Prediction of ash content in coal slime flotation based on CNN-BP method with residual estimation
INTERNATIONAL JOURNAL OF COAL PREPARATION AND UTILIZATION(2024)
摘要
Ash content is a key metric for assessing coal slime flotation performance. Traditional methods of sampling, combustion, and weighing lead to measurement delays, thus image-based real-time ash prediction during flotation has become meaningful. However, the complex characteristics of the multiphase system in flotation pulp images make the extraction of an effective dataset for ash recognition challenging. This paper introduces an image-based method for predicting ash content in solid-liquid pulp mixtures using Convolutional Neural Networks (CNNs). A CNN transfer learning method based on ImageNet is proposed, combined with a Backpropagation (BP) residual estimation module with specified feature filters, reducing the sample size requirement. Four classic CNN networks were utilized. Results indicate that introducing the BP residual estimation module improves the CNN network's prediction accuracy in small samples, with total residuals consistent with the white noise distribution. Notably, the 5% accuracy (absolute error less than 5%) of VGG19 without the CNN classic residual structure improved significantly, reflecting the superior residual estimation of BP and the robust performance of the CNN-BP in predicting pulp ash content. This research offers a new option for transitioning previous dataset collection methods for ash detection in the coal slime flotation process.
更多查看译文
关键词
Coal flotation,ash content,CNN,residual estimation,transfer learning,small datasets
AI 理解论文
溯源树
样例
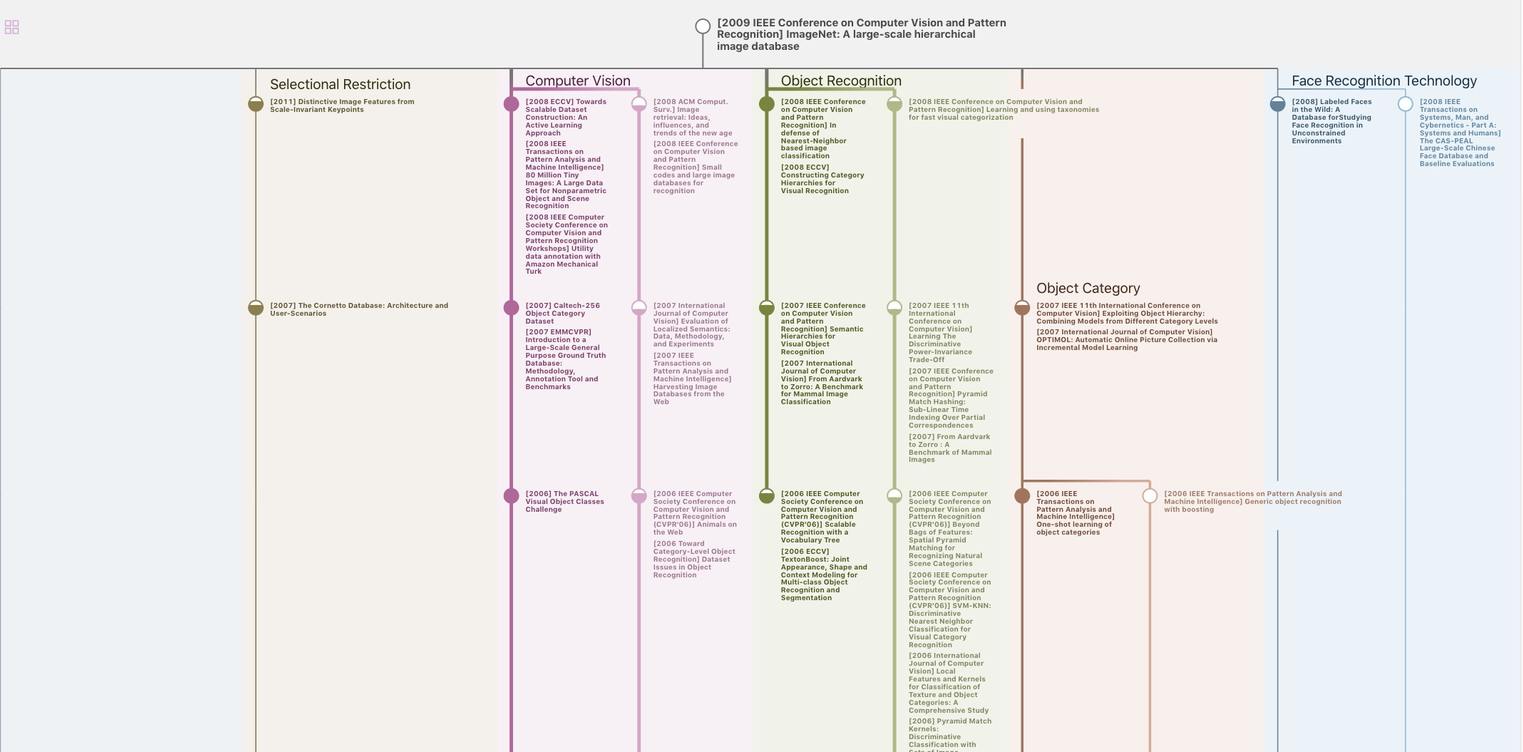
生成溯源树,研究论文发展脉络
Chat Paper
正在生成论文摘要