Reward-Driven Clustered Federated Learning for DRL Based UAV-RIS Networks
2023 IEEE 8th International Conference on Recent Advances and Innovations in Engineering (ICRAIE)(2023)
摘要
Deep reinforcement learning (DRL) has empowered numerous reconfigurable intelligent surface (RIS) aided millimeter-wave unmanned aerial vehicle (UAV) communications. However, most existing works often employ single-task DRL, that could only handle one specific environment (or task). This hurdles the large-scale adoption of DRL-based UAV-RIS networks, since the environment has infinite variations. In this paper, we aim to solve diverse UAV-RIS tasks with a minimum number of DRLs required. Specifically, we proposed a reward-driven clustering technique to autonomously cluster all 50 simulated tasks into 10 clusters. For each cluster, we train a multi-task DRL using Federated Learning to handle all tasks in the cluster. Simulation shows that given 50 unique tasks, the proposed method could reduce the total agents required from 50 to 10, which is a 5-fold reduction.
更多查看译文
AI 理解论文
溯源树
样例
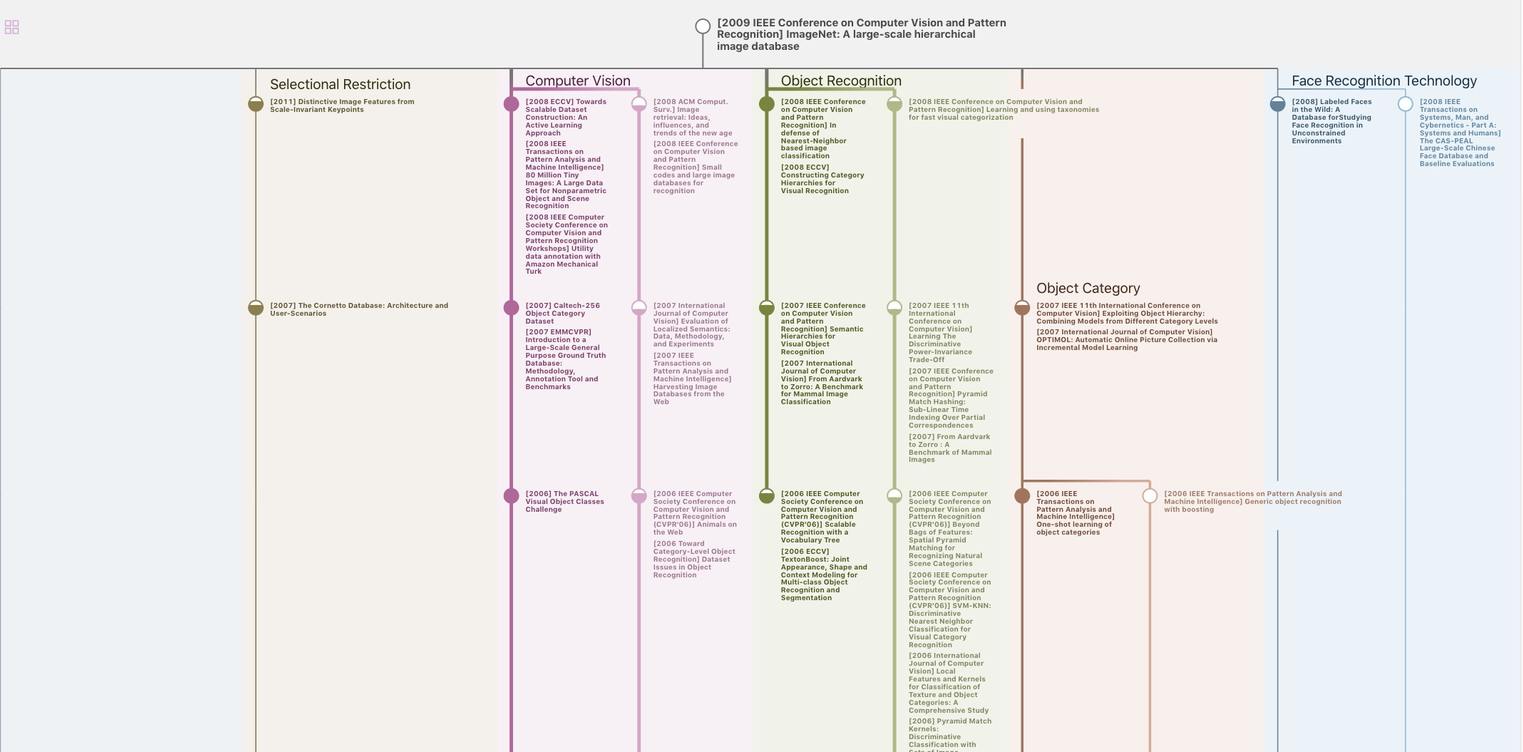
生成溯源树,研究论文发展脉络
Chat Paper
正在生成论文摘要