Analyzing the Variations in Emergency Department Boarding and Testing the Transferability of Forecasting Models across COVID-19 Pandemic Waves in Hong Kong: Hybrid CNN-LSTM approach to quantifying building-level socioecological risk
arxiv(2024)
摘要
Emergency department's (ED) boarding (defined as ED waiting time greater than
four hours) has been linked to poor patient outcomes and health system
performance. Yet, effective forecasting models is rare before COVID-19, lacking
during the peri-COVID era. Here, a hybrid convolutional neural network
(CNN)-Long short-term memory (LSTM) model was applied to public-domain data
sourced from Hong Kong's Hospital Authority, Department of Health, and Housing
Authority. In addition, we sought to identify the phase of the COVID-19
pandemic that most significantly perturbed our complex adaptive healthcare
system, thereby revealing a stable pattern of interconnectedness among its
components, using deep transfer learning methodology.
Our result shows that 1) the greatest proportion of days with ED boarding was
found between waves four and five; 2) the best-performing model for forecasting
ED boarding was observed between waves four and five, which was based on
features representing time-invariant residential buildings' built environment
and sociodemographic profiles and the historical time series of ED boarding and
case counts, compared to during the waves when best-performing forecasting is
based on time-series features alone; and 3) when the model built from the
period between waves four and five was applied to data from other waves via
deep transfer learning, the transferred model enhanced the performance of
indigenous models.
更多查看译文
AI 理解论文
溯源树
样例
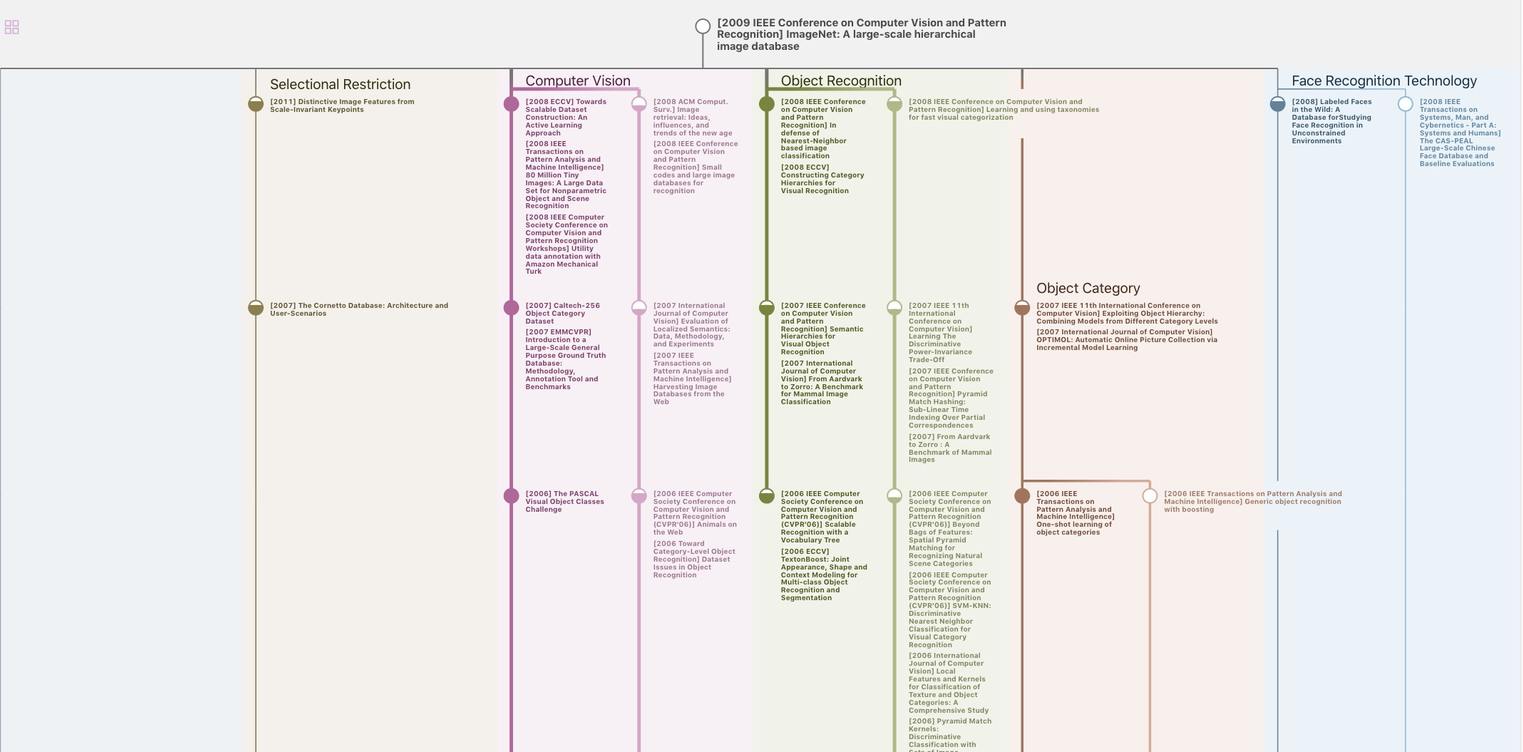
生成溯源树,研究论文发展脉络
Chat Paper
正在生成论文摘要