A modular framework for treatment effect estimation in latent subgroups
crossref(2024)
摘要
Abstract Latent subgroups arise when patients are randomized to an intended treatment, that can only be given for certain, treatable, patients. For biological efficacy, the relevant estimand is then the treatment effect in the subgroup of treatable patients, with the obvious issue that this subgroup is latent, identified only in the intervention arm. We present a modular framework for effect estimation in such latent subgroups. The framework consists of a core and three plug-in models, for subgroup membership and outcomes among treatable and non-treatable patients. The core computes maximum likelihood estimates using the EM algorithm, together with standard errors. It does so without any knowledge about the details of the plug-in models, giving the user great flexibility. The methods are implemented in an R package. The framework is validated in a simulation, where we also explore the use of predictors. Particularly intriguing are predictors of treatability, partly identifying the latent subgroup from baseline data. The results suggest that this can dramatically increase the power, while being robust against model misspecifications. Finally, the methods are applied to a prostate cancer trial.
更多查看译文
AI 理解论文
溯源树
样例
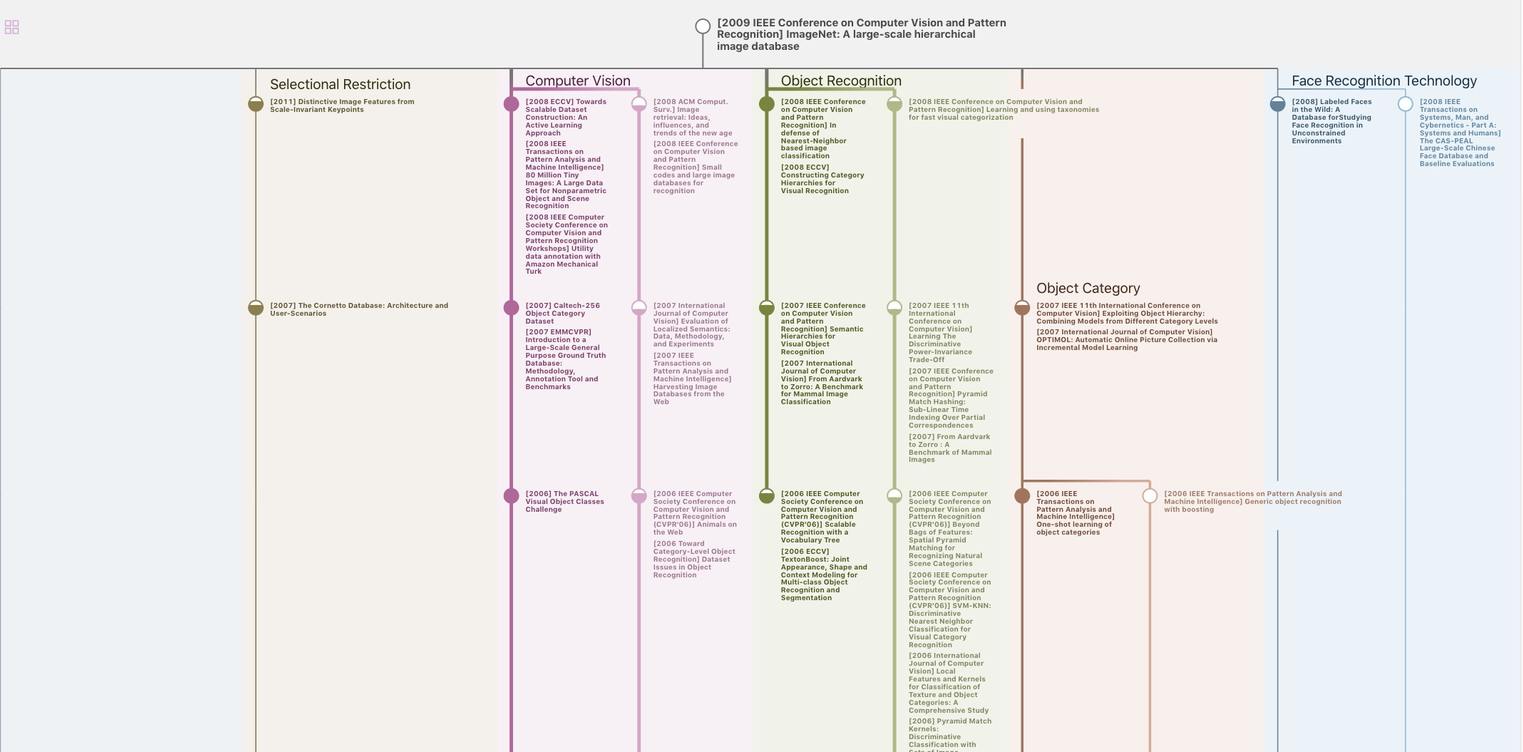
生成溯源树,研究论文发展脉络
Chat Paper
正在生成论文摘要