End-to-End MIMO Systems with Conditional Generative Adversarial Networks.
International Conference on Artificial Intelligence in Information and Communication(2024)
摘要
Conventional autoencoder-based systems, comprising a neural-network encoder at the transmitter and a neural-network decoder at the receiver, often face limitations in re-alistic signal transmission due to its reliance on differentiable channel models. In response to this limitation, an end-to-end framework has emerged, employing a conditional generative adversarial network (CGAN) for channel learning. The CGAN not only learns to represent channel effects through feature extraction but also facilitates gradient back-propagation between the receiver and the transmitter, thereby enhancing the system's adaptability. This paper extends the CGAN-based end-to-end system from single input single output channels to multiple input multiple output (MIMO) scenarios. This CGAN-based MIMO system demonstrates promising performance comparable to the autoencoder communication systems, highlighting the potential of CGANs as effective alternatives for original channels.
更多查看译文
关键词
Autoencoder,deep learning,generative adversarial network (GAN),multiple input multiple output (MIMO)
AI 理解论文
溯源树
样例
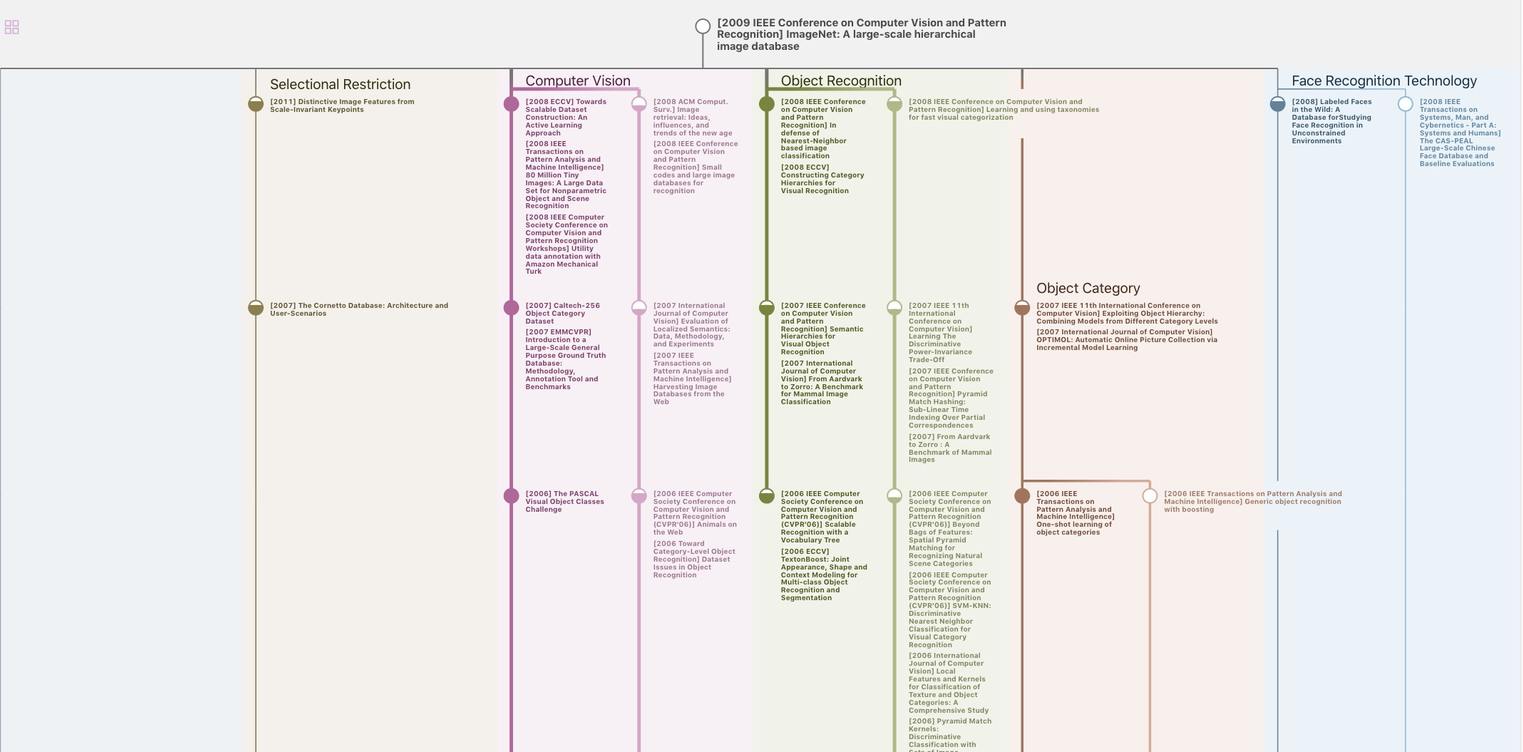
生成溯源树,研究论文发展脉络
Chat Paper
正在生成论文摘要