Generation model meets swin transformer for unsupervised low-dose CT reconstruction
MACHINE LEARNING-SCIENCE AND TECHNOLOGY(2024)
摘要
Computed tomography (CT) has evolved into an indispensable tool for clinical diagnosis. Reducing radiation dose crucially minimizes adverse effects but may introduce noise and artifacts in reconstructed images, affecting diagnostic processes for physicians. Scholars have tackled deep learning training instability by exploring diffusion models. Given the scarcity of clinical data, we propose the unsupervised image domain score generation model (UISG) for low-dose CT reconstruction. During training, normal-dose CT images are utilized as network inputs to train a score-based generative model that captures the prior distribution of CT images. In the iterative reconstruction, the initial CT image is obtained using a filtered back-projection algorithm. Subsequently, diffusion-based prior, high-frequency convolutional sparse coding prior, and data-consistency steps are employed to obtain the high-quality reconstructed image. Given the global characteristics of noise, the score network of the diffusion model utilizes a swin transformer structure to enhance the model's ability to capture long-range dependencies. Furthermore, convolutional sparse coding is applied exclusively to the high-frequency components of the image, to prevent over-smoothing or the loss of crucial anatomical details during the denoising process. Quantitative and qualitative results indicate that UISG outperforms competing methods in terms of denoising and generalization performance.
更多查看译文
关键词
low-dose CT,score-based diffusion model,swin transformer,convolutional sparse coding,data consistency
AI 理解论文
溯源树
样例
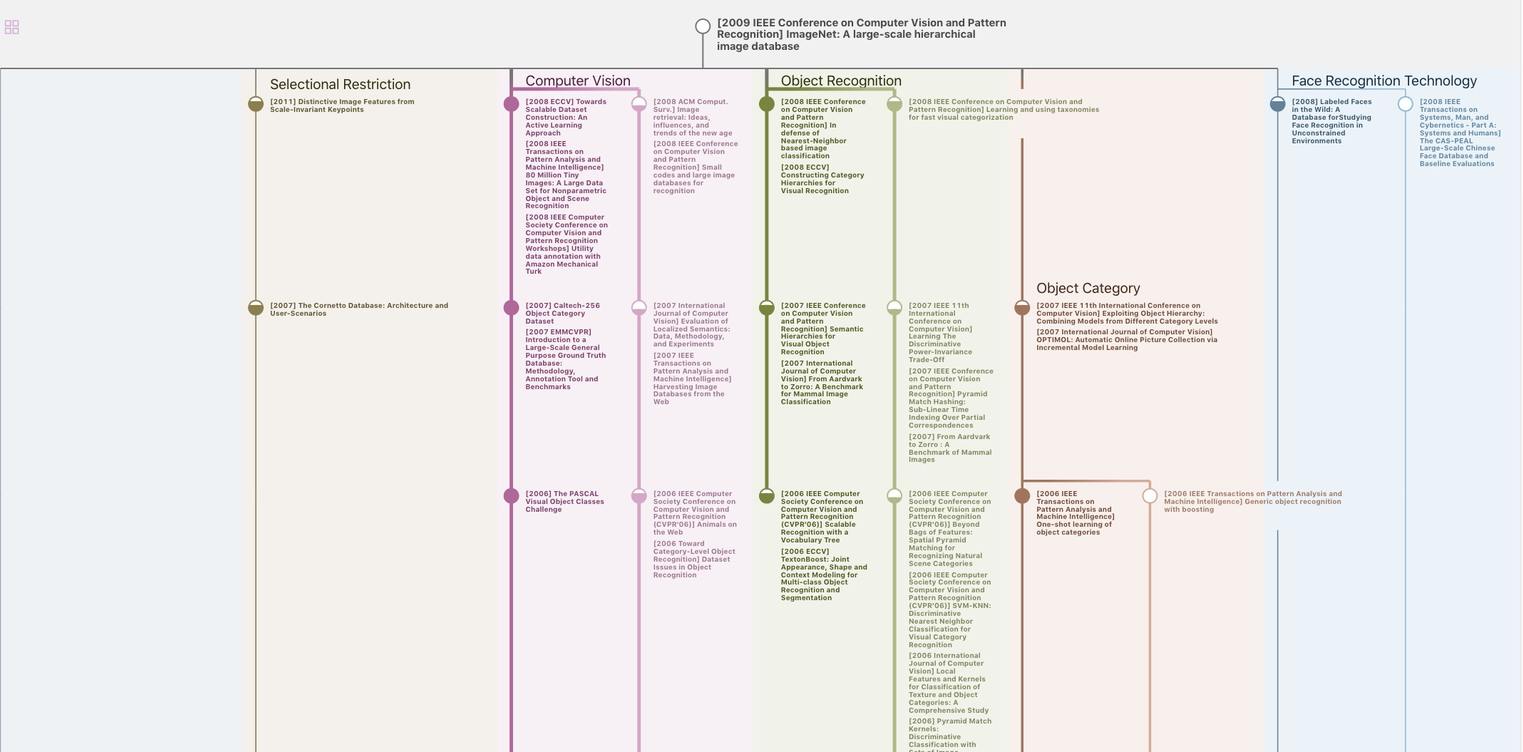
生成溯源树,研究论文发展脉络
Chat Paper
正在生成论文摘要