Univariate Time Series Data Correction Method using LSTM Autoencoder with Temporal Distance.
International Conference on Artificial Intelligence in Information and Communication(2024)
摘要
High-quality data is essential to increase the reliability of machine learning-based prediction models. For time series data, anomalies significantly reduce the accuracy of prediction models. In this paper, we propose a novel time series data correction method that converts abnormal values of univariate time series data into normal ones. For anomaly detection and correction, we utilize the LSTM Autoencoder model, where we propose a new weight function that considers temporal distance. Through experiments using the open NAB data, we show that our proposed method is superior to the recent conventional methods.
更多查看译文
关键词
time series data,LSTM Autoencoder,anomaly detection,data correction,deep learning,data quality
AI 理解论文
溯源树
样例
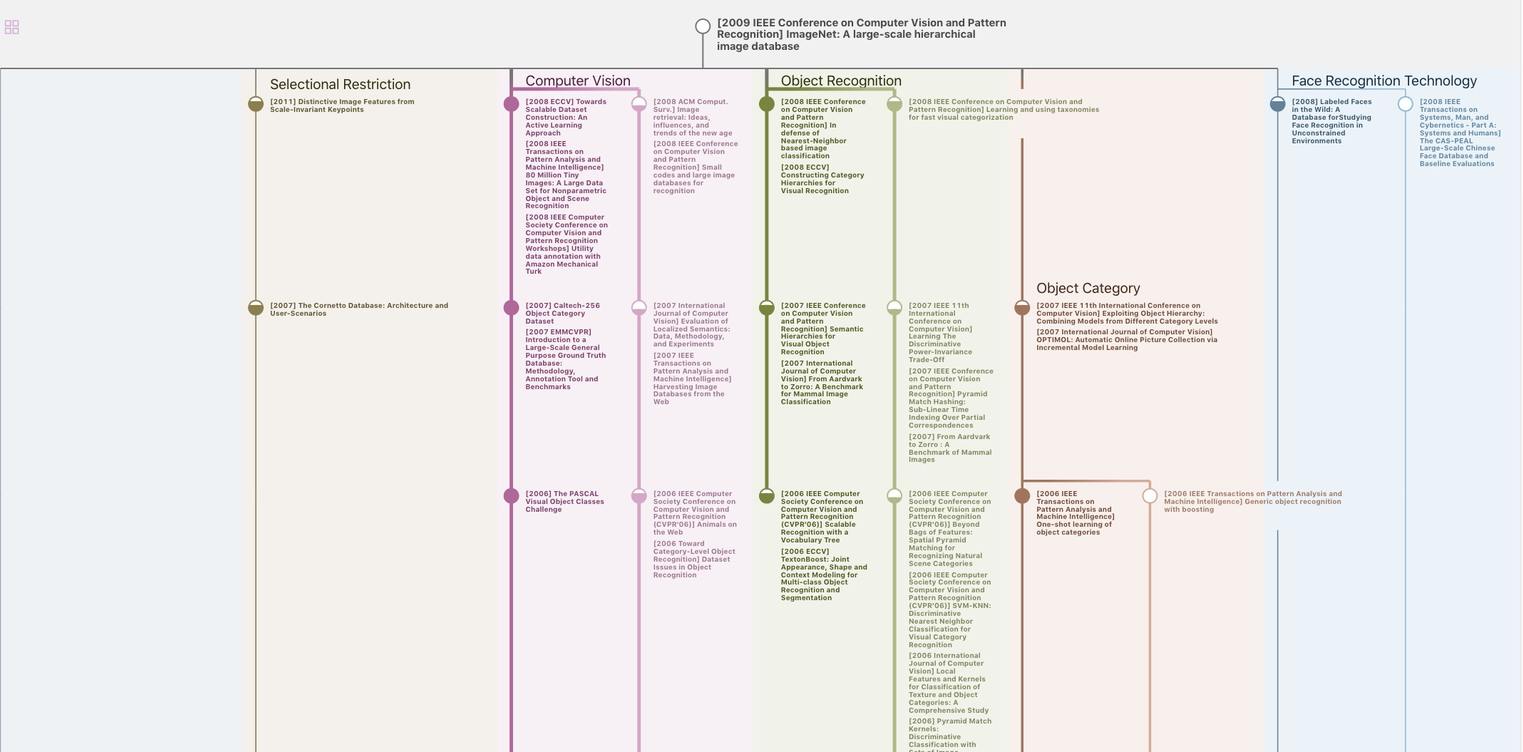
生成溯源树,研究论文发展脉络
Chat Paper
正在生成论文摘要