Abstract 7410: PyVIPER: A fast and scalable toolkit for the identification of dysregulated proteins in tumor-derived single-cell RNA-seq data
Cancer Research(2024)
摘要
Abstract Single-cell RNA sequencing (scRNA-seq) enabled the characterization of tumor microenvironment heterogeneity with unprecedented resolution. However, its low signal-to-noise ratio due to data sparsity hinders biomarkers quantitative measurements. To perform a more robust assessments of protein markers, we have previously introduced the use of the VIPER algorithm that estimates regulatory proteins activity using the expression of their downstream targets by leveraging lineage specific regulatory networks. We have shown that this approach compares favorably with both gene expression and protein abundance measurements to identify novel cell states corresponding to tumor subpopulations that are undetectable at the gene expression level, by inferring the mechanistic contribution of regulatory and signaling proteins to cellular phenotypes. VIPER-inferred cell states have been linked to critical functional roles, including immune-evasion and progression, disease heterogeneity and drug sensitivity in cancer. However, the ever-growing size of scRNA-seq datasets demands for scalable tools that simultaneously analyze tens or hundreds of thousands of cells in tumor contexts, such as tumor atlases and large clinical cohorts. We present PyVIPER, a fast, memory-efficient, and highly scalable Python framework that allows protein activity inference of large scRNA-seq datasets. PyVIPER is fully integrated with both AnnData and Scanpy frameworks for single-cell analysis and enables a seamless interface with cutting-edge machine learning libraries (e.g., sckit-learn and TensorFlow). PyVIPER provides multiple algorithms for enrichment analysis, novel modules for postprocessing and pathway analysis, and a set of procedures tailored for precision oncology studies in single cells, including OncoMatch, a validated platform to assess the fidelity of models (in vitro or animal) with human tumors for drug sensitivity elucidation. We benchmarked PyVIPER against several scRNA-seq cancer datasets obtaining runtimes reduction of several orders of magnitude (from hours to minutes) when compared to previous VIPER implementations. Taken together, PyVIPER scalability and its embedded toolkit for cancer data analysis address the need to efficiently extend protein activity analyses to large single-cell datasets, with the goal of providing cancer researchers with an effective platform to identify aberrant molecular mechanisms in cancer cells. Citation Format: Luca Zanella, Alexander L. Wang, Zizhao J. Lin, Lukas J. Vlahos, Miquel Anglada Girotto, Aziz Zafar, Andrea Califano, Alessandro Vasciaveo. PyVIPER: A fast and scalable toolkit for the identification of dysregulated proteins in tumor-derived single-cell RNA-seq data [abstract]. In: Proceedings of the American Association for Cancer Research Annual Meeting 2024; Part 1 (Regular Abstracts); 2024 Apr 5-10; San Diego, CA. Philadelphia (PA): AACR; Cancer Res 2024;84(6_Suppl):Abstract nr 7410.
更多查看译文
AI 理解论文
溯源树
样例
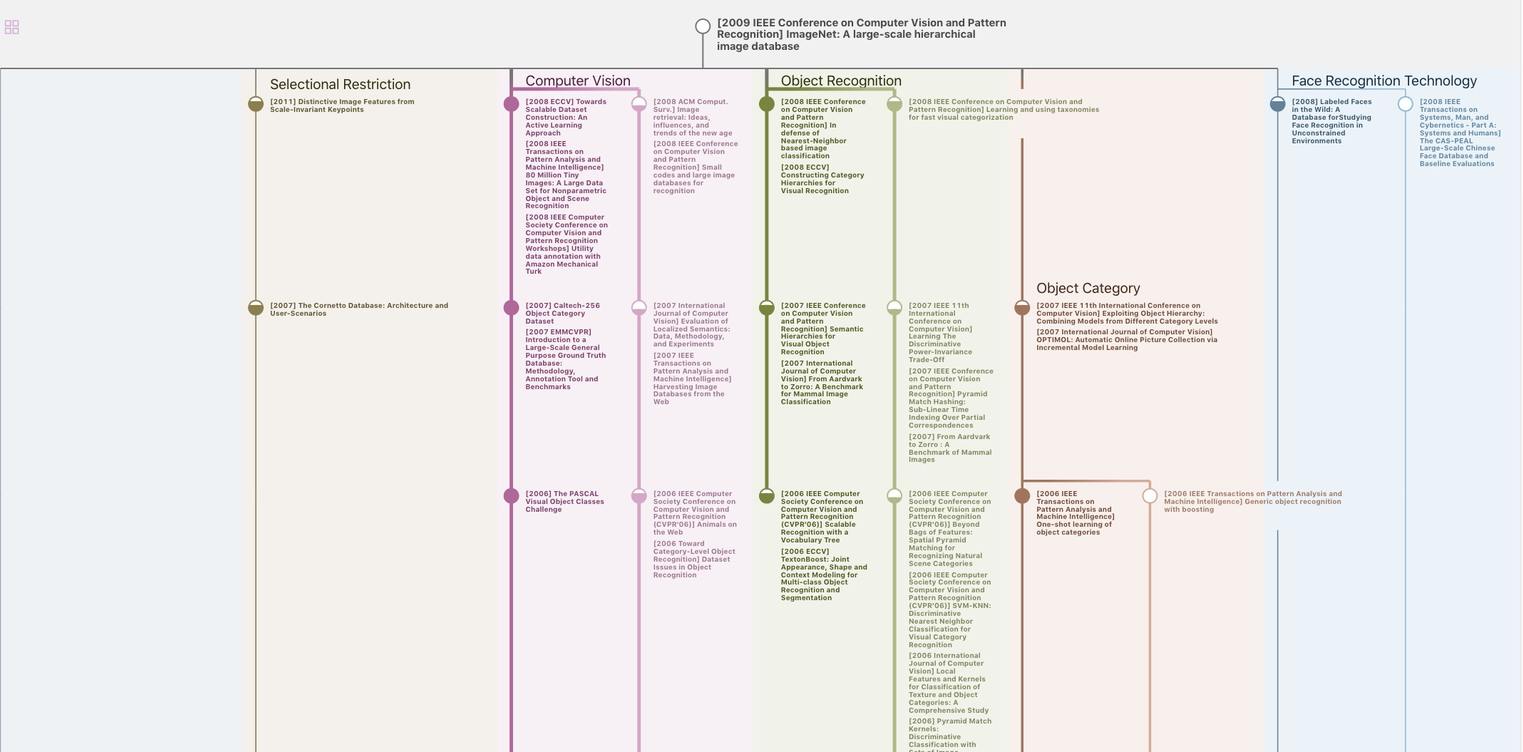
生成溯源树,研究论文发展脉络
Chat Paper
正在生成论文摘要