Lite-Agro 2.0: Integrating Federated and TinyML in Pear Disease Classification IoAT-Edge AI.
International Symposium on Smart Electronic Systems(2023)
摘要
The success of Tiny Machine Learning (TinyML) is dependent on the process of the model training, along with optimized algorithms and quality image dataset. In the process of Pear disease identification through tree leaf analysis, the need to protect the privacy of protected data has led to the topic of Federated Learning (FL). Prior Lite-Agro 1.0 study aims to apply Neural Network trained Deep Learning models on lightweight MCUs. With this second Lite-Agro 2.0 expansion, we explore different models, the integration of Federated Averaging (FedAvg) algorithm and explore decentralized training of models on multiple client devices with an emphasis on preserving data privacy. In federated learning, the data does not leave the client device and individual weights are passed on and aggregated onto a global server which then generates a global model. This federated learning research benchmarks frameworks such as Flower. Dev, model performance, and tabulates training values such as accuracy and loss given various FedAvg algorithms.
更多查看译文
关键词
Smart Agriculture,Agriculture Cyber-Physical System (A-CPS),Internet of Agro Things (IoAT),Smart Village,TinyML,Edge-AI,Federated Learning,Data Privacy and Security,Plant Health and Plant Disease
AI 理解论文
溯源树
样例
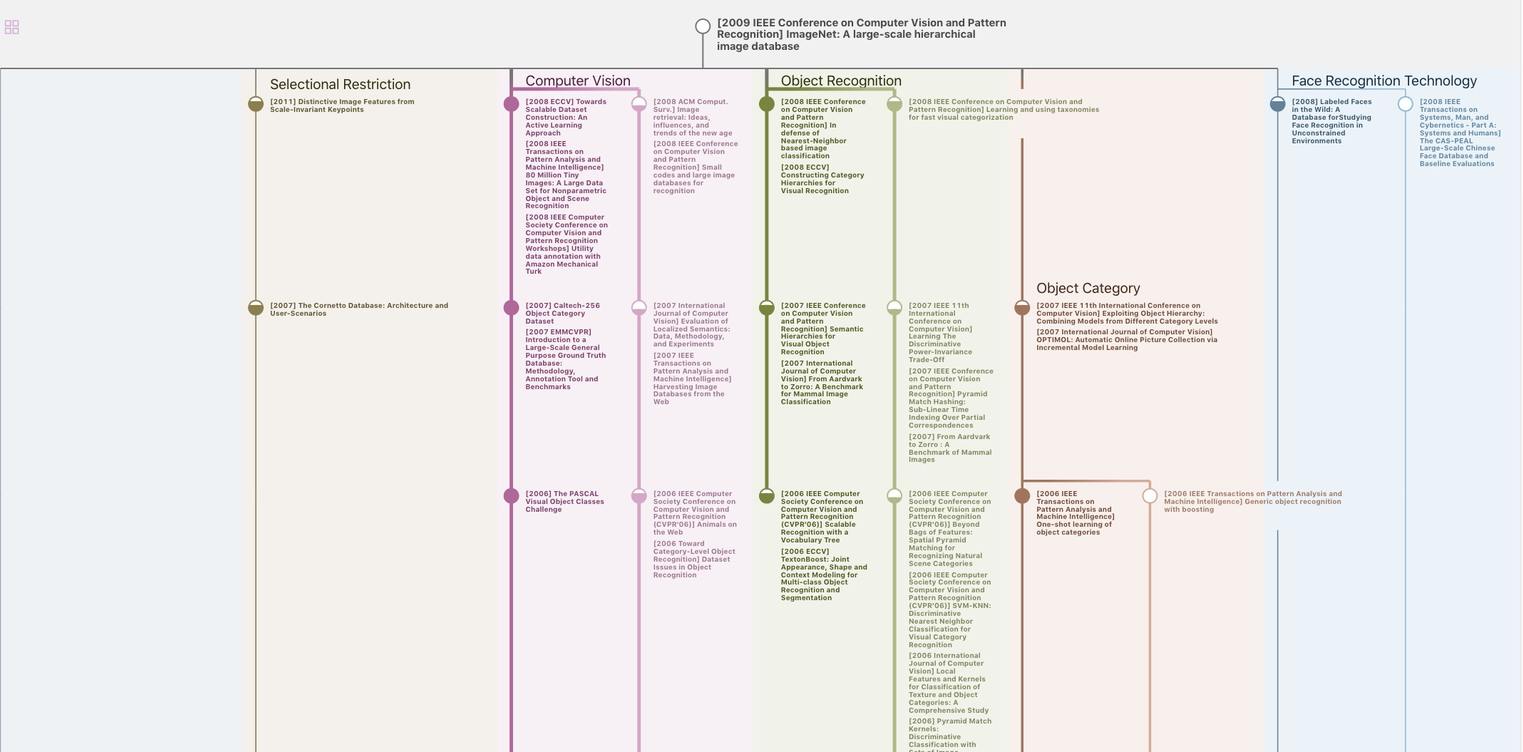
生成溯源树,研究论文发展脉络
Chat Paper
正在生成论文摘要