Lossy Lempel-Ziv Coding for Federated Learning.
GLOBECOM (Workshops)(2023)
摘要
Due to its privacy-preserving and promising learning performance, federated learning (FL) is widely used for distributed model training. However, the limited communication resources will severely slow down the training processing of FL. To address this issue, this paper, for the first time, applies Lempel-Ziv (LZ) coding into the FL system. It proposes a lossy LZ coding strategy that compresses the local model but allows a certain level of distortion. The convergence analysis shows that compared to the quantized stochastic gradient descent (QSGD), the lossy LZ coding further compresses the local models while maintaining the same sub-linear convergence performance when the distortion is relatively small. Another merit of the lossy LZ coding is that it can perform as a secondary compression after quantization or sparsification and thus is easy to implement. Experimental results demonstrate that it can significantly reduce the communication overhead than QSGD and achieve higher compression efficiency than Top-k sparsification under the same communication overhead.
更多查看译文
关键词
Federated learning,lossy compression,Lempel-Ziv
AI 理解论文
溯源树
样例
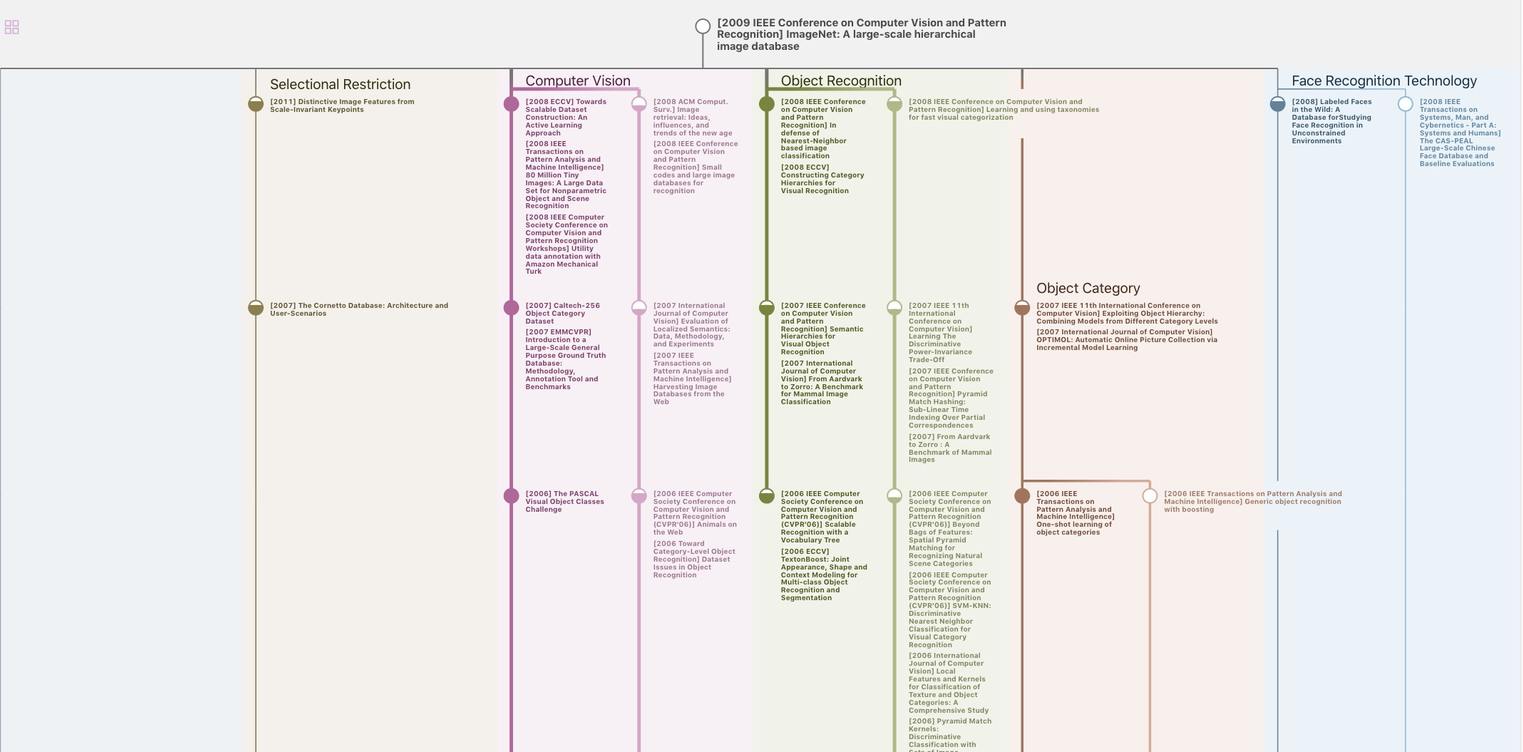
生成溯源树,研究论文发展脉络
Chat Paper
正在生成论文摘要