FTSDC: A novel federated transfer learning strategy for bearing cross-machine fault diagnosis based on dual-correction training
Advanced Engineering Informatics(2024)
摘要
In recent years, although traditional intelligent fault diagnosis methods have achieved satisfactory development in transfer learning tasks, the sample information that the single client can generally provide is extremely limited in real industrial scenarios. And the private data needs to be guaranteed not to leave the local storage during the application process, which leads to obstacles for fault diagnosis methods to solve cross-device and cross-scenario client transfer tasks. Therefore, a novel federated transfer learning strategy based on dual-correction training (FTSDC) is proposed, which enables fault diagnosis for multi-device tasks without target domain samples to participate in model training. The dual correction training of source client is proposed to enhance the local network’s generality, which involves two links: The multiple-functions correction model hyperparameters link and the transition training correction network attention area link. Multiple sets of optimization functions are introduced into local network training to reduce the covariate drift phenomenon caused by domain discrepancies. And the local model fine-tunes the parameters of the trained network through the transition dataset to correct attention areas. Furthermore, The central server evaluates each source client model according to the contribution of the local model to the transfer strategy, and the local model parameters are cumulatively optimized by referring to the diagnosic experience of the global model. It is worth noting that the trained local model can still demonstrate commendable performance even when faced with more complex scenarios. Finally, the proposed scheme was rigorously assessed against diverse federated learning methods across various task settings during the experimental session. The results confirmed the effectiveness of the proposed scheme in safeguarding the data privacy of each client while concurrently enhancing the diagnostic accuracy of the target task.
更多查看译文
关键词
Domain adaptation,Federated transfer learning,Data privacy,Fault diagnosis,Rotating machines
AI 理解论文
溯源树
样例
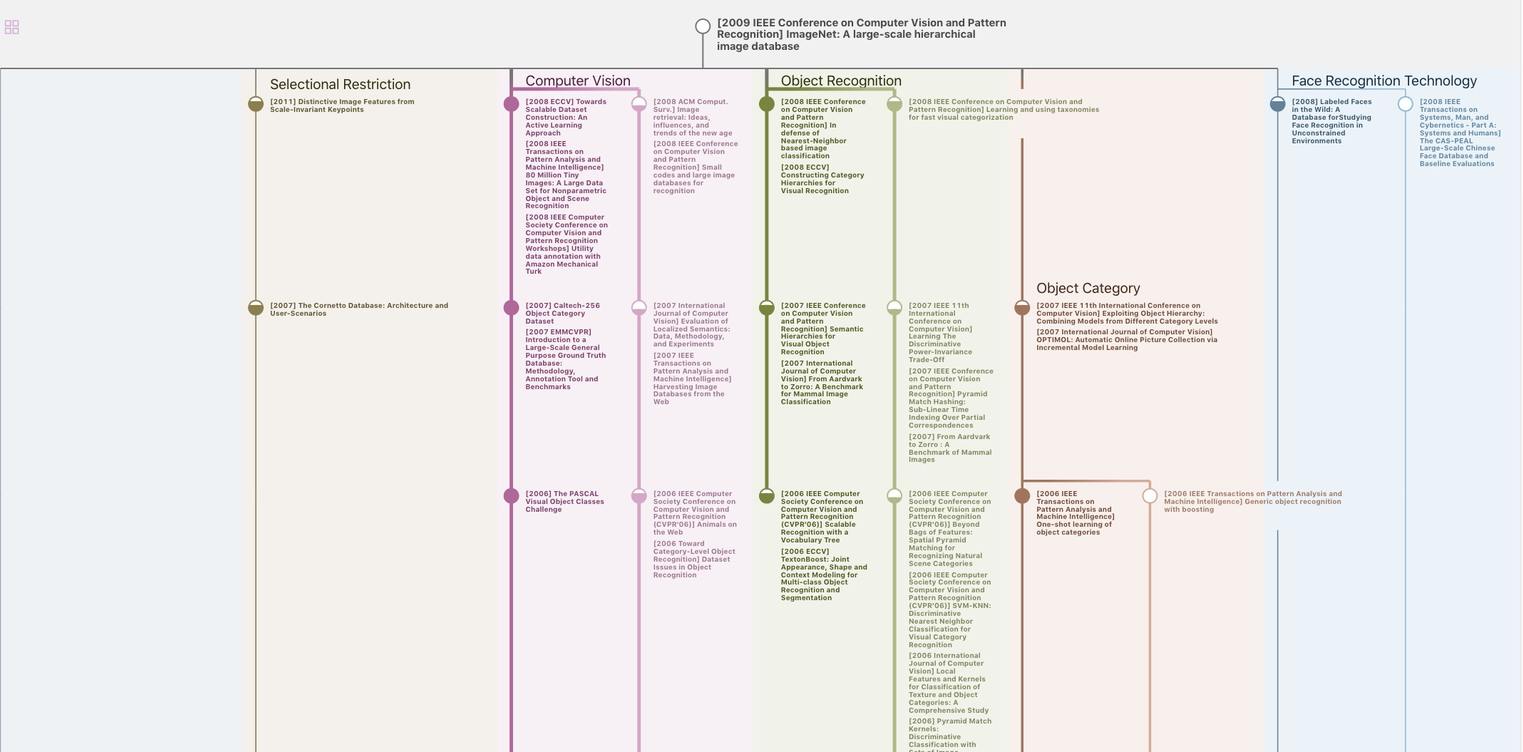
生成溯源树,研究论文发展脉络
Chat Paper
正在生成论文摘要