Hybrid Algorithm for Dynamic Fault Prediction of HVDC Converter Transformer Using DGA Data
IEEE Transactions on Dielectrics and Electrical Insulation(2024)
摘要
Very limited studies have forecasted the gas concentration and faults of high voltage direct current (HVDC) converter transformers. In this study, a hybrid algorithm consisting seasonal autoregressive integrated moving average-convolutional neural network-gated recurrent unit (SARIMA-CNN-GRU) was proposed to forecast the seven gas concentrations, i.e., hydrogen (H
2
), methane (CH
4
), ethane (C
2
H
6
), ethylene (C
2
H
4
), acetylene (C
2
H
2
), carbon-dioxide (CO
2
) and carbon monoxide (CO). Moreover, extreme gradient boost (XGBoost), adaptive boosting (AdaBoost), and light gradient boosting machine (LightGBM) were utilized to predict the fault inside the HVDC converter transformer. Two types of datasets collected from different sources were used to train the regression and classification models. The performance of the regression model was evaluated by root mean squared error and mean absolute error, whereas the performance of the classification model was evaluated by accuracy, precision, and recall. Finally, a comparative analysis was conducted to showcase the superiority of the proposed methodology.
更多查看译文
关键词
Classification,dissolved gas analysis,forecasting,machine learning,oil-immersed transformer
AI 理解论文
溯源树
样例
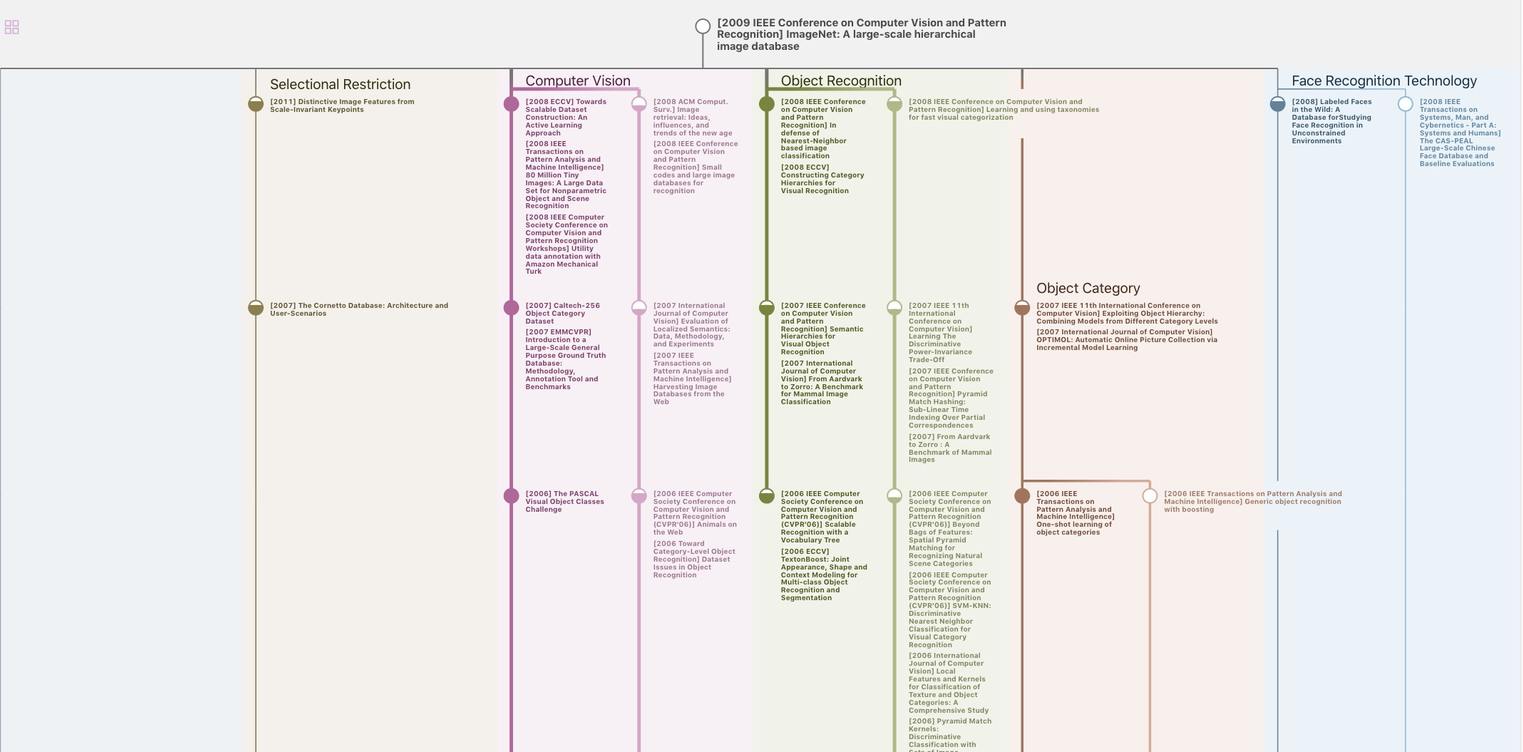
生成溯源树,研究论文发展脉络
Chat Paper
正在生成论文摘要