A Character Position-aware Compression Framework for Screen Text Content
IEEE Transactions on Circuits and Systems for Video Technology(2024)
摘要
With the increasing popularity of screen-sharing applications, efficient encoding of text content has become even more crucial. Text patterns typically exhibit distinct boundaries and sparse color histograms. However, in current hybrid codec frameworks, the positions of coding units are often misaligned with the text patterns, resulting in prediction and color mapping tools consuming a large number of bits to indicate these patterns. Nowadays, some text detection and recognition methods have been proposed to accurately locate and analyze the text regions in screen images. Combined with these techniques, we propose a character position-aware compression framework for screen text content. On the encoder side, a low-complexity detection method is adopted to locate the text characters. Then it copies the detected characters to the position aligned with the coding unit (CU) grid to form a text layer. This text-layer representation can further increase the efficiency of existing screen content coding tools such as Intra Block Copy (IBC). Moreover, we design several compression tools based on this representation. We extend the two Motion Vector (MV) prediction modes: Adaptive Motion Vector Prediction (AMVP) and Merge. We modify the MV encoding syntax according to the layout characteristics of the text layer. We present a Gradient-guided In-loop Filter (GIF) to sharpen the text lines using a convolutional network. Experiments on HEVC-SCC reference software HM-SCM intra_main_scc configuration show that the proposed framework can achieve an average bitrate savings of 9.5% and 8.5% under w/ GIF and w/o GIF versions, with 113% and 16% additional CPU encoding complexity, respectively.
更多查看译文
关键词
Screen content coding,Text detection,Motion vector prediction and coding,In-loop filter
AI 理解论文
溯源树
样例
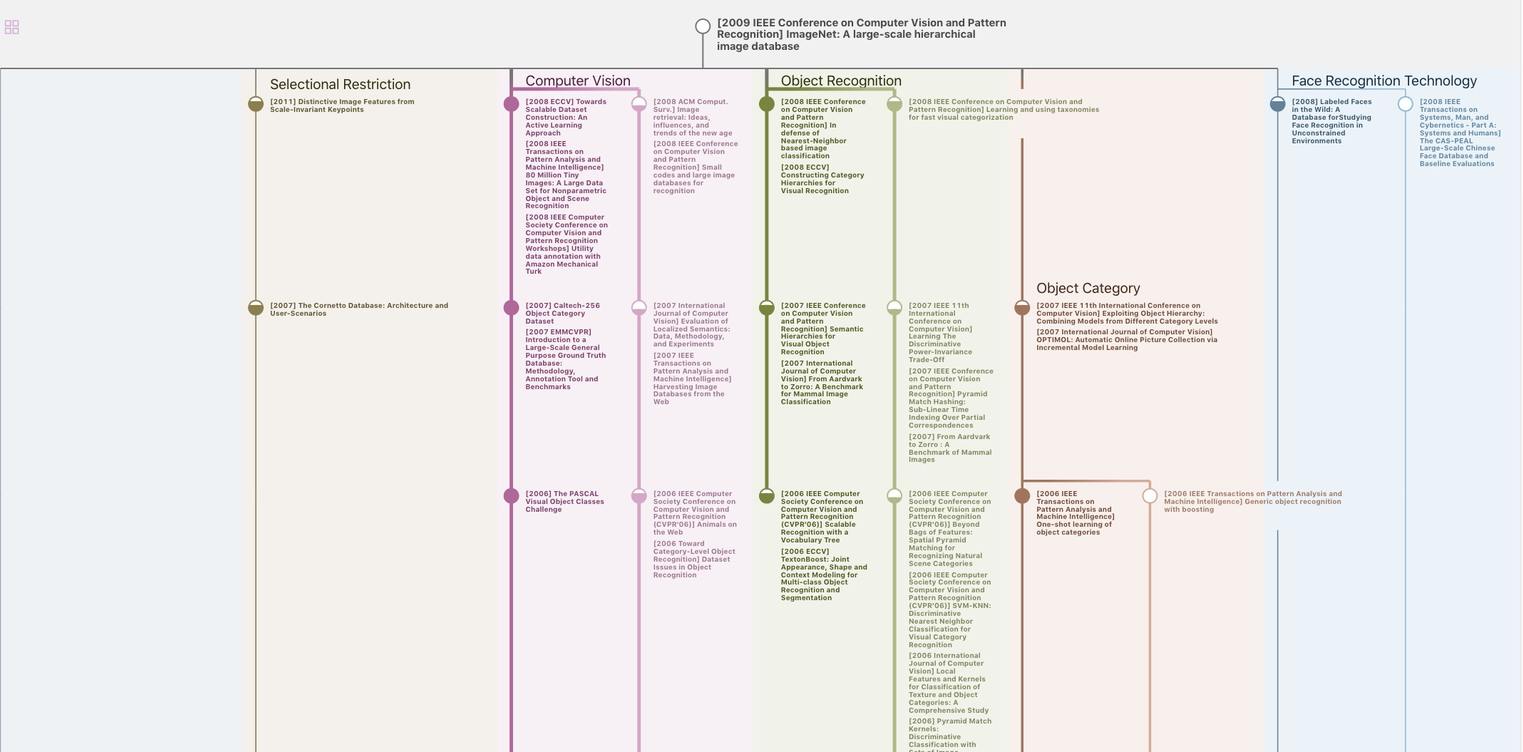
生成溯源树,研究论文发展脉络
Chat Paper
正在生成论文摘要