Query-focused Submodular Demonstration Selection for In-context Learning in Large Language Models
2023 31ST IRISH CONFERENCE ON ARTIFICIAL INTELLIGENCE AND COGNITIVE SCIENCE, AICS(2023)
摘要
The increase in dataset and parameter size of large language models has given rise to an emergent ability known as In-context Learning (ICL). This approach allows models to perform tasks based on human instructions and a few demonstration examples in a prompt. ICL differs from traditional fine-tuning methods by enabling the adaptation of pre-trained models to new tasks without modifying their core parameters or requiring gradient updates. Despite its potential, the intricacies of ICL, particularly the methods for choosing effective demonstration examples to enhance predictive performance, are not fully understood, with prior research often relying on random selection. Our research addresses this gap in two ways. Firstly, we advocate the use of query-focused submodular mutual information functions for selecting demonstration examples in ICL. These functions help identify examples that are both diverse and representative, thereby improving few-shot performance in comparison to random and zero-shot baselines. Our experiments validate this approach. Secondly, we introduce an interactive tool to explore the impact of hyperparameters on model performance. These parameters include the quantity and generation methods of demonstration examples, and their influence on data manifolds and clusters. Our results show that carefully chosen examples can lead to performance improvements of up to 20%. For instance, in sentiment classification, we observed an f1-score of 88.35% compared to 51.95%, and in topic classification, 90.56% versus 31.38%.
更多查看译文
关键词
In-context Learning,Visualization,Language Models,Submodular Optimization,Data Selection
AI 理解论文
溯源树
样例
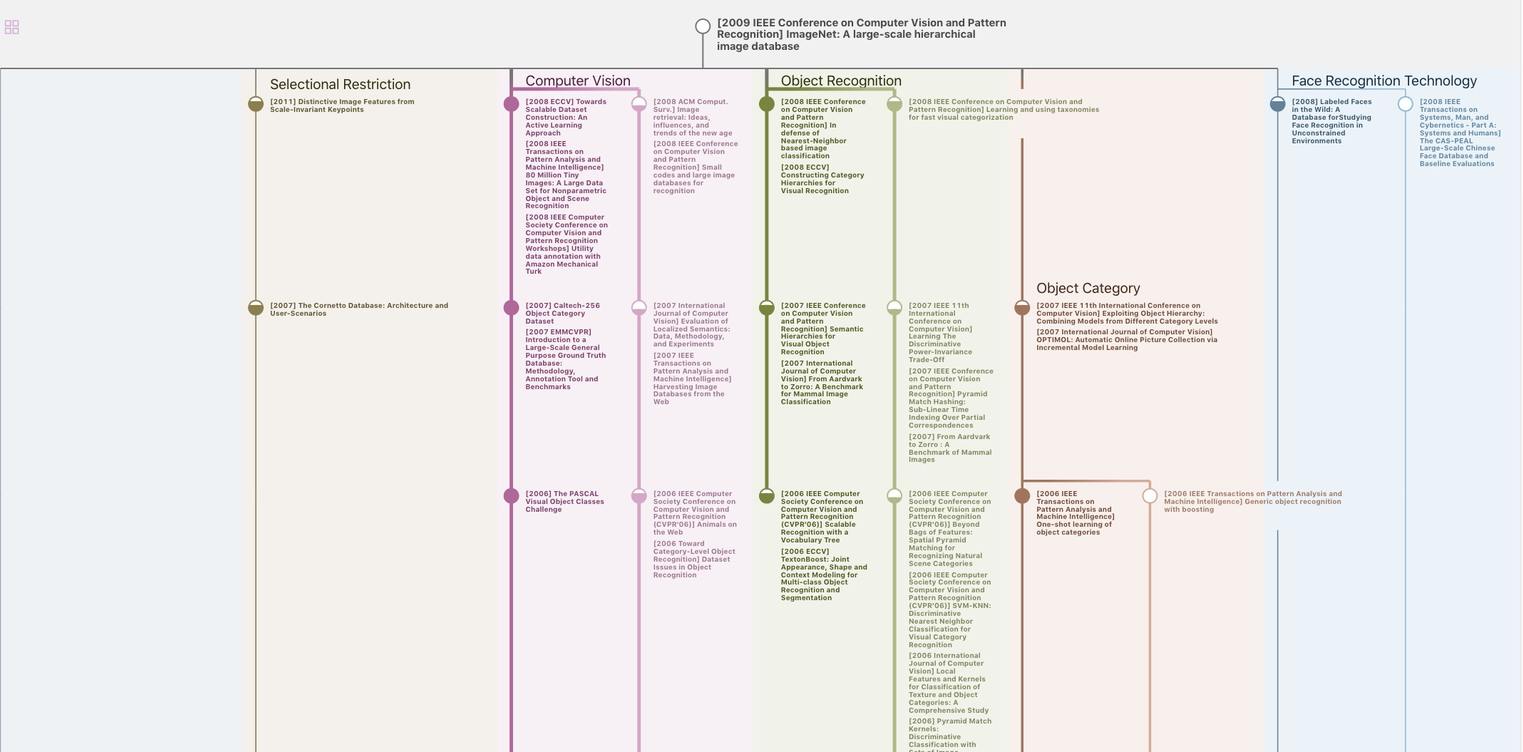
生成溯源树,研究论文发展脉络
Chat Paper
正在生成论文摘要