Optimal control for continuous-time Markov jump singularly perturbed systems : A hybrid reinforcement learning scheme
Journal of the Franklin Institute(2024)
摘要
This article discusses the adaptive optimal control problem for continuous-time Markov jump singularly perturbed systems with unknown system dynamics. First, the subsystems transformation technique is introduced to reconstruct the system with stochastic jump characteristics, yielding a set of parallel subsystems. Next, under the framework of reinforcement learning, an offline model-based hybrid iteration algorithm is developed to approximate the solution of the full-order coupled algebraic Riccati equations. Following this, to escape from the system model constraints, an online model-free hybrid iteration algorithm is introduced and relevant convergence proof is given. Compared to traditional value iteration and policy iteration algorithms, the hybrid iteration algorithm has ideal convergence rate and eliminates the requirement of initial stabilizing control policy. At the end, the availability of the online algorithm is demonstrated by means of an operational amplifier circuit model as a simulation example.
更多查看译文
关键词
Markov jump systems,Singularly perturbed systems,Hybrid iteration algorithm,Reinforcement learning. statement of contribution
AI 理解论文
溯源树
样例
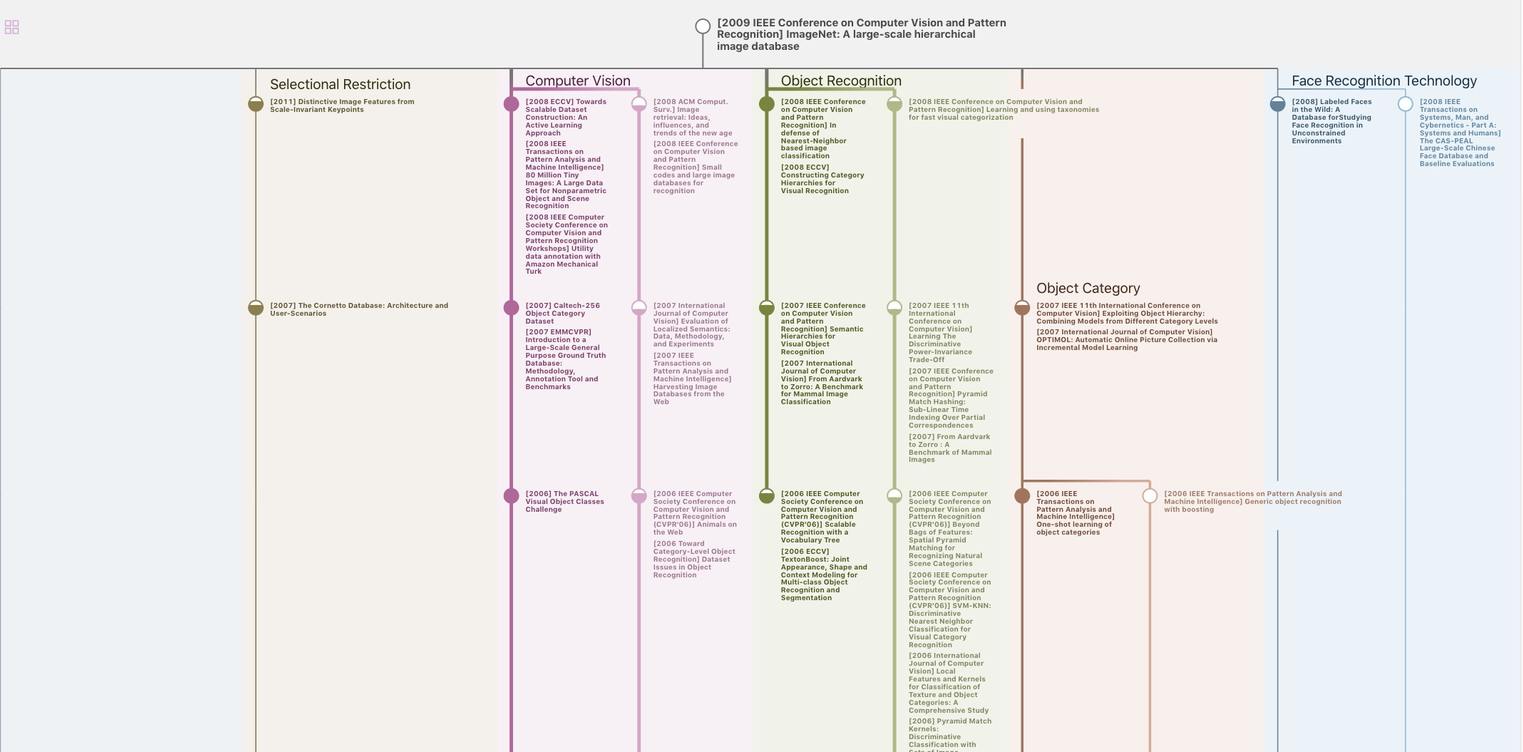
生成溯源树,研究论文发展脉络
Chat Paper
正在生成论文摘要