Hierarchical Federated Edge Learning over Space-Air-Ground Integrated Networks.
GLOBECOM (Workshops)(2023)
摘要
Federated learning (FL), as a key application scenario to support distributed artificial intelligence (AI) services, allows an amount of edge devices to train a global model in tandem. In addition, space-air-ground (SAG) integrated networks are envisioned to provide AI services for remote areas in the next generation of wireless communication system, which provides new opportunities for the integration of SAG and FL. Whereas, the performance of the SAG-FL is limited by significant system latency, high energy consumption, and slow convergence rate. In this paper, we consider a SAG hierarchical FL network, where a low-earth-orbit (LEO) satellite serves as the cloud server and multiple unmanned aerial vehicles (UAVs) act as the edge nodes covering multiple cells of edge devices. Specifically, we provide the convergence analysis of the SAG-FL algorithm, and then alternatively optimize the device scheduling policy and UAVs' trajectory to minimize the system delay and energy consumption between two global aggregations. Simulation results illustrate that the proposed dynamic device scheduling policy is much more time-saving and energy-efficient than the static one.
更多查看译文
关键词
Energy Consumption,Convergence Rate,Energy System,Cloud Computing,Unmanned Aerial Vehicles,High Energy Consumption,System Delay,Federated Learning,Edge Devices,System Energy Consumption,Slow Convergence Rate,Multiple Unmanned Aerial Vehicles,Optimization Problem,Time Constraints,Internet Of Things,Learning Performance,Time Slot,ReLU Activation,Internet Of Things Devices,Computational Capabilities,Unmanned Aerial Vehicle Trajectory,Edge Server,Energy Constraints,Local Dataset,Trajectory Optimization,Communication Rounds,Time Division Multiple Access,Uplink Transmission,Computation Energy,Delay Reduction
AI 理解论文
溯源树
样例
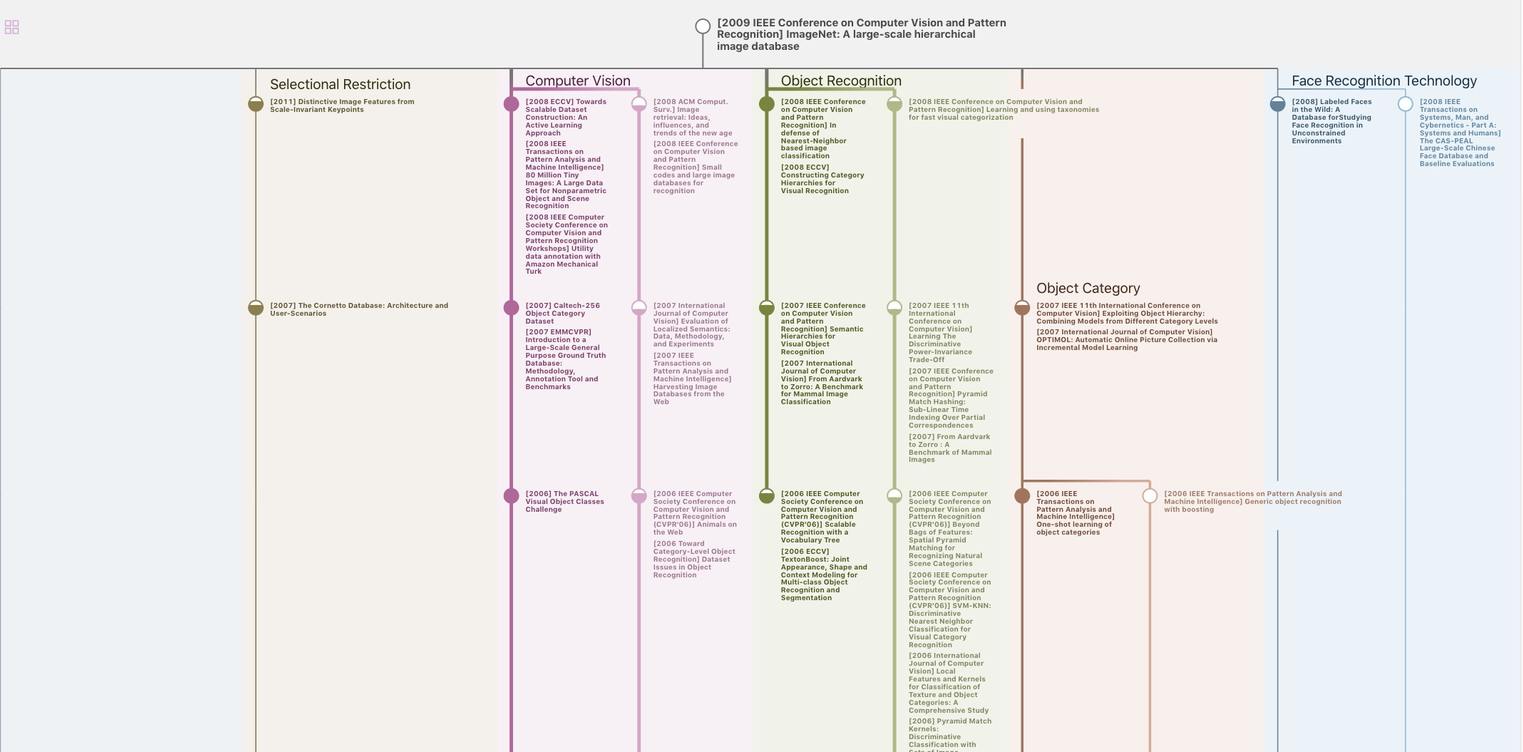
生成溯源树,研究论文发展脉络
Chat Paper
正在生成论文摘要