Abstract 7386: Multi-plane rectal tumor segmentation on pre-treatment MRI via human-in-the-loop deep learning
Cancer Research(2024)
摘要
Abstract Introduction: Precise segmentation of rectal tumors on pre-treatment MRI is critical for in vivo clinical staging and treatment selection. While automated deep learning models can help alleviate laborious manual annotation, these require significant expertise and time commitment. Towards addressing these issues, we present initial results of an integrated human-in-the-loop refinement learning model for semi-automated segmentation of rectal tumors across multiple acquisition planes (axial, coronal) on pre-treatment MRI scans. Methods: This retrospective study accrued baseline MRI scans from rectal cancer patients at four different institutions. A deep learning (DL) nnUNet tumor segmentation model was first trained on both axial plane and coronal plane T2w MRIs from two institutions, with segregated training and internal validation cohorts based on available radiologist annotations. The DL model outputs tumor delineations on both axial and coronal MRI scans in a separate human-in-the-loop (HITL) optimization cohort. Two rounds of HITL refinement learning were then conducted, where in each round model-generated segmentations were refined by two novice radiology readers. Refined annotations were then used to re-optimize the nnUnet model to yield final tumor segmentations. Model-generated segmentations were evaluated before HITL as well as after each round of HITL against “ground truth” radiologist annotations obtained for an external validation cohort, with performance quantified via dice similarity coefficient (DSC) as well as time taken for human annotation of MRIs. Statistical significance was determined via Wilcoxon rank-sum testing. Results: A total of 381 MRI scans from 231 rectal cancer patients were considered. After one round of HITL refinement, DL-based segmentations yielded the best overall performance on the external validation cohort (N=20, two institutions) for both axial (DSC = 0.76) and coronal scans (DSC=0.75). A second round of HITL refinement did not yield significantly different model performance. By comparison, the pre-HITL DL segmentation model yielded significantly worse performance on both axial (DSC=0.60, p=0.0362) and coronal (DSC=0.62, p=0.0186) MRI scans. HITL refinement for rectal tumor annotation was also found to significantly improve reader efficiency by requiring only 18 minutes/scan compared to manual “ground truth” de novo annotations which took up to 38 minutes/scan (p<0.001). Conclusion: Human-in-the-loop deep learning models can help generate accurate rectal tumor segmentations on pre-treatment MRI scans across both axial and coronal acquisition planes. Improvements to model generalizability, model efficacy, and reader efficiency suggest the potential for HITL-based semi-automated segmentation schemes for other medical imaging tasks. Citation Format: Michael Kong, Thomas DeSilvio, Leo Bao, Brennan Flannery, Benjamin N. Parker, Stephen Tang, Gregory O'Connor, Amit Gupta, Emily Steinhagen, Andrei S. Purysko, Eric L. Marderstein, Aaron Carroll, Marka Crittenden, Michael Gough, Kristina Young, Satish E. Viswanath. Multi-plane rectal tumor segmentation on pre-treatment MRI via human-in-the-loop deep learning [abstract]. In: Proceedings of the American Association for Cancer Research Annual Meeting 2024; Part 1 (Regular Abstracts); 2024 Apr 5-10; San Diego, CA. Philadelphia (PA): AACR; Cancer Res 2024;84(6_Suppl):Abstract nr 7386.
更多查看译文
AI 理解论文
溯源树
样例
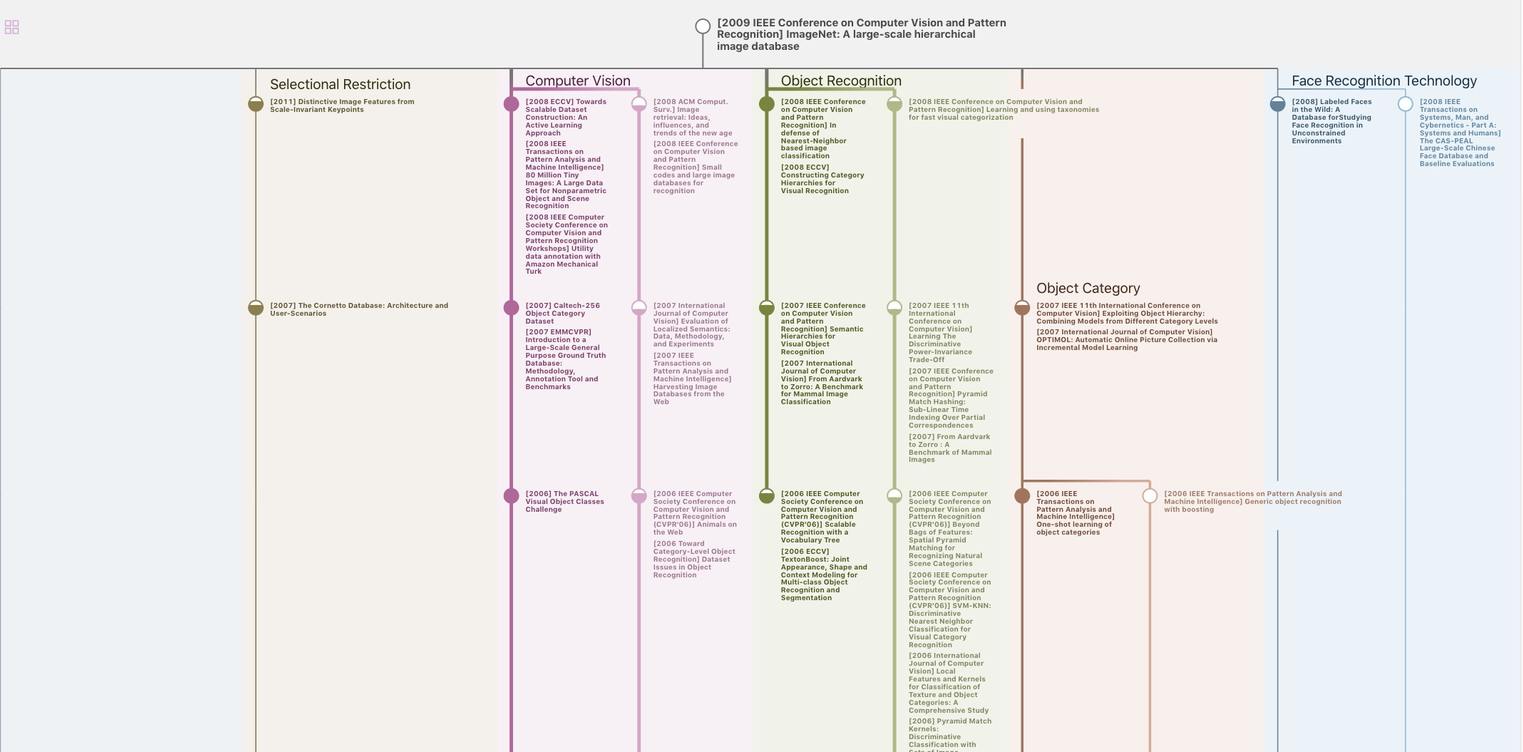
生成溯源树,研究论文发展脉络
Chat Paper
正在生成论文摘要