Evaluating the impact of instrumental variables in propensity score models using synthetic and negative control experiments
arxiv(2024)
摘要
In pharmacoepidemiology research, instrumental variables (IVs) are variables
that strongly predict treatment but have no causal effect on the outcome of
interest except through the treatment. There remain concerns about the
inclusion of IVs in propensity score (PS) models amplifying estimation bias and
reducing precision. Some PS modeling approaches attempt to address the
potential effects of IVs, including selecting only covariates for the PS model
that are strongly associated to the outcome of interest, thus screening out
IVs. We conduct a study utilizing simulations and negative control experiments
to evaluate the effect of IVs on PS model performance and to uncover best PS
practices for real-world studies. We find that simulated IVs have a weak effect
on bias and precision in both simulations and negative control experiments
based on real-world data. In simulation experiments, PS methods that utilize
outcome data, including the high-dimensional propensity score, produce the
least estimation bias. However, in real-world settings underlying causal
structures are unknown, and negative control experiments can illustrate a PS
model's ability to minimize systematic bias. We find that large-scale,
regularized regression based PS models in this case provide the most centered
negative control distributions, suggesting superior performance in real-world
scenarios.
更多查看译文
AI 理解论文
溯源树
样例
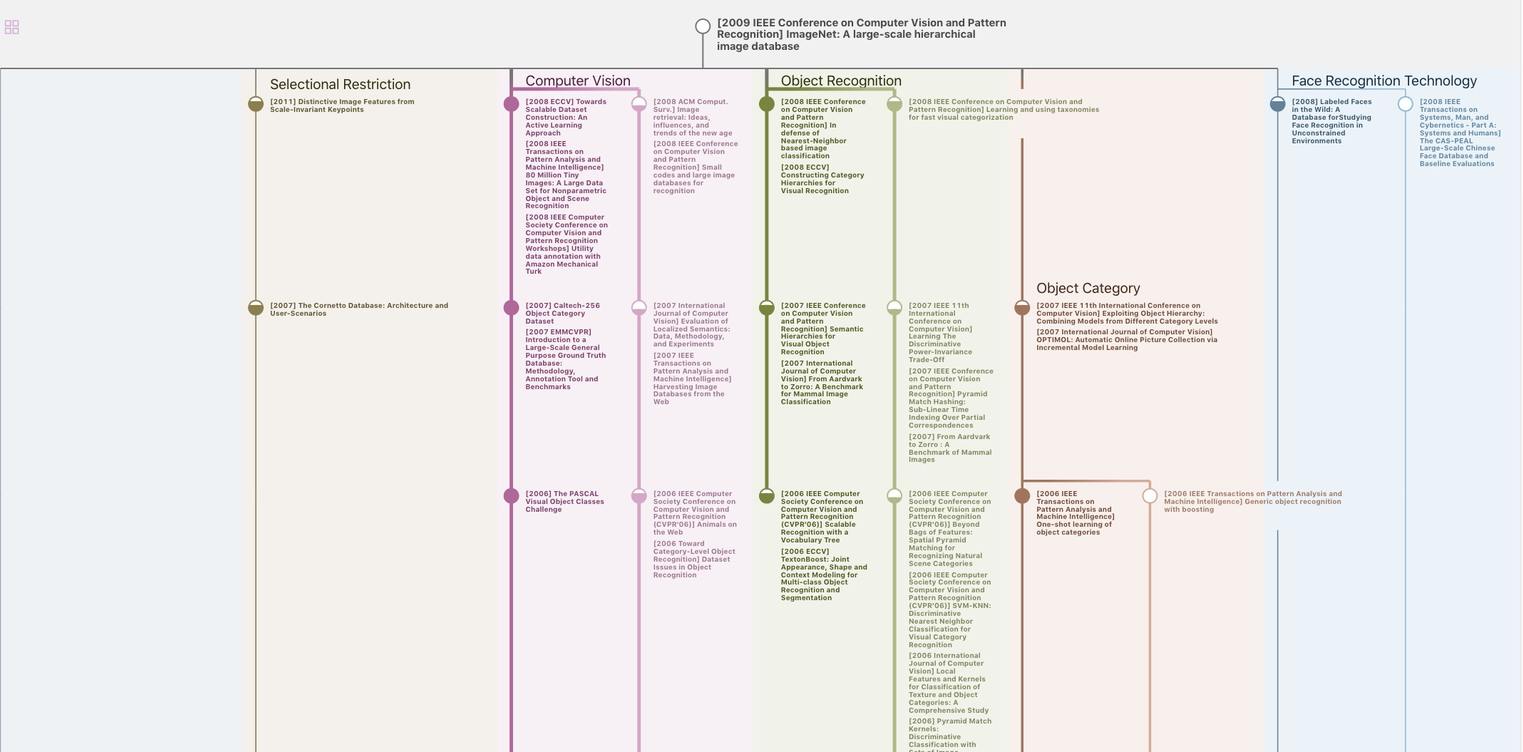
生成溯源树,研究论文发展脉络
Chat Paper
正在生成论文摘要