I2T: From Intention Decoupling to Vehicular Trajectory Prediction Based on Prioriformer Networks
IEEE TRANSACTIONS ON INTELLIGENT TRANSPORTATION SYSTEMS(2024)
摘要
A reliable driving trajectory prediction of surrounding vehicles is an essential reference for decision-making and safe driving of an autonomous vehicle. Although predicting short-term trajectories can be well achieved, it is still very challenging for long-term prediction of trajectories since the prediction space grows exponentially. In this paper, we propose a novel architecture for trajectory prediction from factored intention estimation (I2T), which decouples the trajectory prediction space into a high-level space for intention estimation and a low-level space for motion prediction. The long-term dependencies between intention cues and future motions during driving are naturally extended to the internal sharing mechanism of I2T, leading to improved performance. Furthermore, we design a Prioriformer model to serve as the backbone network for I2T so that it can accurately capture the long-term dependency couplings related to the task of intention estimation or motion prediction. Prioriformer model adopts a personalized normalization method, which facilitates learning latent representations of long-term features and avoids getting stuck on local optimum. A designed multi-scale fusion encoder extracts features from various receptive fields and then learns richer information from the representation subspaces. An efficient non-autoregressive decoder reduces the pressure in long-term prediction of trajectories while avoiding cumulative errors. Experiments on three real-world motion datasets show that I2T can significantly outperform the state-of-the-art.
更多查看译文
关键词
Trajectory,Feature extraction,Transformers,Predictive models,Decoding,Task analysis,Estimation,Autonomous vehicles,intention estimation,trajectory prediction,Transformer
AI 理解论文
溯源树
样例
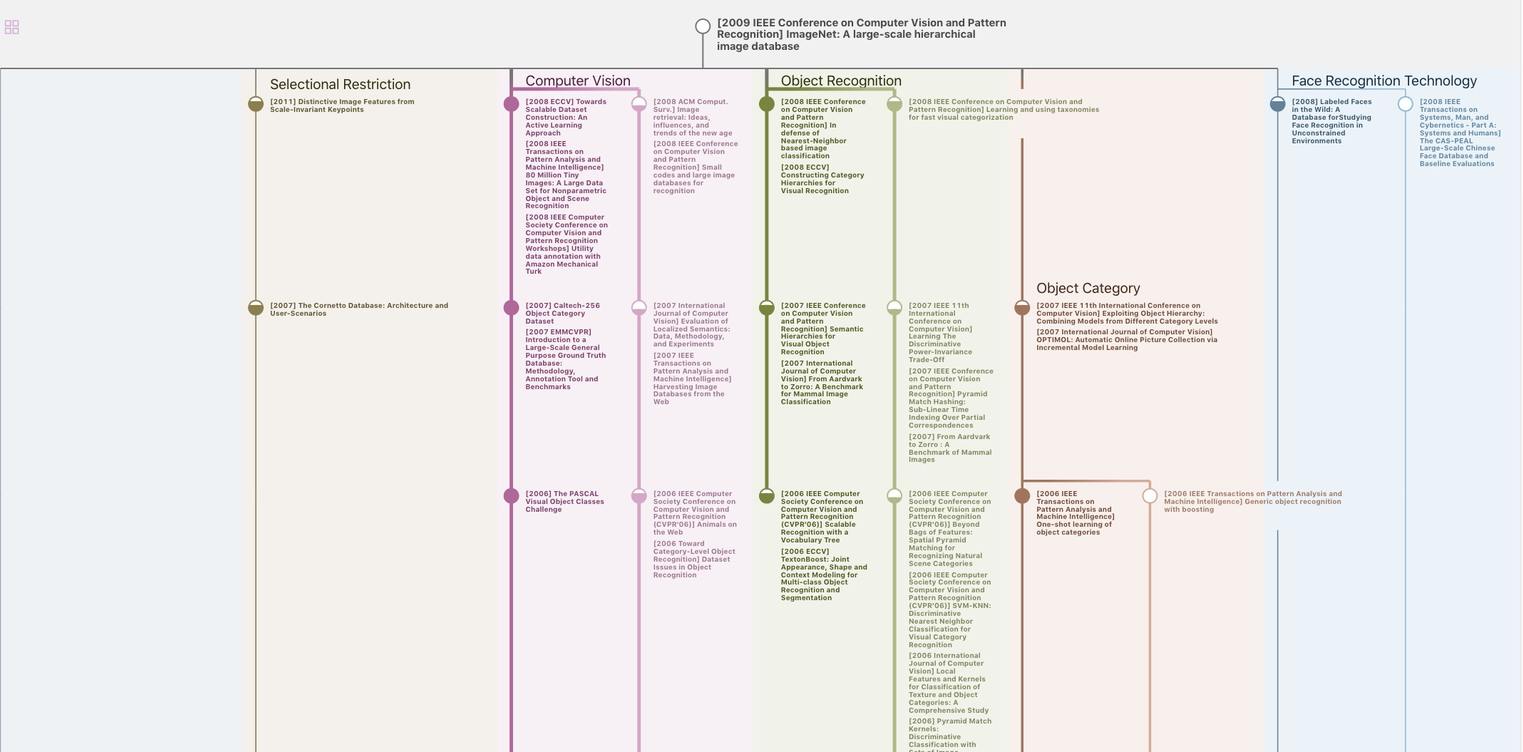
生成溯源树,研究论文发展脉络
Chat Paper
正在生成论文摘要