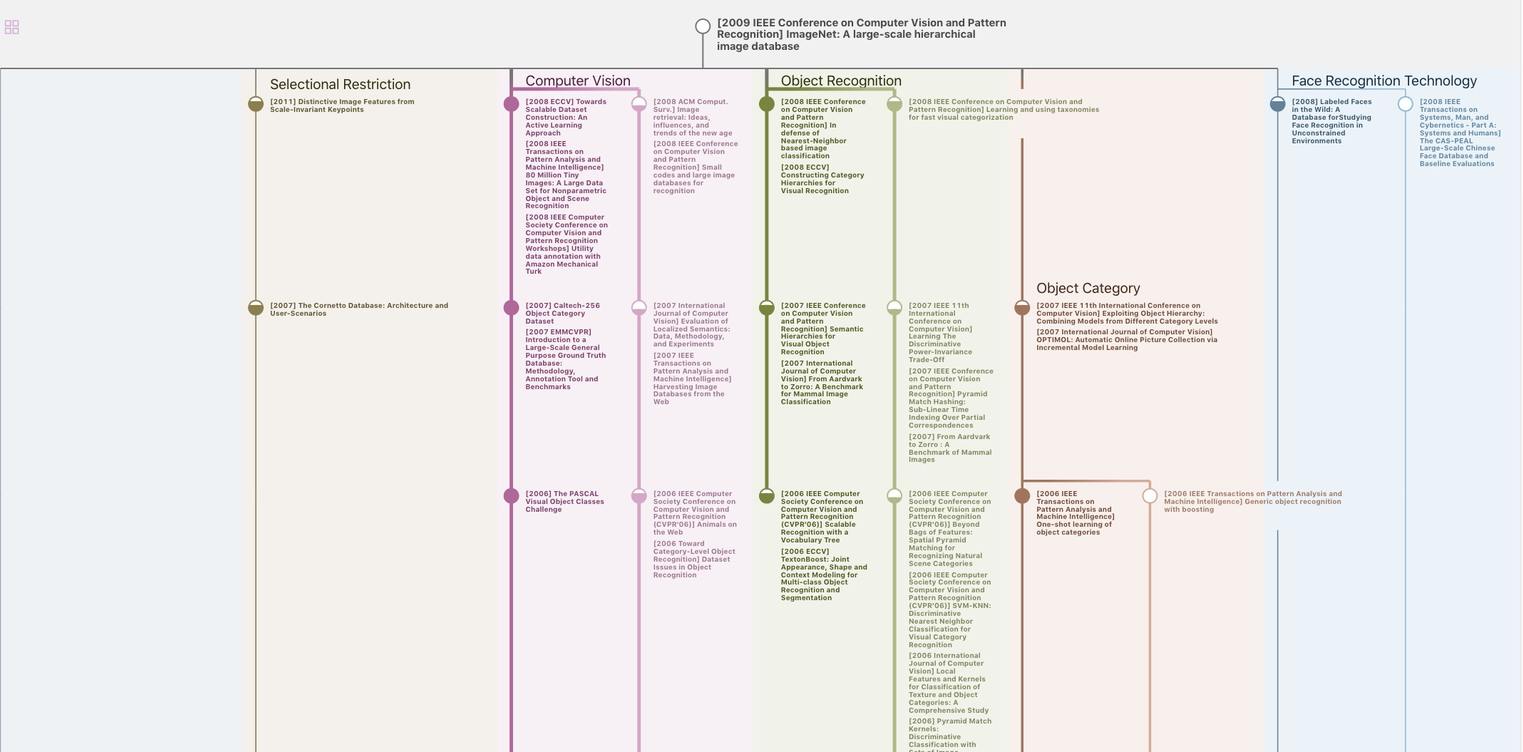
生成溯源树,研究论文发展脉络
Chat Paper
正在生成论文摘要