Assessing Membership Leakages via Task-Aligned Divergent Shadow Datasets in Vehicular Road Cooperation
IEEE Internet of Things Journal(2024)
摘要
Deep classification models have been widely utilized in Vehicular Road Cooperation. However, previous work indicates that deep classification models are vulnerable to the privacy risks of Membership Inference Attacks (MIAs). Most existing work of MIAs is based on two different assumptions. One assumes adversary-own shadow datasets with aligned tasks and distributions as private datasets, while this assumption necessitates that the adversary knows the distributions of private datasets. The other assumes adversary-own shadow datasets with distinct tasks and distributions from private datasets, while this assumption requires that the adversary knows the classification boundaries between members and non-members of the private dataset. Hence, these two assumptions do not always hold in real-world scenarios. In this work, we systematically assess the impact of adversaryown shadow datasets with aligned tasks but distinct distributions from private datasets on MIAs. These realistic shadow datasets acknowledge adversaries limited insights into the data distribution of the private dataset and the decision boundary between members and non-members. We divide these practical shadow datasets hosted by adversaries into 4 types: Data Noise, Label Noise, Imbalanced Data, and Cross-domain Data. We conduct extensive experiments with 7 prevalent MIAs and 4 types of shadow datasets. Experimental results mainly reveal two-fold findings. First, MIAs still maintain effectiveness using the shadow dataset with the aligned task but distinct distributions from the private dataset. Second, different levels of data distribution disparities manifest varying MIAs’ performances under certain types of shadow datasets.
更多查看译文
关键词
Membership Inference Attacks,Privacy,Deep Learning,Vehicular Road Cooperation,Shadow Dataset
AI 理解论文
溯源树
样例
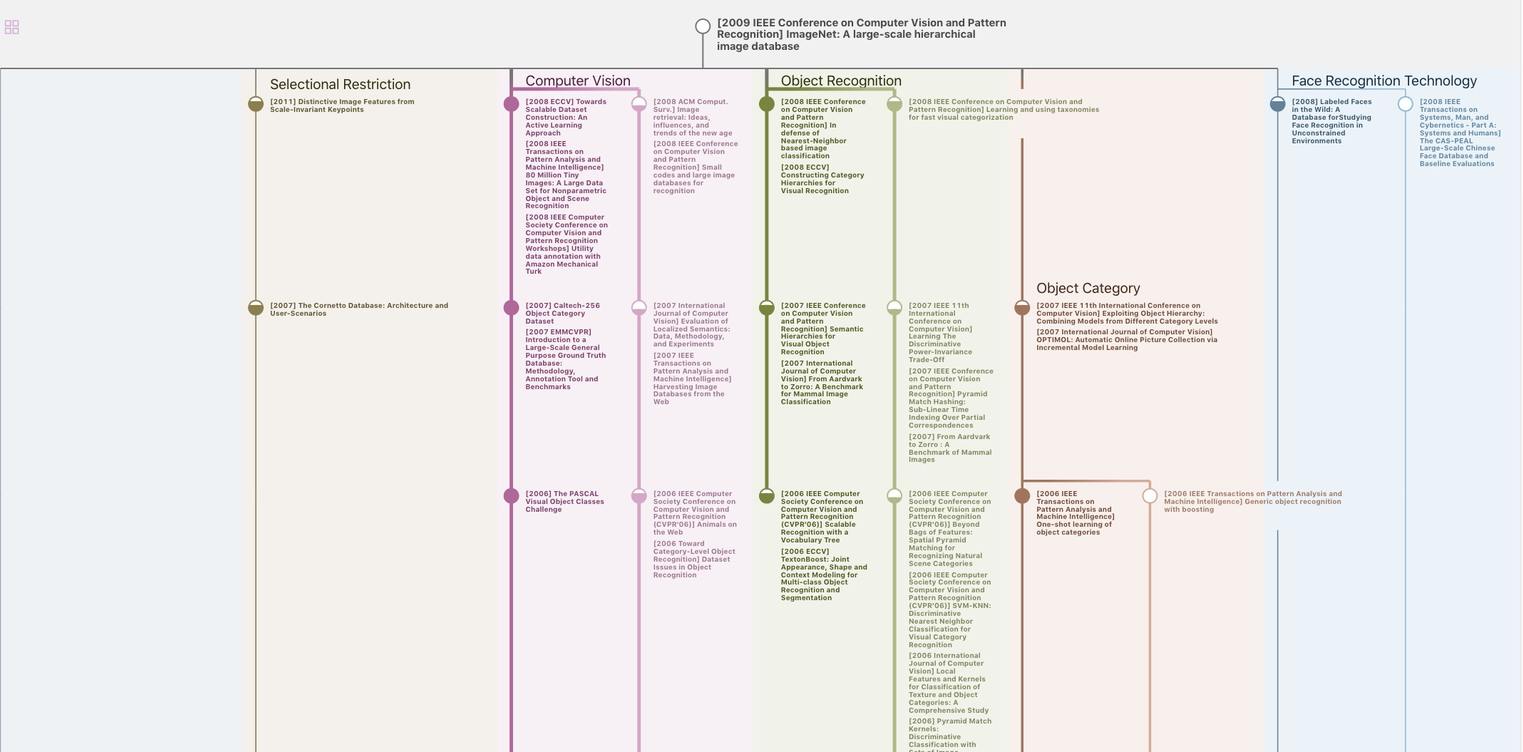
生成溯源树,研究论文发展脉络
Chat Paper
正在生成论文摘要