PCGNet: Point Cloud Generation Network for 3D Perception using Monocular Images and Radar
IEEE Transactions on Intelligent Vehicles(2024)
摘要
Millimeter-wave (mmWave) radar is widely used in autonomous driving thanks to its robustness under harsh weather conditions. However, compared to LiDAR point clouds, mmWave radar point clouds tend to be sparse and include several “ghost” targets. Existing methods attempt to optimize radar signal processing to generate superior radar point cloud. Nonetheless, these methods are primarily designed for simple scenarios. How to obtain superior radar point clouds for complex autonomous driving scenarios is still unexplored. In this article, we propose a novel point cloud generation network that generates superior radar point clouds by utilizing complementary advantage of radar and camera. Technically, our approach comprises three key steps. First, to obtain precise relationships between neighboring pixels, the mmWave range-azimuth map (RAM) is enhanced to extract the radar affinity matrix. Second, to obtain dense image bird's-eye-view (BEV) features, image features are diffused by learning local details from the radar affinity matrix. Third, to obtain superior radar point clouds, radar features and diffused image features are combined in a unified BEV representation, and optimized by K-nearest-neighbor (KNN) graph. To verify the effectiveness of the proposed methods, we collect a real-world dataset under autonomous driving scenarios to conduct experiments. The results demonstrate that our method outperforms exisiting ones in producing high-quality radar point clouds. And as a consequence, the radar point cloud generated using our methods significantly improve the performance of object detection task.
更多查看译文
关键词
Millimeter-wave(mmWave) radar,point clouds,sensor fusion,environment perception
AI 理解论文
溯源树
样例
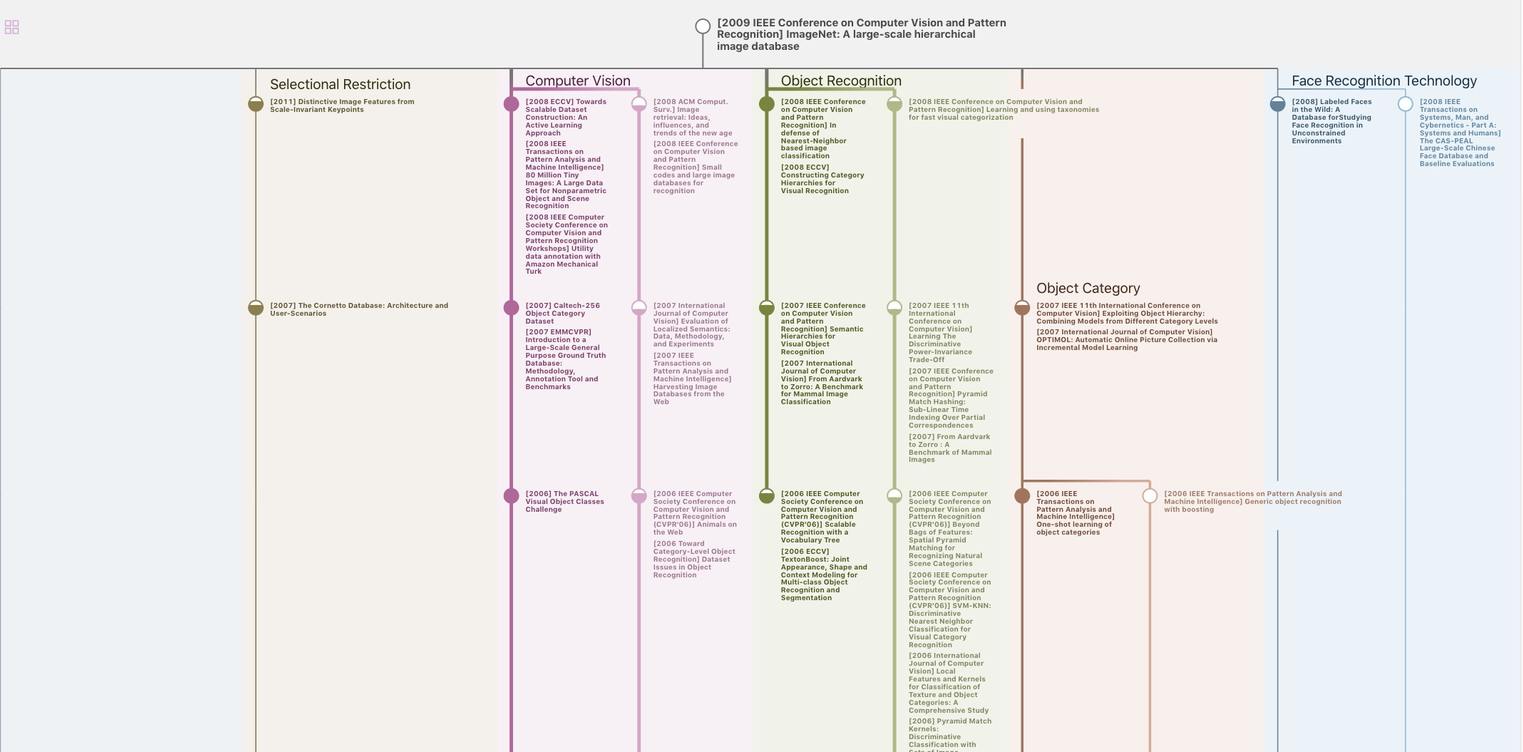
生成溯源树,研究论文发展脉络
Chat Paper
正在生成论文摘要