A two-stage multi-view partial multi-label learning for enhanced disambiguation
Knowledge-Based Systems(2024)
摘要
Multi-view partial multi-label (MVPML) problems are often encountered in our real life. Each training sample described by multi-view is associated with a set of candidate labels, including multiple ground-truth labels and noisy labels. Currently, to tackle the problem, some attempts are focusing on label enhancement. Moreover, disambiguation is a relatively valid method to recover the ground-truth label by disambiguating the candidate label for each sample. However, due to the complexity of the data, the one-stage disambiguation method is hard to fully recover the ground-truth label. To deal with the challenge, we propose a novel two-stage MVPML method motivated by feature-induced manifold disambiguation and low-rank sparse decomposition (TFMDD). Specifically, in the first stage, the KNN-based aggregate manifold structure adaptively fuses feature information from different views, and the obtained structure information is used to build a feature-induced manifold structure in the label space to remove the ambiguity of candidate labels. In the second stage, under the supervision of the disambiguated label matrix, multi-label classifiers and noise recognizers are simultaneously optimized in a unified framework by considering label correlation and low noise levels. Through the two stages, TFMDD attains satisfactory results. Extensive experiments on real-world datasets clearly confirm the method validity and give a validation in a statistical sense.
更多查看译文
关键词
Multi-view,Partial multi-label,Label disambiguation,Low-rank,Sparse
AI 理解论文
溯源树
样例
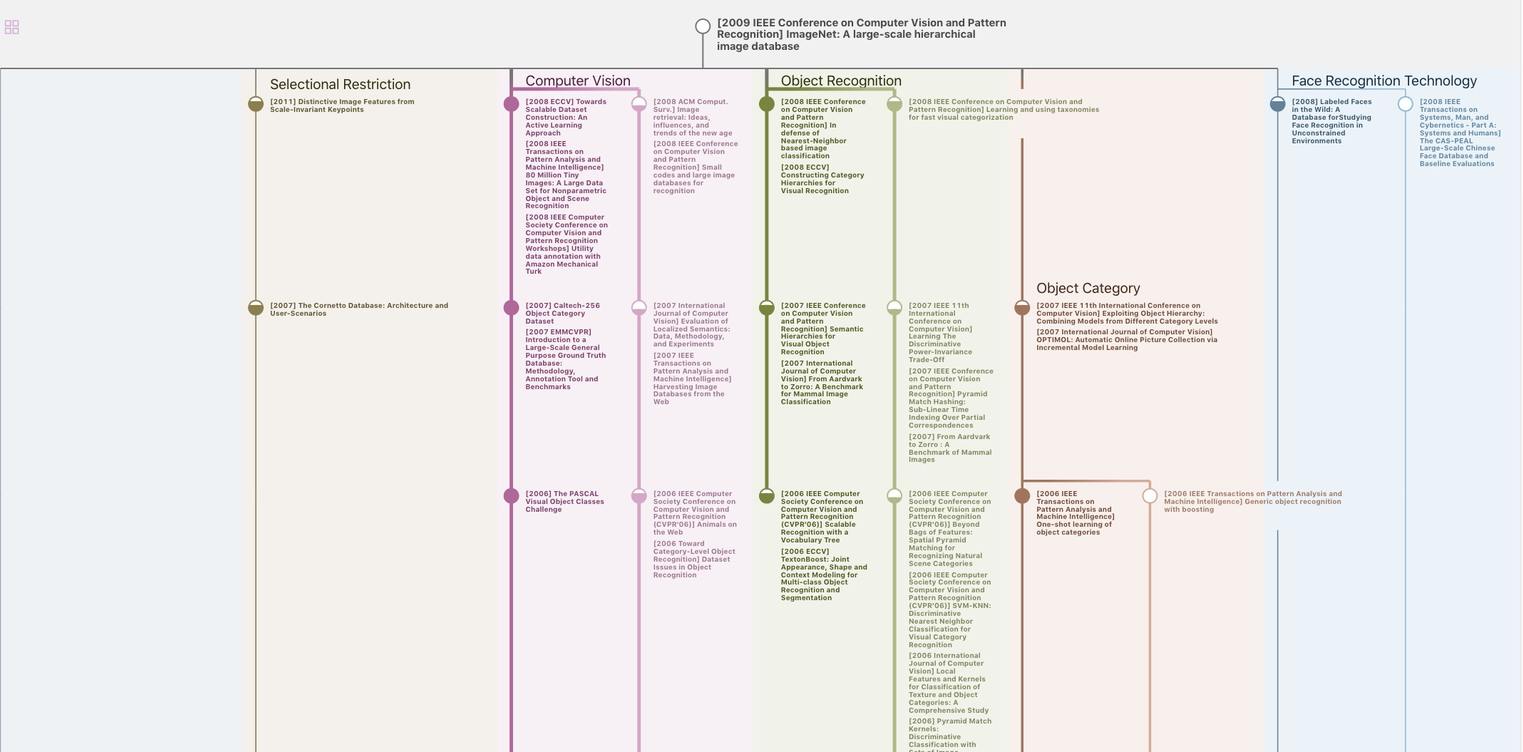
生成溯源树,研究论文发展脉络
Chat Paper
正在生成论文摘要