Abstract 1789: Machine learning-based method to analyze metabolic fluxes of patient tumors
Cancer Research(2024)
摘要
Abstract Personalized oncology must overcome the complex challenge of distinct cancer phenotypes in each patient, formed in part in response to unique metabolic crosstalk among cells in the tumor microenvironment which can be a determining factor in therapeutic response. Although isotope tracing sheds light on differences of metabolite enrichments in tumor and normal tissues, quantifying the metabolic fluxes in patients remains elusive. The conventional metabolic flux analysis tools are unable to estimate patient metabolic fluxes because we are limited to a single time point enrichment datapoint from each patient’s in-vivo measurement at isotopic non-steady state. We overcame this challenge by generating training data from the limited patient data and implementing a machine learning model to predict fluxes. To generate training data, we first simulated constrained random fluxes and enrichment patterns at different time points using patient circulating metabolites enrichment via an isotopic non-stationary metabolic flux analysis framework. Using this data, we trained a convolutional neural network to predict flux ratios. To validate our model, we compared predicted flux ratios to the experimental data from mouse models for two different treatments. Our recent findings from a glioblastoma (GBM) mouse model study using conventional metabolic flux analysis revealed higher de novo guanosine monophosphate (GMP) synthesis in GBM than normal cortex. These tumors can be sensitive to mycophenolate mofetil (MMF) which inhibits inosine monophosphate dehydrogenase, but only if the major source of GMP production is de novo synthesis and not salvage. Our machine learning model can discriminate between sources of GMP synthesis in patients, thus enabling prediction of potential responders. Furthermore, we previously showed that serine uptake is higher in GBM than normal cortex, and a serine- and glycine-restricted diet slows tumor growth in mice. While both circulating serine and microenvironment-derived serine could account for serine uptake in GBM, we hypothesized that the circulating serine dependence would better predict response to dietary serine depletion. Hence, we combined patient scRNA-seq and metabolomics data to feed our neural network framework to estimate microenvironmental and circulating serine uptake in six patients with brain cancer. Thus, we have shown that our model can identify patients who will benefit from MMF treatment or a serine- and glycine-restricted diet, enabling administration of highly effective personalized treatments for GBM patients. Citation Format: Baharan Meghdadi, Anjali Mittal, Andrew J. Scott, Sravya Palavalasa, Daniel R. Wahl, Deepak Nagrath. Machine learning-based method to analyze metabolic fluxes of patient tumors [abstract]. In: Proceedings of the American Association for Cancer Research Annual Meeting 2024; Part 1 (Regular Abstracts); 2024 Apr 5-10; San Diego, CA. Philadelphia (PA): AACR; Cancer Res 2024;84(6_Suppl):Abstract nr 1789.
更多查看译文
AI 理解论文
溯源树
样例
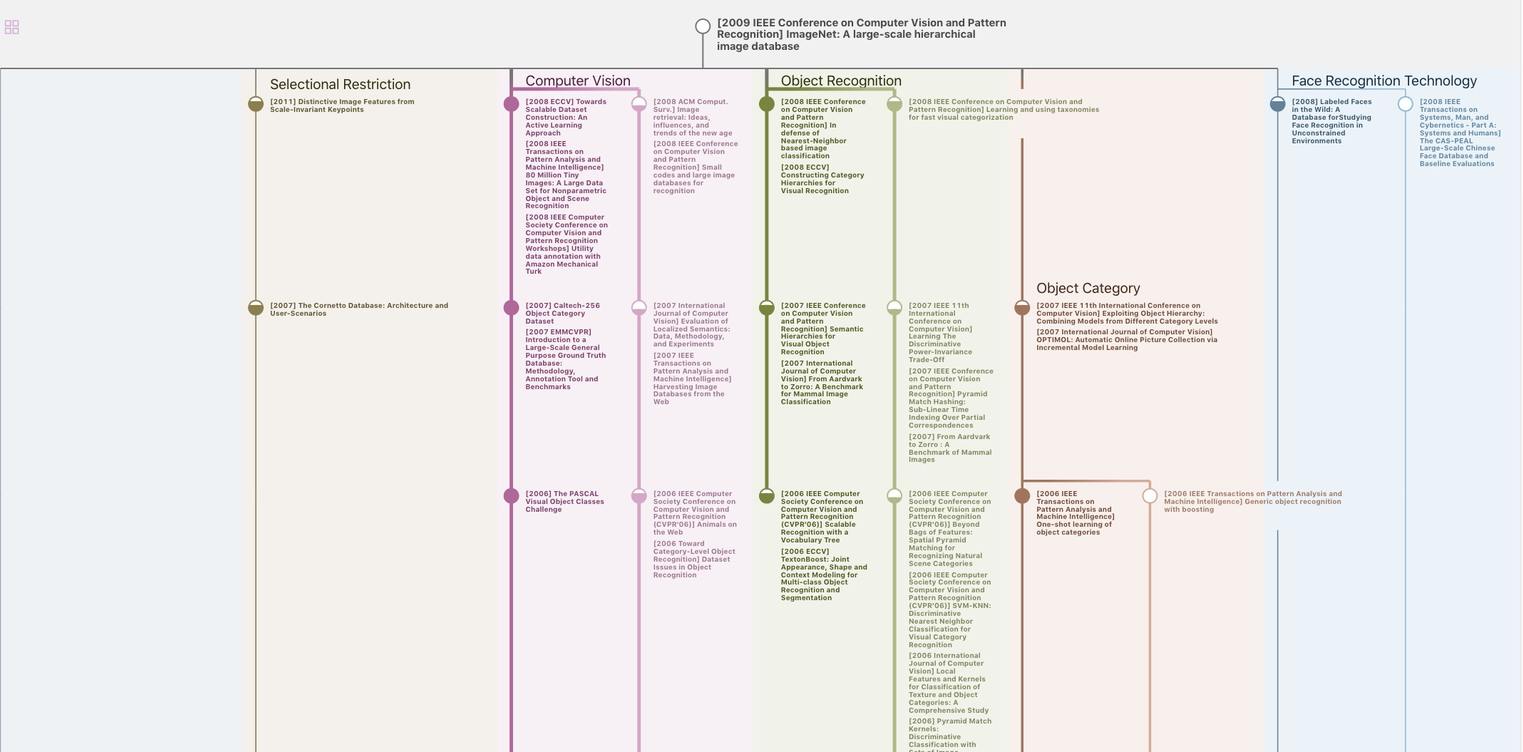
生成溯源树,研究论文发展脉络
Chat Paper
正在生成论文摘要