Abstract 3537: Assessing machine learning models of drug response predictions using orthotopic patient-derived xenograft
Cancer Research(2024)
摘要
Abstract Accurate prediction of drug response using machine learning (ML) remains a significant challenge in drug development as well as in personalized cancer therapy. Furthermore, there is a scarcity of rigorous external validation studies for evaluating drug response prediction models. Even when available, such validations are often confined to in-vitro studies. Orthotopic patient-derived xenograft (O-PDX) mice serve as essential preclinical models that closely replicate the human tumor microenvironment and assess the therapeutic response to cancer treatments. We present CertisAITM, a novel ensemble of machine learning models that have been trained on a vast array of experimental high-throughput screenings of both monotherapies and combination therapies, incorporating over 4500 investigational and FDA-approved drugs across 10 major cancer indications. The results of our ML drug response prediction models show an average R2 of 0.75 and RMSE of 0.62 (across internal 5-fold cross-validation) for all 10 cancer prediction models. Our evaluation of CertisAI's drug response predictions within O-PDX pharmacology studies has shown a notable overall correlation between actual observed tumor growth inhibition (TGI) and predicted TGI, with an r value of 0.45 across seven indications. The most accurate prediction model achieved an r value of 0.7 for colorectal cancer, encompassing 37 treatments in 6 O-PDX studies. Altogether, these results highlight CertisAI’s potential in enhancing pre-clinical model selection as well as personalized treatment strategies in oncology. Citation Format: Yuan-Hung Chien, Raffaella Pippa, Warren Andrews, Javier Rodriguez, Kristen Buck, Derrick Gorospe, Elizabeth Valencia, Bianca Carapia, Emily Eastwood, Jantzen Sperry, Jonathan Nakashima, Long Do. Assessing machine learning models of drug response predictions using orthotopic patient-derived xenograft [abstract]. In: Proceedings of the American Association for Cancer Research Annual Meeting 2024; Part 1 (Regular Abstracts); 2024 Apr 5-10; San Diego, CA. Philadelphia (PA): AACR; Cancer Res 2024;84(6_Suppl):Abstract nr 3537.
更多查看译文
AI 理解论文
溯源树
样例
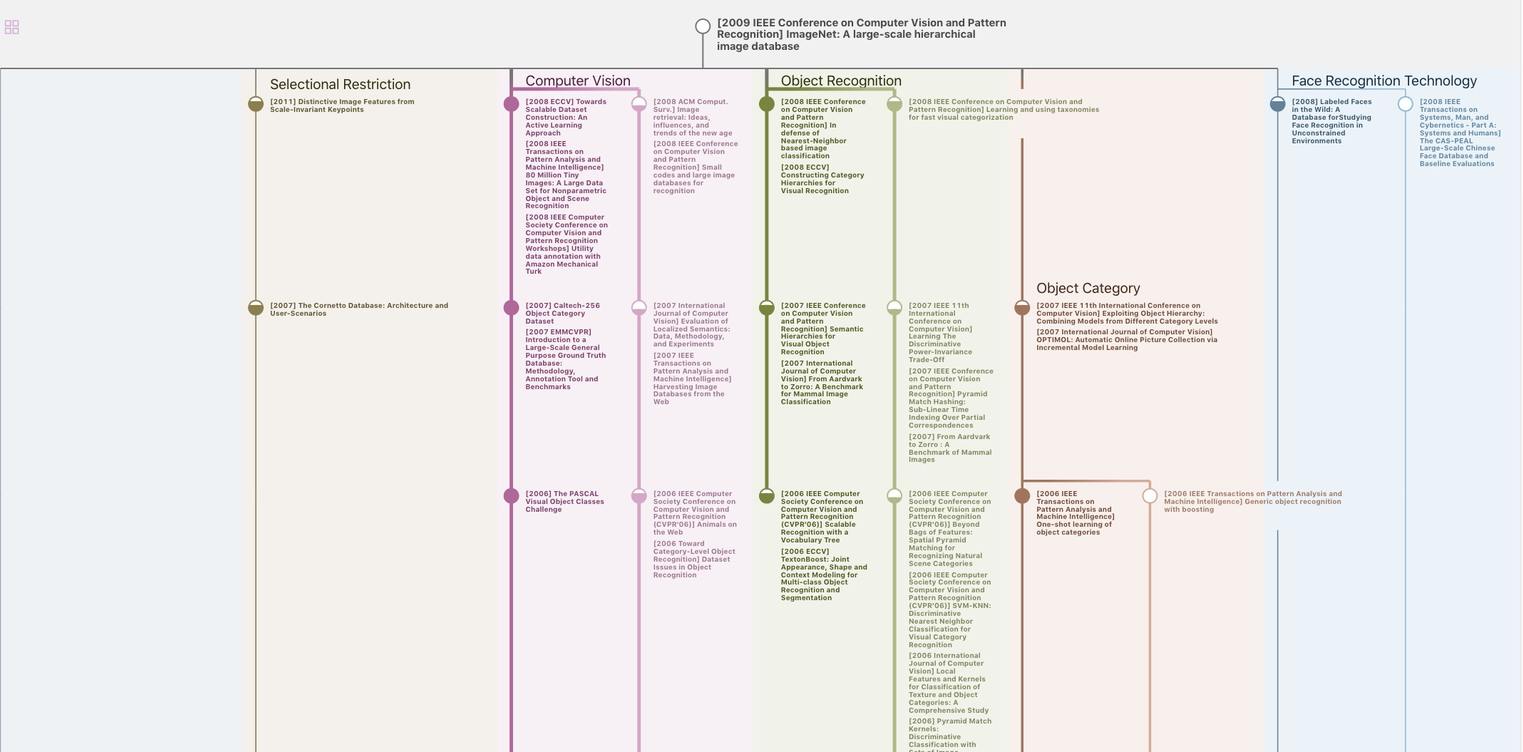
生成溯源树,研究论文发展脉络
Chat Paper
正在生成论文摘要