An Evolutionary Neural Architecture Search Method based on Performance Prediction and Weight Inheritance
Information Sciences(2024)
摘要
Evolutionary Neural Architecture Search (ENAS) algorithms attract great attention since they can automatically search for appropriate network architectures for a given task. However, most ENAS algorithms suffer from a prohibitive computational burden. Moreover, some of these approaches directly use performance predictors for evaluations, which may introduce inaccurate assessments and harm the evolution. To overcome these shortcomings, we propose an efficient ENAS algorithm named EPPGA. EPPGA employs a predictor to pre-select potentially high-performing offspring, enhancing the performance and accelerating the evolution. As the offspring will be further accurately evaluated, even potentially inaccurate predictions will not adversely affect the evolution. Furthermore, a weight inheritance method is suggested to accelerate the evaluation, and new genetic operations are developed to produce offspring that share a substantial proportion of beneficial genetic materials with one parent, improving the performance predictor's effectiveness and promoting weight inheritance. Finally, a new efficient backbone block structure is designed to facilitate the search for lightweight networks. The experimental results demonstrate that EPPGA is a highly competitive algorithm on three benchmarks in terms of accuracy, model size, and computational cost, reveal the superiority of the proposed block structure, and confirm the effectiveness of the proposed performance predictor and weight inheritance method.
更多查看译文
关键词
Neural architecture search,Performance predictor,Weight inheritance,Image classification
AI 理解论文
溯源树
样例
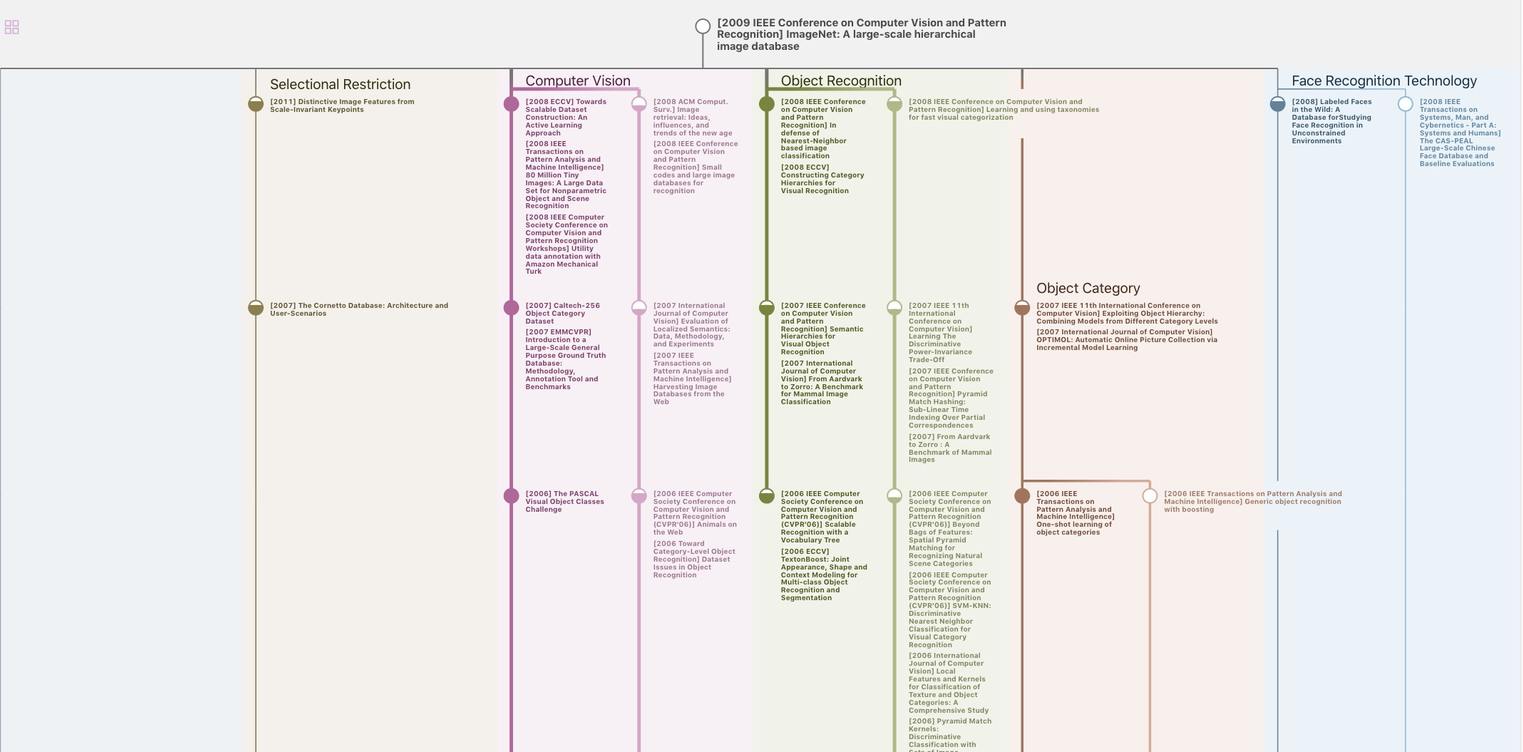
生成溯源树,研究论文发展脉络
Chat Paper
正在生成论文摘要