Joint Optimization of Training Job Placement and SFC Orchestration for ML Services in ZTN.
GLOBECOM (Workshops)(2023)
摘要
Machine learning (ML) services are vital to providing timely intelligent decision-making capabilities in Zero-touch Net-work (ZTN). However, such a service relies on efficient distributed ML model training which adds overheads in the perspectives of computing and network. A huge amount of model parameters are to be calculated by distributed ML training jobs and exchanged by reliable network service function chains (SFC), which require balanced computing loads and increase bandwidth consumption. It is thus important to optimize the problems of training job placement and SFC orchestration to increase the efficiency of model training and reduce bandwidth consumption. Since both mentioned issues are related, it is necessary to study both problems simultaneously. In this paper, an integer linear problem model is formulated to jointly optimize both problems. We then propose a heuristic algorithm to optimize training job load balancing rate and network resource consumption. We evaluate the performance of our algorithm on realistic Google cluster-usage traces with results showing that our algorithm is 18 times faster than the traditional algorithm. Our study shows that our proposed algorithm achieves near-optimal with no exceeding 3% from the optimal performance.
更多查看译文
关键词
ZTN,SFC orchestration,training job placement
AI 理解论文
溯源树
样例
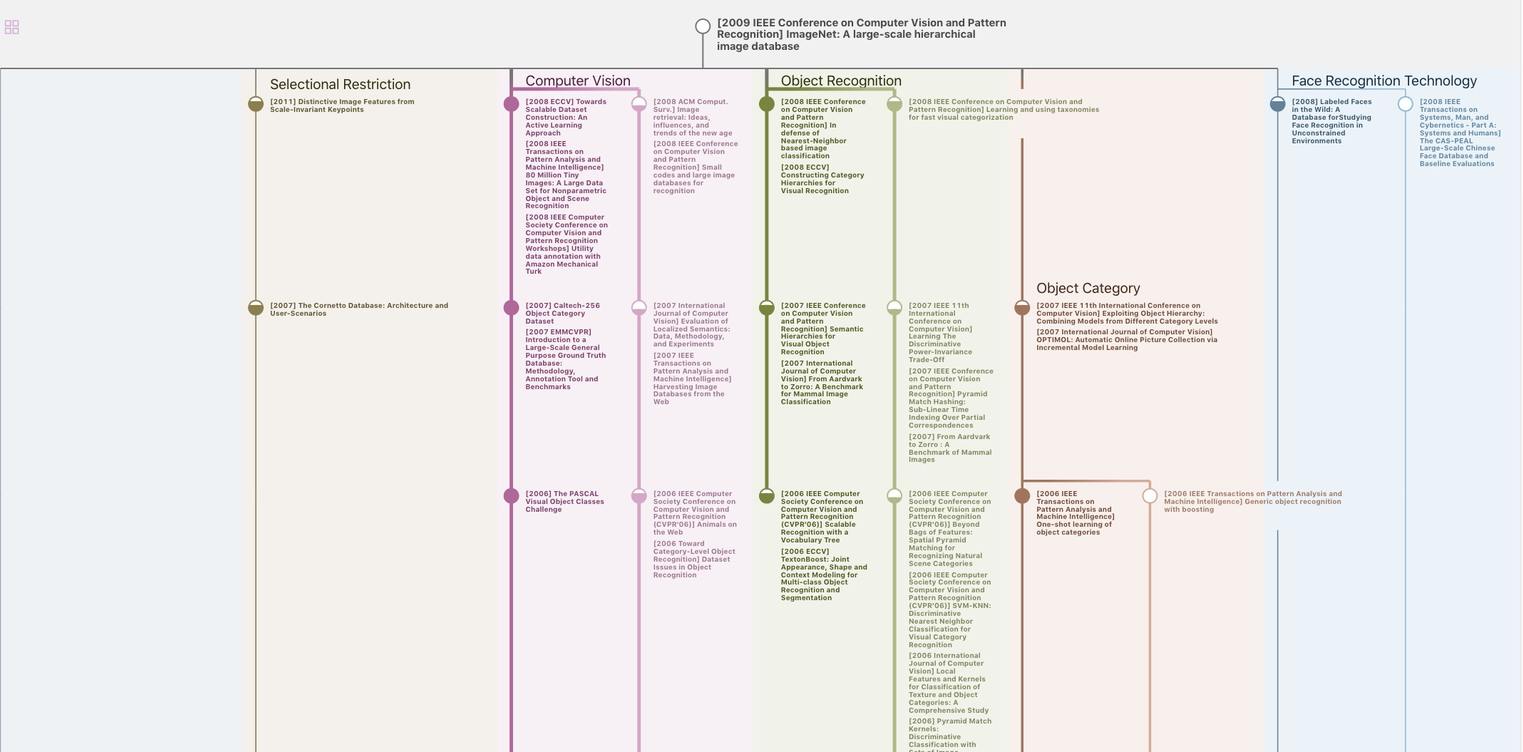
生成溯源树,研究论文发展脉络
Chat Paper
正在生成论文摘要