Anomaly Detection and Fault Disambiguation in Large Flight Data: A Multi-modal Deep Auto-encoder Approach
Annual Conference of the PHM Society(2016)
摘要
Flight data recorders provide large volumes of heterogeneous data from arrays of sensors on-board to perform fault diagnosis. Challenges such as large data volumes, lack of labeled data, and increasing numbers of sensors (multiple modalities) exacerbate the challenges of being able to hand-craft the features needed for state-of-the-art PHM algorithms to effectively perform system diagnosis. In this paper, the authors propose leveraging existing unsupervised learning methods based on Deep Auto-encoders (DAE) on raw time series data from multiple sensors to build a robust model for anomaly detection. The anomaly detection algorithm analyzes the reconstruction error of a DAE trained on nominal data scenarios. The reconstruction error of individual sensors is examined to perform fault disambiguation. Training and validation are conducted in a laboratory setting for various operating conditions. The proposed framework does not need any hand-crafted features and uses raw time series data. Our approach is tested on data from the NASA open database and demonstrates high fault detection rates (~ 97:8%) with zero false alarms. Our paper also demonstrates robust fault disambiguation on two different fault scenarios. Moreover, the paper provides a strong rationale for utilizing deep architecture (multi-hidden-layer neural network) via thorough comparison with a single hidden-layer DAE.
更多查看译文
AI 理解论文
溯源树
样例
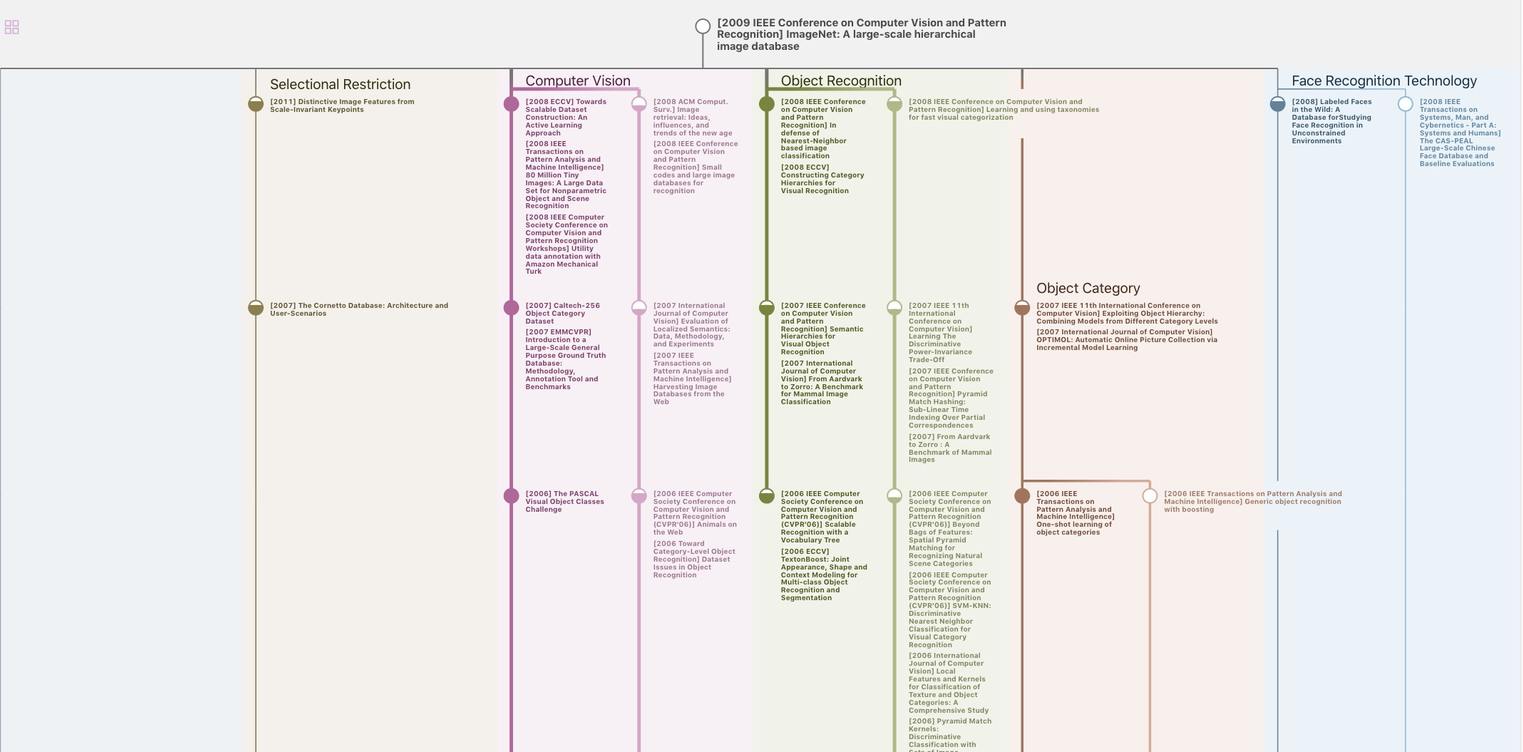
生成溯源树,研究论文发展脉络
Chat Paper
正在生成论文摘要