Hybrid physics data-driven model-based fusion framework for machining tool wear prediction
The International Journal of Advanced Manufacturing Technology(2024)
摘要
Accurate tool wear prediction is of great significance to improve production efficiency, ensure product quality and reduce machining cost. This paper proposes a hybrid physics data-driven model-based fusion framework for tool wear prediction to improve low prediction accuracy of physical model and poor interpretation of data-driven model. In this framework, physical information and local features of sensor measurement signals are used as inputs to build a hybrid physics data-driven (HPDD) model. And data mining and physics principles are effectively integrated by using unlabeled samples for data expansion. Piecewise prediction is introduced to reduce difficulty in parameter estimation. Then, in order to manage prediction uncertainty of physical information and HPDD method, two prediction results are gradually combined based on Bayesian fusion mechanism to eliminate prediction error. Finally, the effectiveness of the proposed method is verified by experiment. Compared with existing methods, this method significantly improves prediction. The mean values of root mean square error (RMSE) and mean relative error (MARE) for tool wear prediction results are respectively 2.28 and 1.85.
更多查看译文
关键词
Tool wear prediction,Physical information,Hybrid physics data-driven model,Fusion framework,Bayesian method
AI 理解论文
溯源树
样例
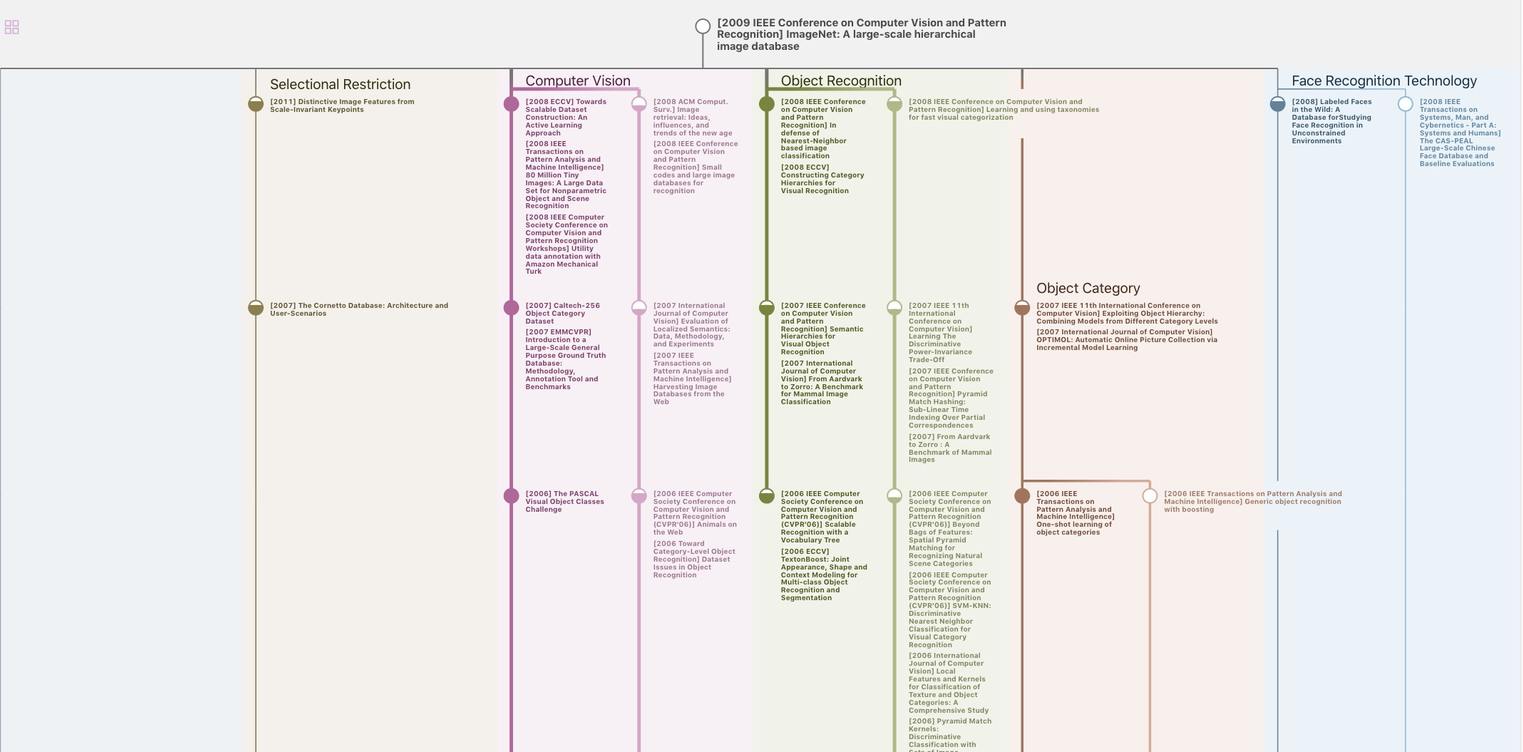
生成溯源树,研究论文发展脉络
Chat Paper
正在生成论文摘要