Modeling Student Performance using Feature Crosses Information for Knowledge Tracing
IEEE Transactions on Learning Technologies(2024)
摘要
Knowledge tracing (KT) is an intelligent educational technology used to model students’ learning progress and mastery in adaptive learning environments for personalized education. Despite utilizing deep learning models in KT, current approaches often oversimplify students’ exercise records into knowledge sequences, which fail to explore the rich information within individual questions. Additionally, existing KT models tend to neglect the complex, higher-order relationships between questions and latent concepts. Therefore, we introduce a novel model called Feature Crosses Information-based Knowledge Tracing (FCIKT) to explore the intricate interplay between questions, latent concepts, and question difficulties. FCIKT utilizes a fusion module to perform feature crosses operations on questions, integrating information from our constructed multi-relational heterogeneous graph using graph convolutional networks. We deployed a multi-head attention mechanism, which enriches the static embedding representations of questions and concepts with dynamic semantic information to simulate real-world scenarios of problem-solving. We also used gated recurrent units to dynamically capture and update the students’ knowledge state for final prediction. Extensive experiments demonstrated the validity and interpretability of our proposed model.
更多查看译文
关键词
Knowledge tracing,intelligent education,graph neural network,recurrent neural network
AI 理解论文
溯源树
样例
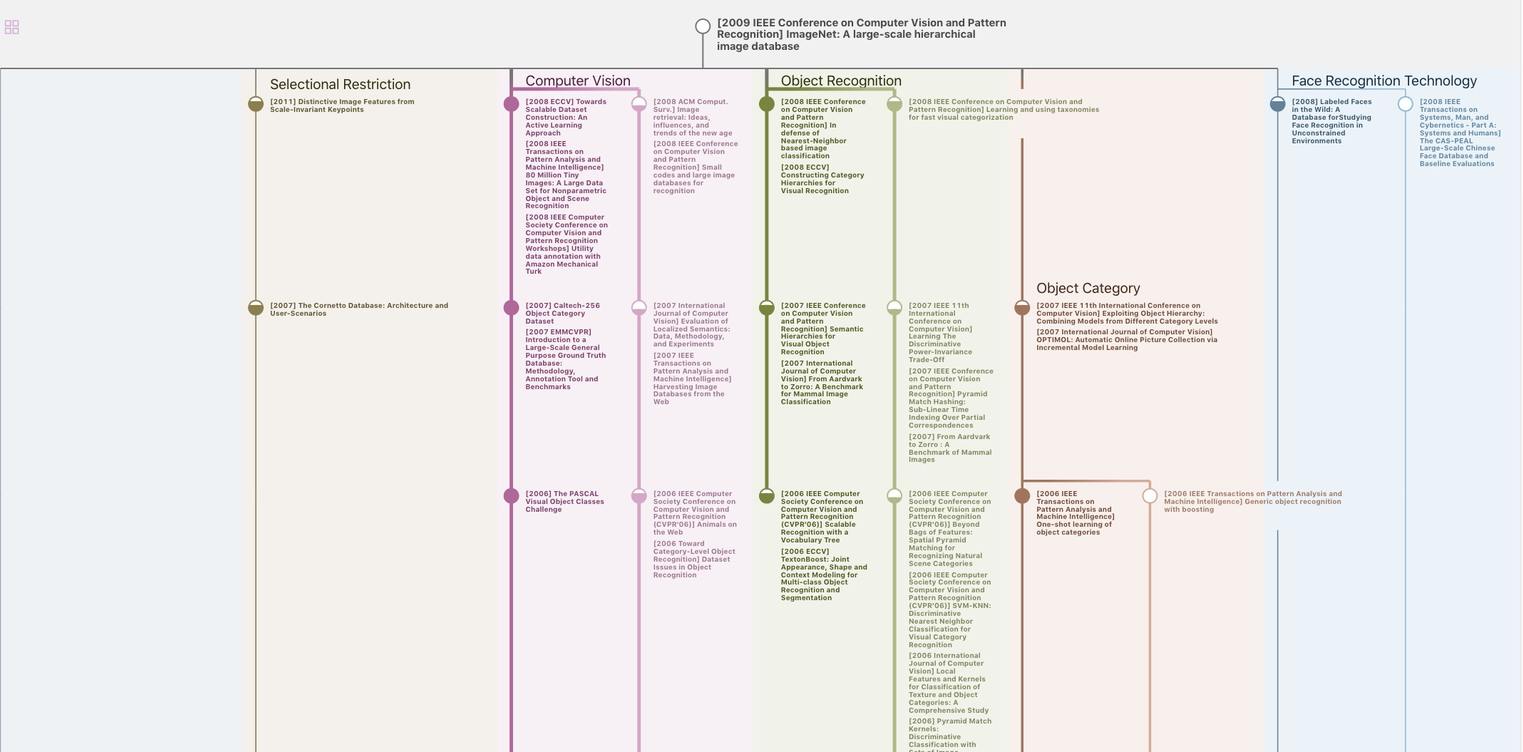
生成溯源树,研究论文发展脉络
Chat Paper
正在生成论文摘要