Automated prostate gland segmentation in challenging clinical cases: comparison of three artificial intelligence methods
Abdominal Radiology(2024)
摘要
Automated methods for prostate segmentation on MRI are typically developed under ideal scanning and anatomical conditions. This study evaluates three different prostate segmentation AI algorithms in a challenging population of patients with prior treatments, variable anatomic characteristics, complex clinical history, or atypical MRI acquisition parameters. A single institution retrospective database was queried for the following conditions at prostate MRI: prior prostate-specific oncologic treatment, transurethral resection of the prostate (TURP), abdominal perineal resection (APR), hip prosthesis (HP), diversity of prostate volumes (large ≥ 150 cc, small ≤ 25 cc), whole gland tumor burden, magnet strength, noted poor quality, and various scanners (outside/vendors). Final inclusion criteria required availability of axial T2-weighted (T2W) sequence and corresponding prostate organ segmentation from an expert radiologist. Three previously developed algorithms were evaluated: (1) deep learning (DL)-based model, (2) commercially available shape-based model, and (3) federated DL-based model. Dice Similarity Coefficient (DSC) was calculated compared to expert. DSC by model and scan factors were evaluated with Wilcox signed-rank test and linear mixed effects (LMER) model. 683 scans (651 patients) met inclusion criteria (mean prostate volume 60.1 cc [9.05–329 cc]). Overall DSC scores for models 1, 2, and 3 were 0.916 (0.707–0.971), 0.873 (0–0.997), and 0.894 (0.025–0.961), respectively, with DL-based models demonstrating significantly higher performance (p < 0.01). In sub-group analysis by factors, Model 1 outperformed Model 2 (all p < 0.05) and Model 3 (all p < 0.001). Performance of all models was negatively impacted by prostate volume and poor signal quality (p < 0.01). Shape-based factors influenced DL models (p < 0.001) while signal factors influenced all (p < 0.001). Factors affecting anatomical and signal conditions of the prostate gland can adversely impact both DL and non-deep learning-based segmentation models.
更多查看译文
关键词
Prostate,Magnetic resonance imaging,Segmentation,Machine learning,Deep learning
AI 理解论文
溯源树
样例
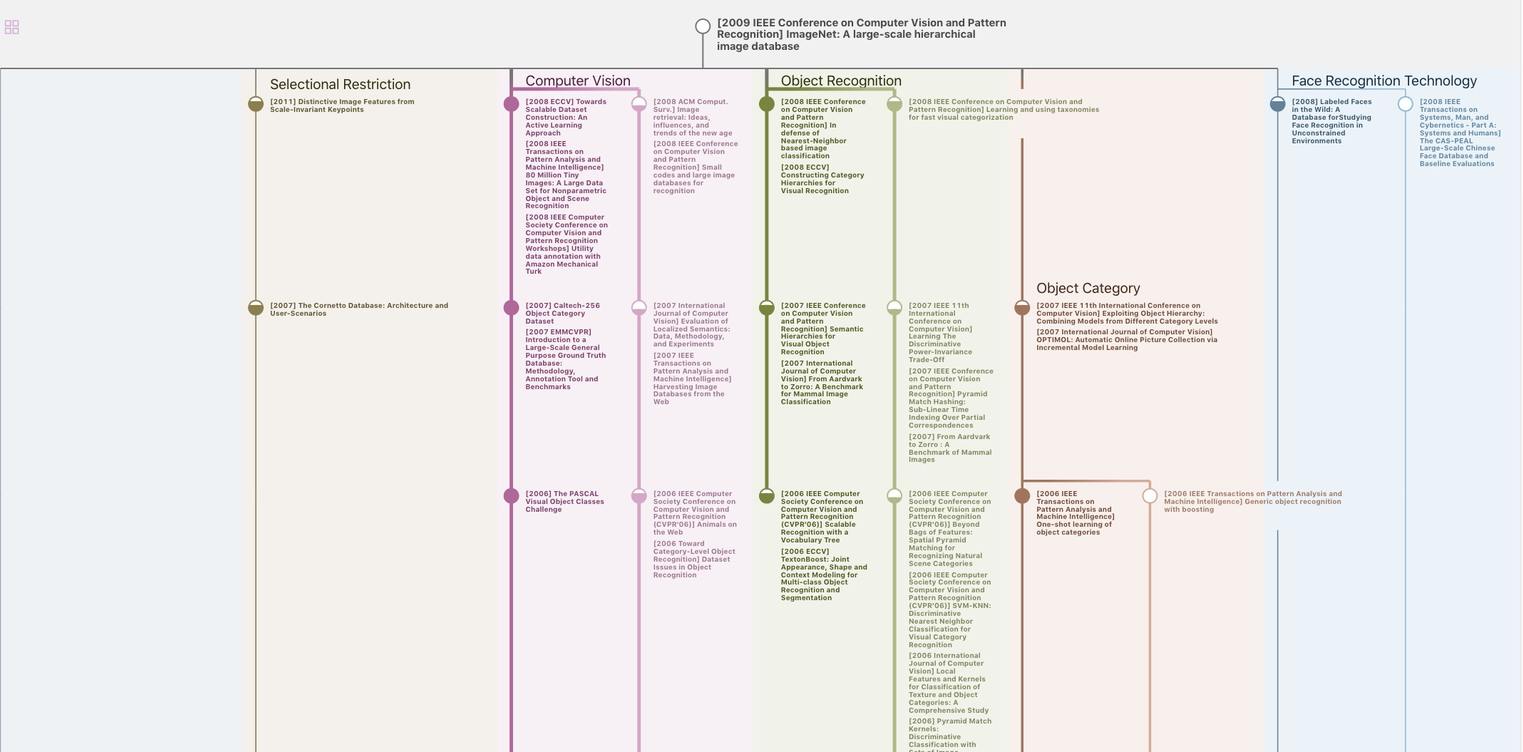
生成溯源树,研究论文发展脉络
Chat Paper
正在生成论文摘要