Abstract 1141: Spatial-specific gene signatures to predict immunotherapy outcomes in lung cancer
Cancer Research(2024)
摘要
Abstract The widespread use of immunotherapy in lung cancer, and its more recent approval for early stages, underscores the need for biomarkers that can identify the most responsive patients. Spatial transcriptomics, which maps gene expression in its spatial tissue context, offers a unique approach over bulk transcriptomics. By incorporating spatial information, the predictive power of the signature can be enhanced. Here, we aim to develop spatially informed gene signatures that could be translated into clinical RNA in situ assays, distinguishing patients unlikely to benefit from immunotherapy and thereby sparing them unnecessary side effects. We utilized the NanoString GeoMX Whole Transcriptome Atlas for spatially resolved transcriptomic profiling of retrospectively collected lung cancer tissue samples from patients treated with immunotherapy in an advanced-stage setting (N=60). By targeting 18,190 genes within distinct areas of interest (AOIs)—including stromal (macrophages/CD68+ and leukocytes/CD45+) and tumor (cytokeratin, CK+) cells—we developed AOI-specific gene signatures to predict objective responses. These were derived from a robust computational framework employing LASSO logistic regression on a split-sample approach, yielding predictive models for treatment outcome.We achieved high predictive accuracy on the training set, with the area under the curve (AUC) exceeding 0.86 for all AOI-specific spatial signatures, indicating strong potential for clinical application. Validation against an independent cohort (N=42) corroborated the efficacy of these signatures. Our 6-gene tumor signature was validated with an AUC of 0.73 (95% CI: 0.67-0.89, p = 0.009**), while the CD45 5-gene signature showed an AUC of 0.75 (95% CI: 0.53-0.97, p = 0.022*). Our 18-gene CD68 signature trended towards validation but lacked statistical significance. A combined CD68 and CD45 signature predicted outcomes with greater accuracy, achieving an AUC of 0.79 (95% CI: 0.62-0.98, p = 0.0088*). Following gene set enrichment analysis on our differentially expressed and signature genes, we identified genes with positive coefficients in both tumor and stroma signatures that were associated with glucocorticoid response and glycolytic processes linked to T-cell homeostasis, while genes with negative coefficients were associated with epithelial cell differentiation and cytokine production. These associations are concordant with the observation that genes with positive coefficients are predictors of treatment response, while those with negative coefficients indicate resistance.Our findings indicate that AOI-specific signatures predict the immunotherapy outcome in lung cancer with high accuracy, suggesting that spatial assessment can provide substantial predictive information. The high performance of these signatures indicates their potential for prospective clinical applications. Citation Format: Thazin N. Aung, Myrto Moutafi, Ioannis Trontzas, Arutha Kulasinghe, James Monkman, Niki Gavrielatou, Ioannis Vathiotis, Jonathan H. Warrell, David L. Rimm. Spatial-specific gene signatures to predict immunotherapy outcomes in lung cancer [abstract]. In: Proceedings of the American Association for Cancer Research Annual Meeting 2024; Part 1 (Regular Abstracts); 2024 Apr 5-10; San Diego, CA. Philadelphia (PA): AACR; Cancer Res 2024;84(6_Suppl):Abstract nr 1141.
更多查看译文
AI 理解论文
溯源树
样例
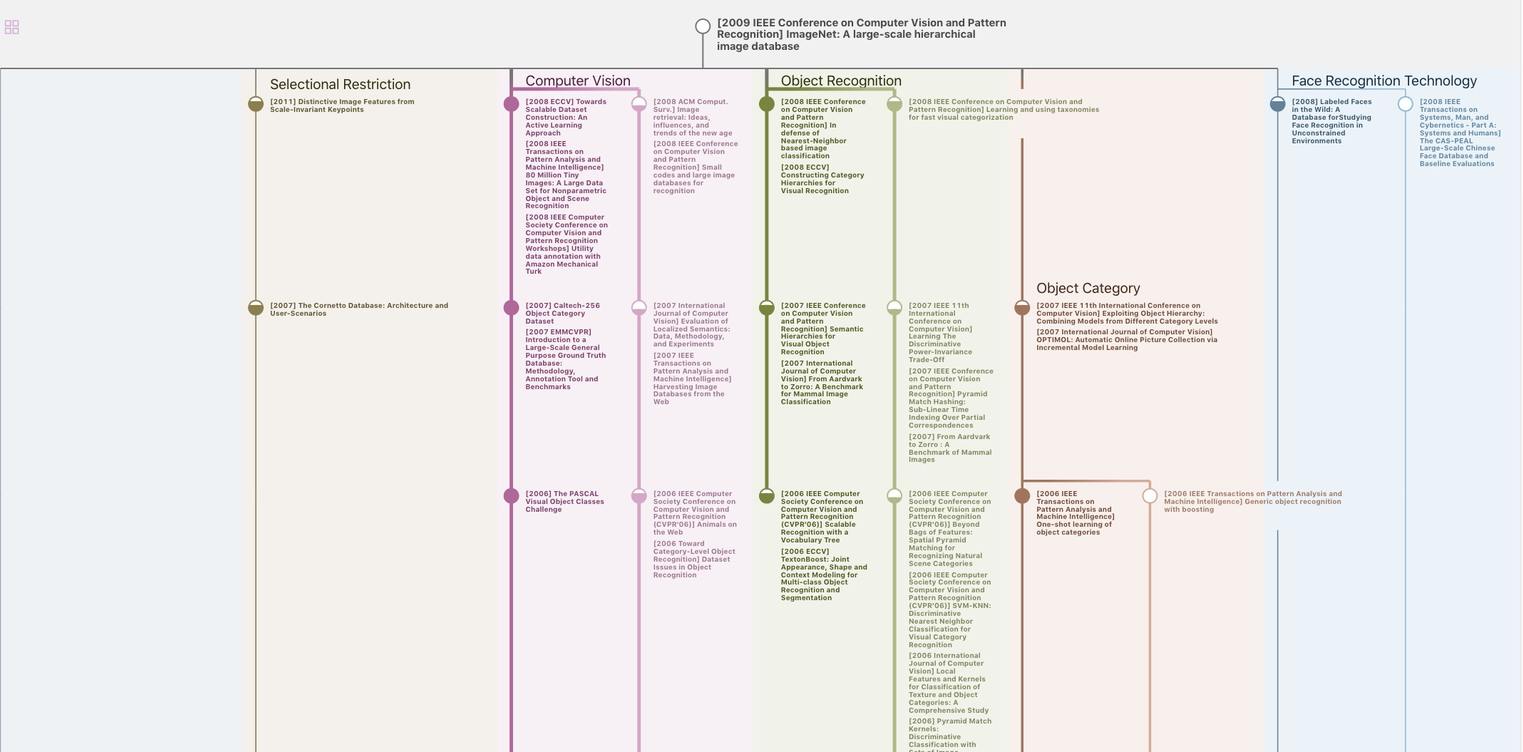
生成溯源树,研究论文发展脉络
Chat Paper
正在生成论文摘要