Efficient Automatic Annotation of Binary Masks for Enhanced Training of Computer Vision Models.
ISM(2023)
摘要
In modern computer vision models, the quality and quantity of training data have become crucial. Datasets deemed sufficient a few years ago now require data augmentation to increase their size. This presents a challenge, especially when these supplementary datasets lack annotations in standard formats like COCO, VGG, or YOLO. One solution to this problem is to learn semantic boundaries from binary images of unannotated datasets, thereby increasing the data available for training and evaluating models. However, choosing an efficient annotation method can be both time-consuming and effort-intensive. This research paper explores three approaches, ranging from traditional image processing algorithms to the recently introduced Segment Anything Model (SAM). The study demonstrates how these different algorithms perform on various datasets and concludes that the proposed image processing method strikes the best balance between performance and efficiency.
更多查看译文
关键词
Computer Vision,Data Annotation,Datasets
AI 理解论文
溯源树
样例
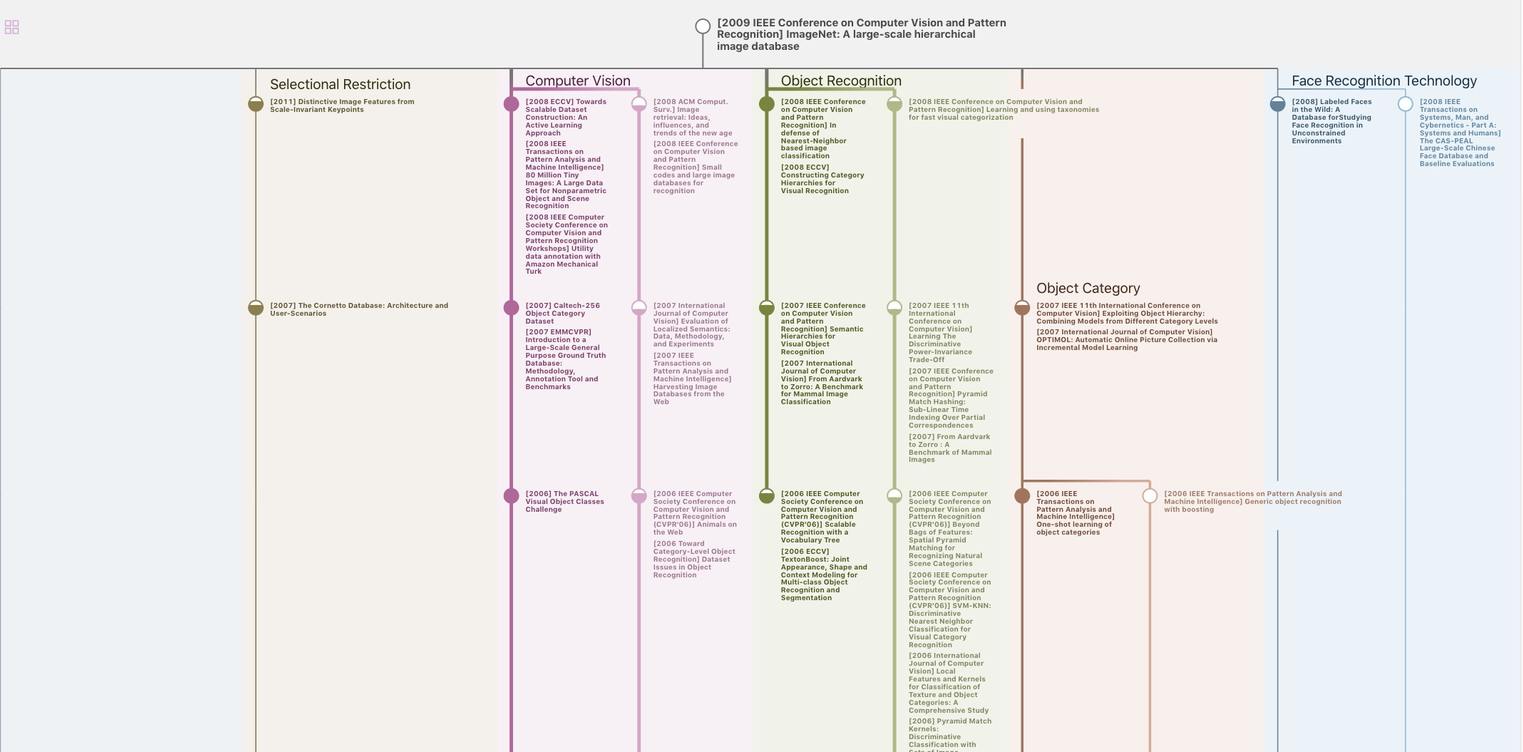
生成溯源树,研究论文发展脉络
Chat Paper
正在生成论文摘要