Regular decision processes
Artificial Intelligence(2024)
摘要
We introduce and study Regular Decision Processes (RDPs), a new, compact model for domains with non-Markovian dynamics and rewards, in which the dependence on the past is regular, in the language theoretic sense. RDPs are an intermediate model between MDPs and POMDPs. They generalize k-order MDPs and can be viewed as a POMDP in which the hidden state is a regular function of the entire history. In factored RDPs, transition and reward functions are specified using formulas in linear temporal logics over finite traces, or using regular expressions. This allows specifying complex dependence on the past using intuitive and compact formulas, and building models of partially observable domains without specifying an underlying state space.
更多查看译文
关键词
Markov-decision processes,Non-Markovian decision processes,POMDPs,Regular languages
AI 理解论文
溯源树
样例
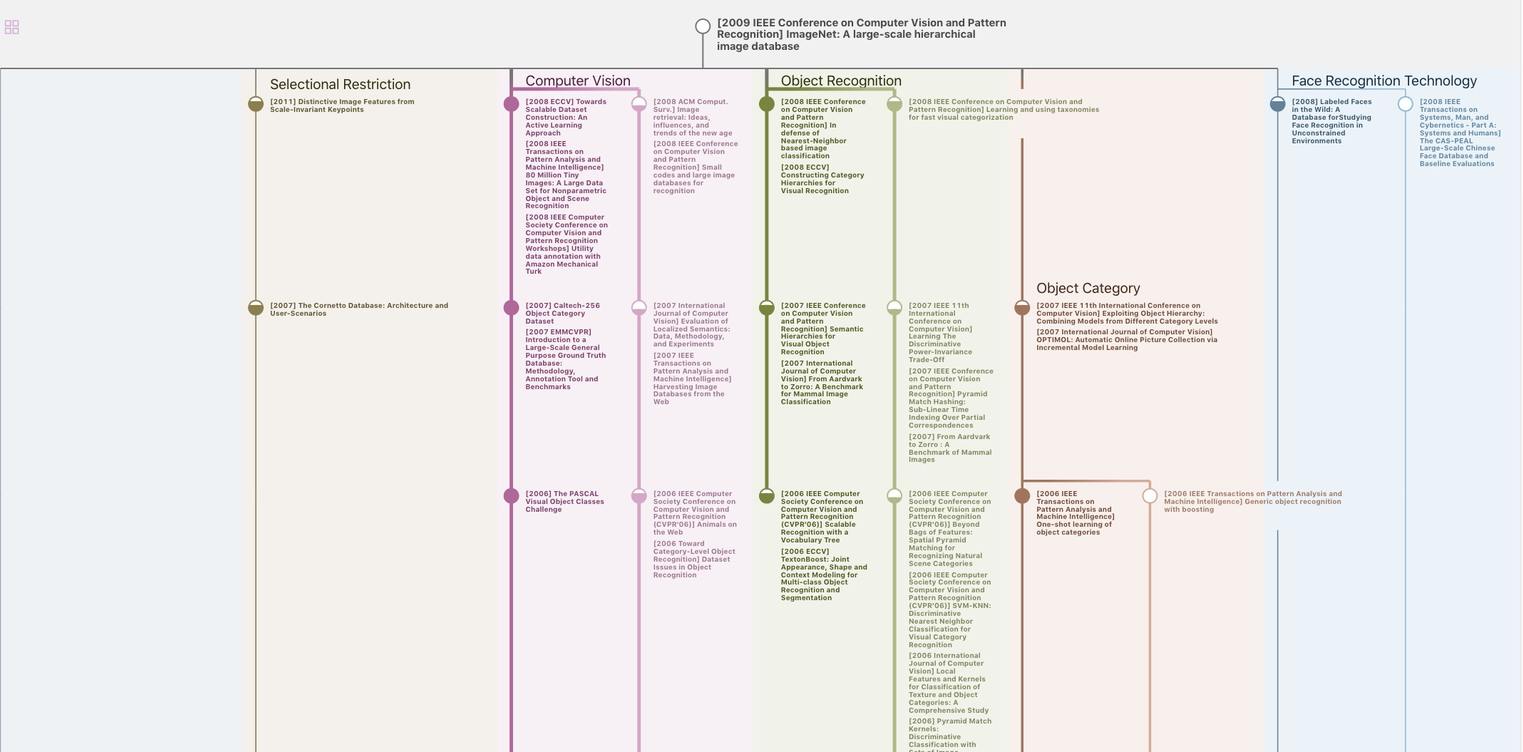
生成溯源树,研究论文发展脉络
Chat Paper
正在生成论文摘要