A survey on Concept-based Approaches For Model Improvement
arxiv(2024)
摘要
The focus of recent research has shifted from merely increasing the Deep
Neural Networks (DNNs) performance in various tasks to DNNs, which are more
interpretable to humans. The field of eXplainable Artificial Intelligence (XAI)
has observed various techniques, including saliency-based and concept-based
approaches. Concept-based approaches explain the model's decisions in simple
human understandable terms called Concepts. Concepts are human interpretable
units of data and are the thinking ground of humans. Explanations in terms of
concepts enable detecting spurious correlations, inherent biases, or
clever-hans. With the advent of concept-based explanations, there have been
various concept representation methods and automatic concept discovery
algorithms. Some recent methods use concepts for post-hoc model disentanglement
evaluation, while others use them for ante-hoc training. The concept-based
approaches are new, with many representations coming up, and there is very
limited work on Concept-based Model improvement. We provide a systematic review
and taxonomy of various concept representations and their discovery algorithms
in DNNs, specifically in vision. We also provide details on concept-based model
improvement literature, which is the first to survey concept-based model
improvement methods.
更多查看译文
AI 理解论文
溯源树
样例
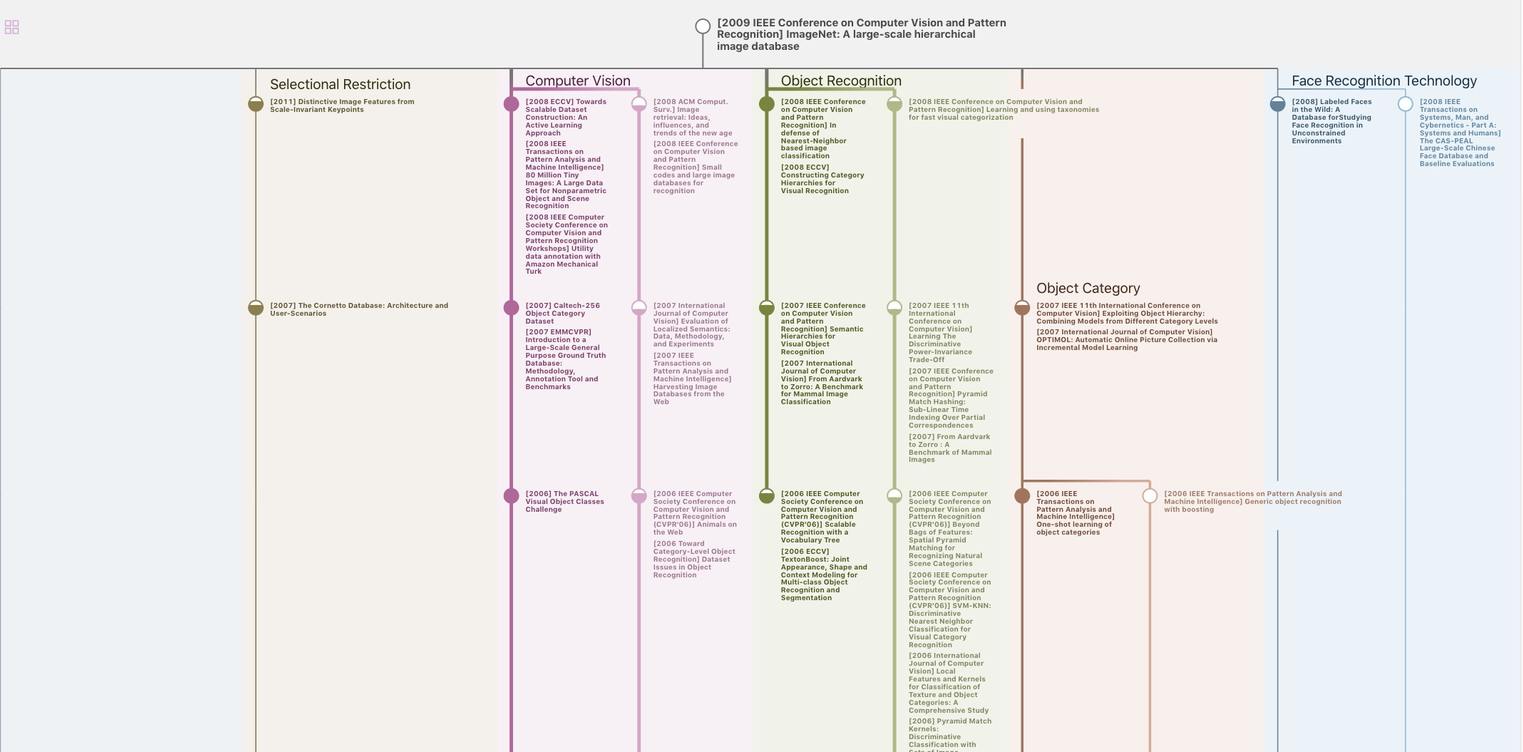
生成溯源树,研究论文发展脉络
Chat Paper
正在生成论文摘要