Abstract 3799: Spatial biomarker prediction in papillary renal cell carcinoma using artificial intelligence-based immunofluorescent inference
Cancer Research(2024)
摘要
Abstract Papillary renal cell carcinoma (pRCC) accounts for ~15-20% of the global burden for kidney cancers but still lacks effective mechanisms to predict patient responses to therapeutic intervention. Existing therapies targeting kidney cancers (anti-angiogenesis and immunotherapy) intimately involve the tumor microenvironment in their mechanism of action. However, the role of the physical organization of the tumor in determining therapeutic efficacy remains unknown. Previous efforts have utilized transcriptional profiling to stratify patients into treatment categories but here we propose to leverage artificial neural networks (“AI”) to infer the expression of marker genes for particular cell types of interest based on ubiquitously expressed immunofluorescence channels, unstained brightfield images, and hematoxylin and eosin (H&E) stained images. We have developed AI models that are capable of the prediction of a range of relevant cell types based on the expression profile of DAPI, type IV collagen, and integrin beta-1, including endothelial cells, T cells, macrophages and other cell types. This work applies an AI model class known as generative adversarial networks (GANs) to produce photo-realistic simulated immunofluorescence images based on the input channels. This approach may allow us to accurately predict complex multiplex immunofluorescence (mIF) images from simpler, more routine histological methods, greatly reducing the cost burden required to profile histopathology images for cell types of interest. Our previous work identified a number of interesting cell-cell association clusters correlated with patient prognosis and this extension of that previous work aims to allow for the prediction of these interactions while obviating the need to perform the original, costly multiplex immunofluorescence assay or even a more reduced immunofluorescence staining panel which may still be out of reach for many clinical pathology labs and the interpretation of which may be challenging. By simplifying and increasing the availability of predictive AI models for digital pathology, we hope to further develop and apply predictive biomarkers for patient prognosis and treatment response in papillary renal cell carcinoma. Citation Format: Jared Brewer, Joseph Vento, Scott Haake, Anupama Reddy. Spatial biomarker prediction in papillary renal cell carcinoma using artificial intelligence-based immunofluorescent inference [abstract]. In: Proceedings of the American Association for Cancer Research Annual Meeting 2024; Part 1 (Regular Abstracts); 2024 Apr 5-10; San Diego, CA. Philadelphia (PA): AACR; Cancer Res 2024;84(6_Suppl):Abstract nr 3799.
更多查看译文
AI 理解论文
溯源树
样例
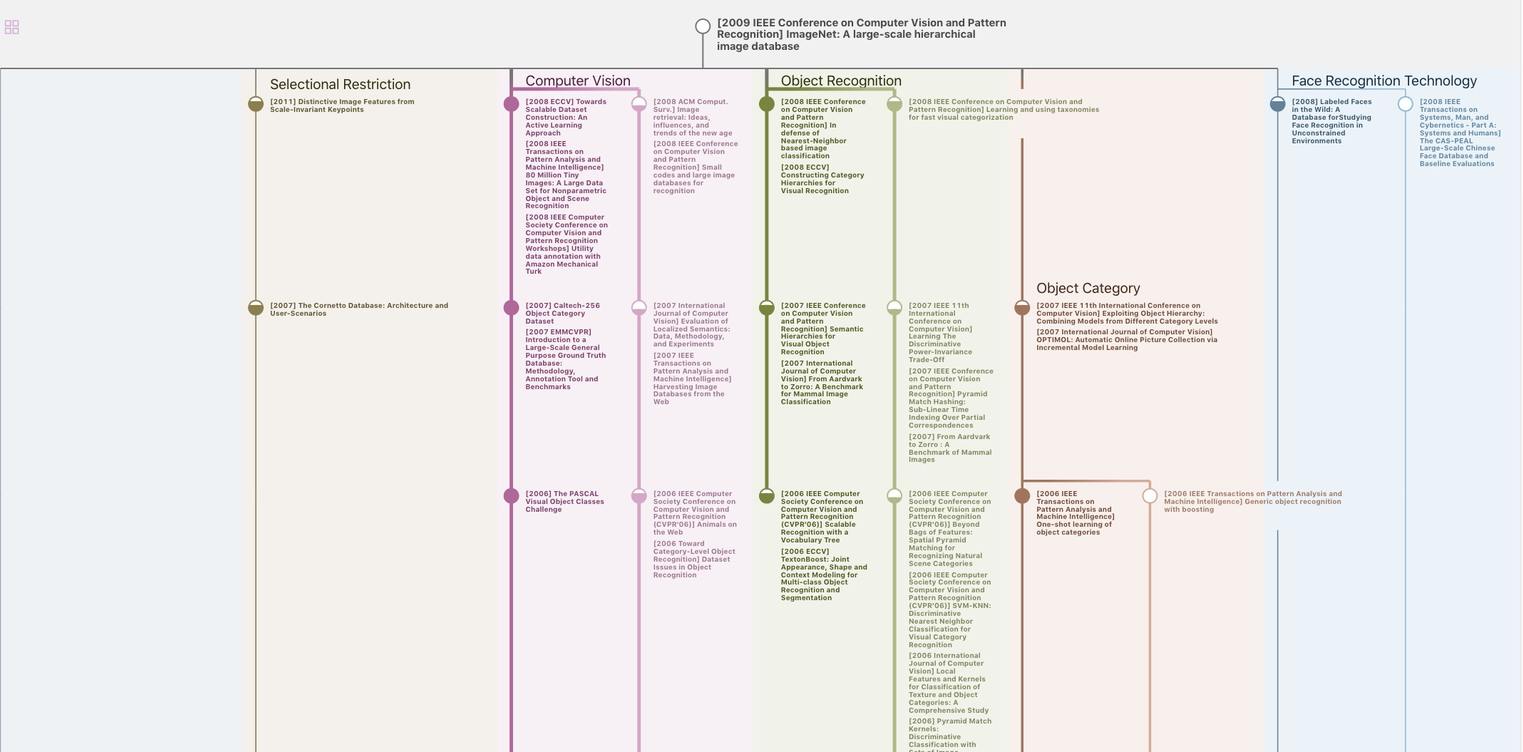
生成溯源树,研究论文发展脉络
Chat Paper
正在生成论文摘要