Malicious-Resistant Non-Interactive Verifiable Aggregation for Federated Learning
IEEE Transactions on Dependable and Secure Computing(2024)
摘要
In cross-device federated learning, verifiable secure aggregation enables clients to aggregate their locally trained model parameters through a malicious server to obtain a global model. To prevent the malicious server from tampering with the results, solutions have been proposed to ensure verifiability during this aggregation process. However, previous solutions either failed to achieve non-interactivity, which is critical in the cross-device setting, or failed to achieve malicious server resistance due to their underlying approach. Thus in this paper, we propose a lightweight non-interactive multi-client verifiable computation scheme (lwMVC) and then construct the malicious-resistant non-interactive verifiable aggregation (MRNIVA) for cross-device federated learning by the newly introduced underlying approach lwMVC. By combining Half Gates and non-iterative proxy oblivious-transfer, lwMVC meets both the non-interactivity and malicious resistance properties. Then, after solving the user dropout and efficiency problem, we construct the MRNIVA by lwMVC. Since we focus on the cross-device scenario, we conduct experiments on a Single Board Computer. The experiment result demonstrates that MRNIVA remains efficient compared to previous works after achieving both non-interactive and malicious resistance properties. As far as we know, we are the first to carry out verifiable aggregation experiments using such resource-constrained devices.
更多查看译文
关键词
Federated learning,garbling,secure aggregation,verifiable computation
AI 理解论文
溯源树
样例
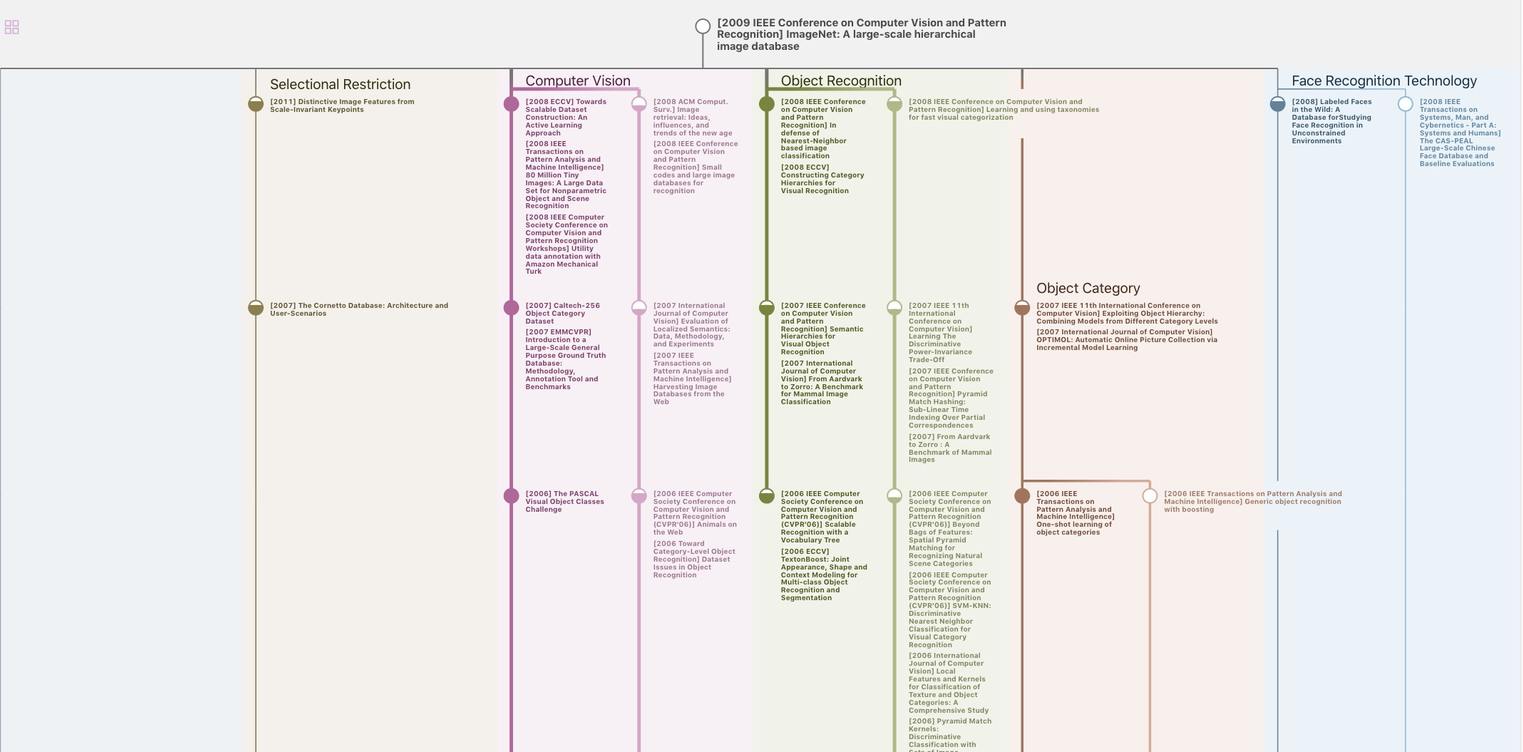
生成溯源树,研究论文发展脉络
Chat Paper
正在生成论文摘要