Fine-Tuning for Few-shot Image Classification by Multimodal Prototype Regularization
IEEE Transactions on Multimedia(2024)
摘要
Large pre-trained vision-language models, such as CLIP [1], have demonstrated remarkable performance in few shot image classification. To facilitate the rapid adaptation of CLIP in downstream tasks with limited visual samples, two primary frameworks have been proposed. The first framework centers on the image encoder and introduces a trainable visual classifier after the backbone to generate logits for each object class. Nevertheless, this framework heavily depends on limited visual features extracted by the pre-trained visual encoder, which can result in over-fitting issues. The second framework aims to optimize the text encoder by using trainable soft language prompts and computing logits for each class based on the similarity between image features and optimized prompt features. However, this framework encounters the issue of imperfect alignment between the representations extracted by the image and text encoders, making it difficult to fine-tune the language prompts using visual samples. This paper proposes a Multi- Modal Prototype Regularization (MMPR) method for CLIP based few-shot fine-tuning for image classification. MMPR can address the challenges of effectively utilizing both image and text features. MMPR fine-tunes a classifier and regularizes its weights using both image-based (ImgPR) and text-based (TexPR) prototypes. ImgPR represents the mean of image representations within the same class, derived from the image encoder, to distill specific visual distribution knowledge for classifier adaptation. TexPR represents the hand-crafted prompt associated with the class, derived from the text encoder, to incorporate general encyclopedic knowledge and mitigate visual over-fitting. MMPR significantly leverages both image and text information without increasing computational complexity during the inference stage compared to existing methods. Experimental results on various challenging public benchmarks demonstrate the superiority of the proposed MMPR method over state-of-the-art methods.
更多查看译文
关键词
Few-shot classification,large pre-trained vision language models,model fine-tuning,prototype regularization
AI 理解论文
溯源树
样例
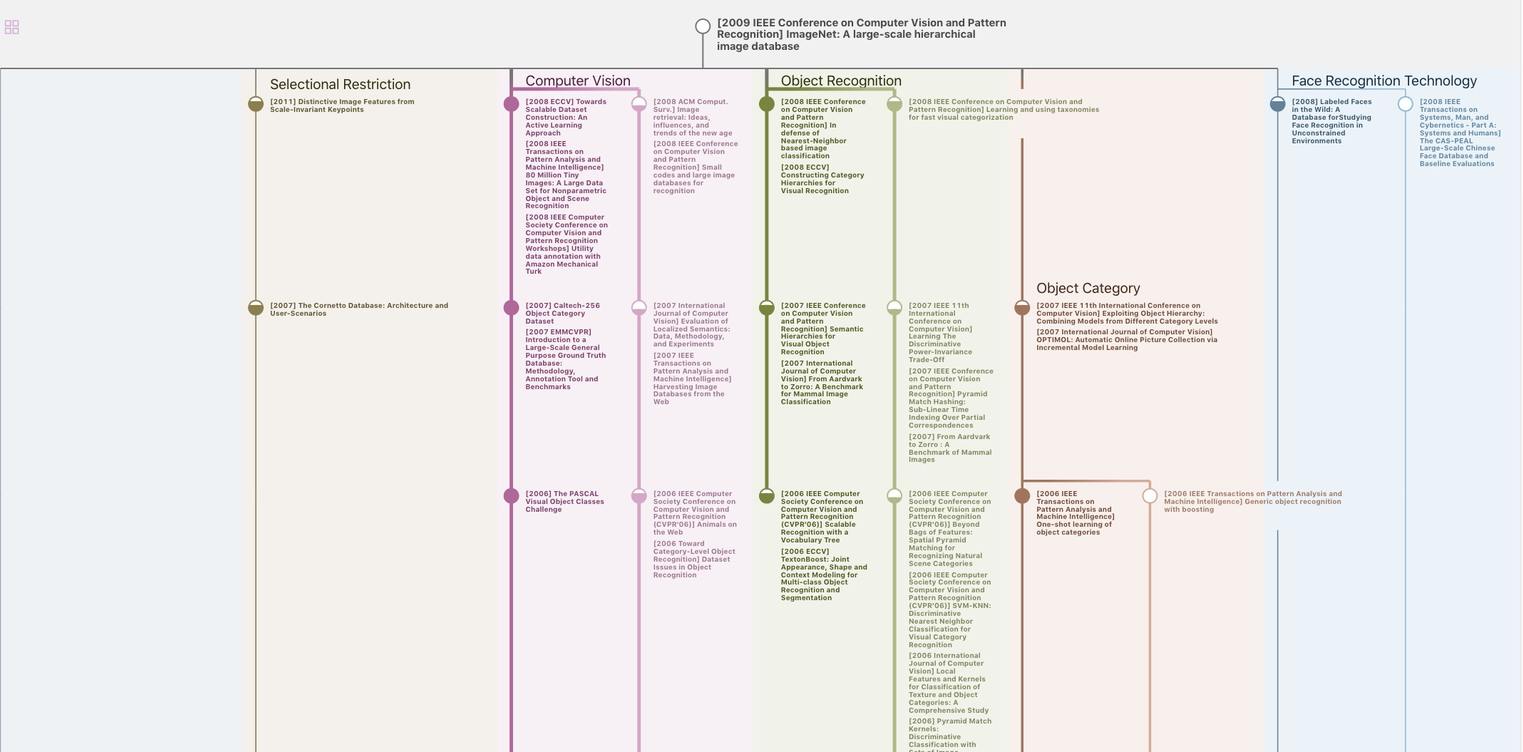
生成溯源树,研究论文发展脉络
Chat Paper
正在生成论文摘要