Domain-Adaptive HRRP Generation Using Two-Stage Denoising Diffusion Probability Model
IEEE GEOSCIENCE AND REMOTE SENSING LETTERS(2024)
摘要
High-resolution range profile (HRRP) plays a crucial role in radar target recognition. In real-world applications, variations in operational conditions during testing, such as changes in depression angles, resulting in unsatisfactory performance for HRRP target recognition methods. One way to alleviate this issue is to augment training data with samples that embody the testing domain style. Therefore, we propose a domain-adaptive HRRP generation approach based on a two-stage denoising diffusion probability model (DDPM). In the first stage, we leverage the category labels as conditioning factors, ensuring precise category control over the pregenerated contents. In the second stage, we harness style information from reference samples to steer the pregenerated content closer to the testing domain. By augmenting training data with the generated samples, the disparity between the two domains is bridged. Results on the moving and stationary target acquisition and recognition (MSTAR) dataset show that the proposed method improves the recognition rate by 2.01% and 4.93% for the data of 15(degrees) and 30(degrees) depression angle, respectively.
更多查看译文
关键词
Category-controllable generation,denoising diffusion probability model (DDPM),high-resolution range profile (HRRP),operation condition adaption
AI 理解论文
溯源树
样例
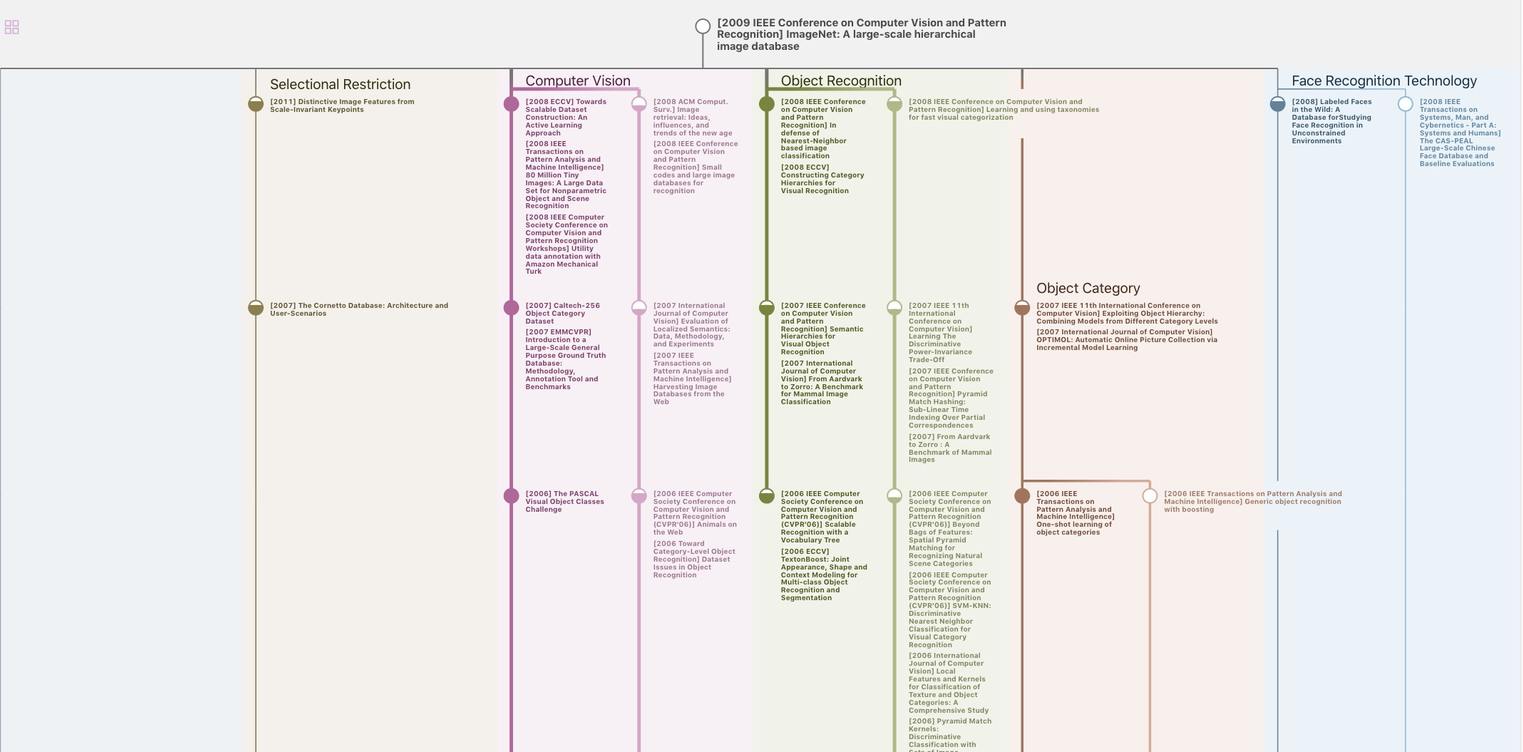
生成溯源树,研究论文发展脉络
Chat Paper
正在生成论文摘要