Abstract 7440: Symptom network analysis and unsupervised clustering of a cohort of oncology patients identifies drivers of symptom burden and patient subgroups with distinct symptom patterns
Cancer Research(2024)
摘要
Abstract Purpose: Inter-individual variability in oncology patients’ symptom experiences poses significant challenges in prioritizing symptoms for targeted intervention(s). In this study, computational approaches were used to unbiasedly characterize the heterogeneity of the symptom experience of oncology patients to elucidate their symptom patterns and drivers of symptom burden. Methods: Severity ratings for 32 symptoms on the Memorial Symptom Assessment Scale from 3088 oncology patients were analyzed. Gaussian Graphical Model symptom networks were constructed for the entire cohort and subgroups identified through unsupervised clustering of symptom co-severity patterns. Symptom network characteristics were analyzed and compared using permutation-based statistical tests. Demographic and clinical characteristic differences between subgroups were assessed using multinomial logistic regression. Results: Network analysis of the entire cohort revealed three core symptom clusters: constitutional, gastrointestinal-epithelial, and psychological. Lack of energy was identified as central to the network, suggesting that it plays a crucial role in driving overall symptom burden and may be the most salient to target clinically. Unsupervised clustering of patients based on shared symptom co-severity patterns identified six patient subgroups with distinct symptom patterns and demographic and clinical characteristics. The first subgroup represented a baseline cohort of patients who are most affected by common constitutional symptoms of cancer care. The second subgroup represented patients with severe overall symptom burden. The third subgroup represented patients primarily experiencing neuropathy. The fourth subgroup represented patients with advanced disease and treatment-related symptoms but relatively high quality of life. The fifth subgroup represented younger patients with pronounced psychological disease burden. The sixth subgroup represented patients with minimal symptom burden. Age, treatment status, and Karnofsky Performance Score were the strongest determinants of subgroup membership, but their interplay (in one case even with ethnicity) reaffirms the complex multi-factorial nature of the symptom experience. For each subgroup, symptoms with the highest network centralities that should be prioritized for management were identified. Conclusions: This study provides a translational research framework for clinicians to better understand and address the symptom care needs of oncology patients. The framework combines unbiased stratification of patients and in-depth modeling of symptom relationships to capture the heterogeneity in their experiences. When validated, the identified core symptoms and associated clinical determinants may inform precision-based symptom management. Citation Format: Brandon H. Bergsneider, Terri S. Armstrong, Yvette P. Conley, Bruce Cooper, Marilyn Hammer, Jon D. Levine, Steven Paul, Christine Miaskowski, Orieta Celiku. Symptom network analysis and unsupervised clustering of a cohort of oncology patients identifies drivers of symptom burden and patient subgroups with distinct symptom patterns [abstract]. In: Proceedings of the American Association for Cancer Research Annual Meeting 2024; Part 1 (Regular Abstracts); 2024 Apr 5-10; San Diego, CA. Philadelphia (PA): AACR; Cancer Res 2024;84(6_Suppl):Abstract nr 7440.
更多查看译文
AI 理解论文
溯源树
样例
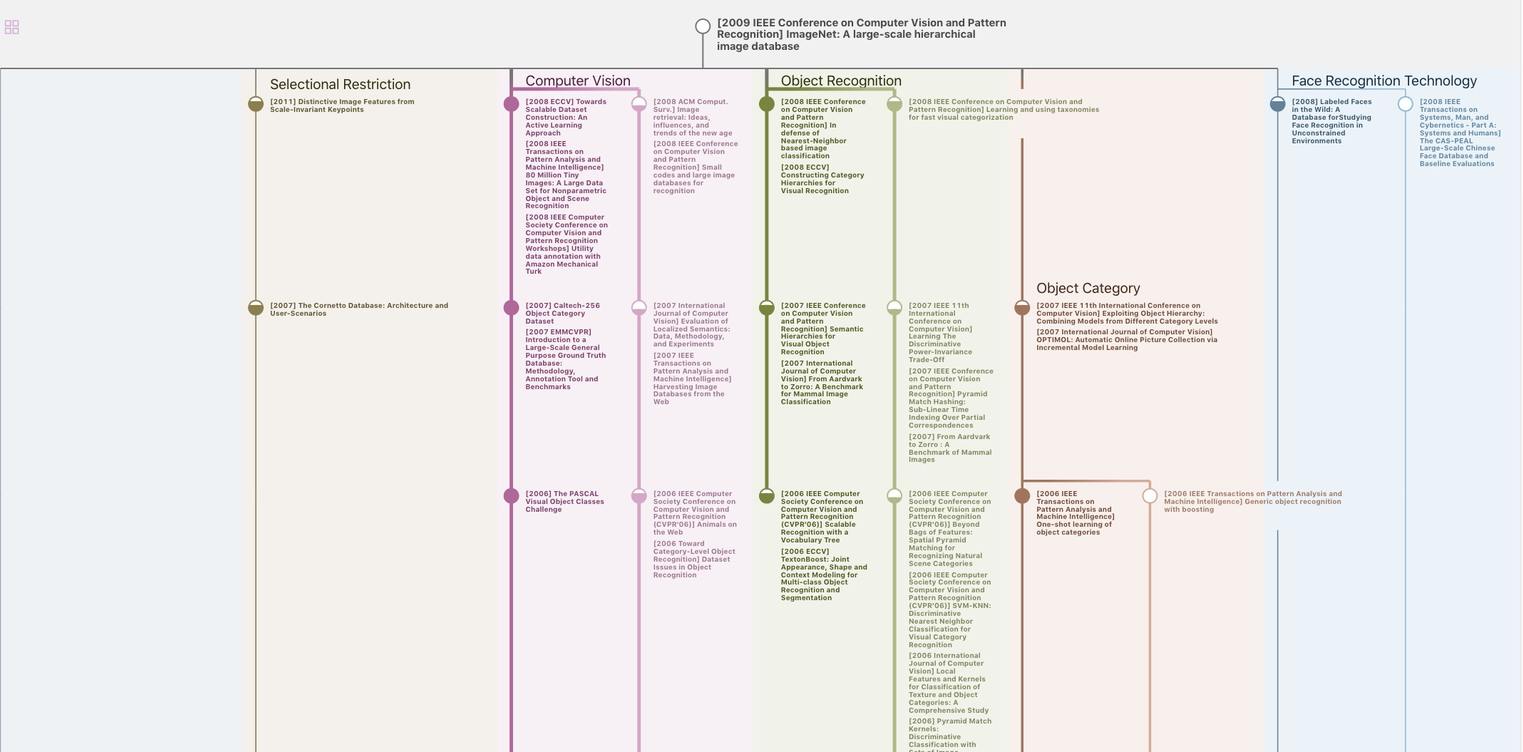
生成溯源树,研究论文发展脉络
Chat Paper
正在生成论文摘要