Advancing 6-DoF Instrument Pose Estimation in Variable X-Ray Imaging Geometries.
IEEE transactions on image processing : a publication of the IEEE Signal Processing Society(2024)
摘要
Accurate 6-DoF pose estimation of surgical instruments during minimally invasive surgeries can substantially improve treatment strategies and eventual surgical outcome. Existing deep learning methods have achieved accurate results, but they require custom approaches for each object and laborious setup and training environments often stretching to extensive simulations, whilst lacking real-time computation. We propose a general-purpose approach of data acquisition for 6-DoF pose estimation tasks in X-ray systems, a novel and general purpose YOLOv5-6D pose architecture for accurate and fast object pose estimation and a complete method for surgical screw pose estimation under acquisition geometry consideration from a monocular cone-beam X-ray image. The proposed YOLOv5-6D pose model achieves competitive results on public benchmarks whilst being considerably faster at 42 FPS on GPU. In addition, the method generalizes across varying X-ray acquisition geometry and semantic image complexity to enable accurate pose estimation over different domains. Finally, the proposed approach is tested for bone-screw pose estimation for computer-aided guidance during spine surgeries. The model achieves a 92.41% by the 0.1∙d ADD-S metric, demonstrating a promising approach for enhancing surgical precision and patient outcomes. The code for YOLOv5-6D is publicly available at https://github.com/cviviers/YOLOv5-6D-Pose.
更多查看译文
关键词
X-ray instrument detection,6-DoF pose estimation,surgical vision,imaging geometry,deep learning
AI 理解论文
溯源树
样例
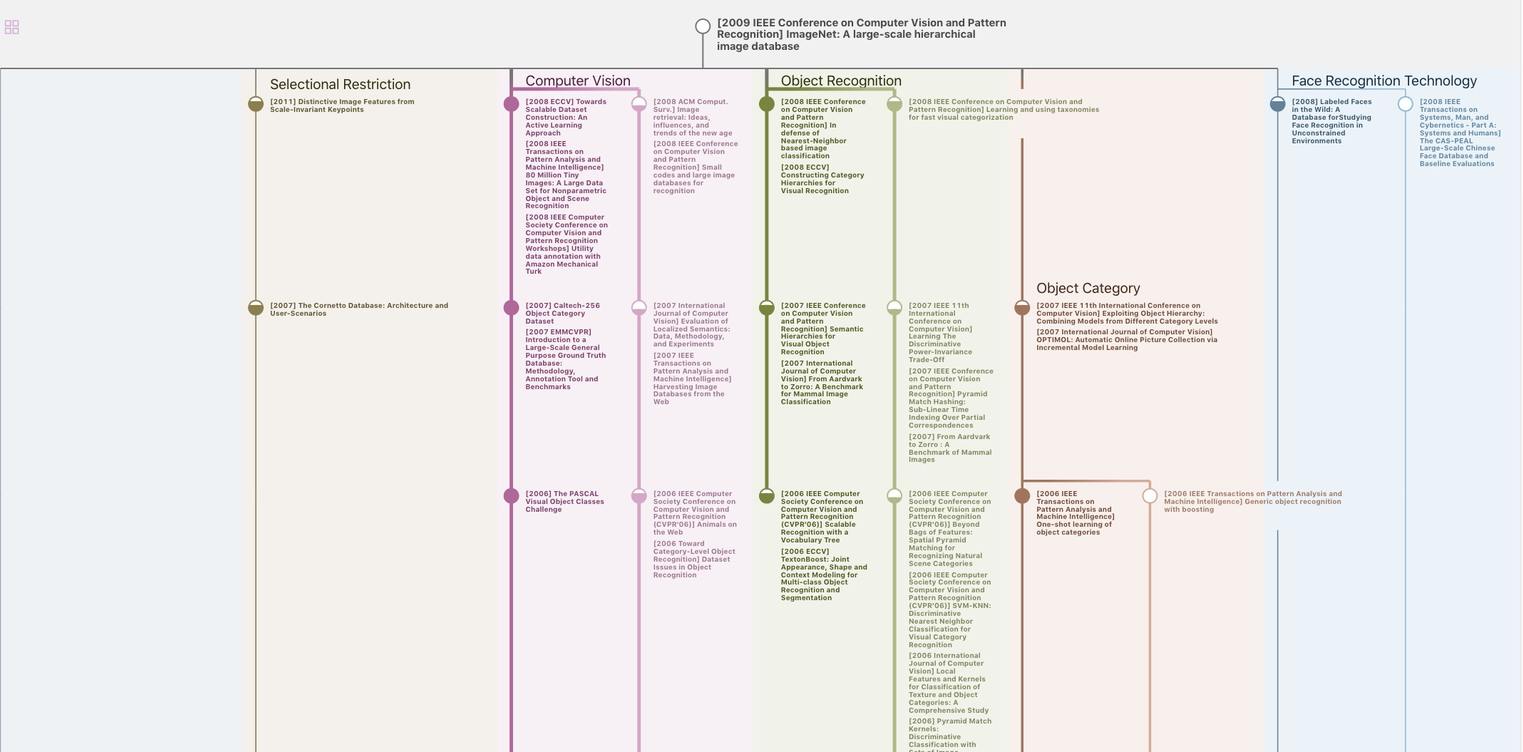
生成溯源树,研究论文发展脉络
Chat Paper
正在生成论文摘要