Efficient Few-Shot Classification Using Self-Supervised Learning and Class Factor Analysis.
International Conference on Artificial Intelligence in Information and Communication(2024)
摘要
Recently, significant advancements have been made in the few-shot classification task by integrating pre-trained self-supervised learning models. Although the self-supervised learning models have demonstrated their effectiveness, their application in few-shot scenarios, specifically in meta-training or fine-tuning, is computationally intensive and complicated. This paper introduces an efficient approach to address these challenges. We propose to use feature analysis methods instead of network model training. This method uses two factors that define the data generation model, resulting in easily classifiable features. The two factors are estimated from a set of different vectors from the train dataset and the test dataset. Although this method is simple compared to network learning, it provides good performance in experiments using few-shot classification benchmark datasets.
更多查看译文
关键词
Few-shot classification,Feature analysis,Class factor,Environment factor Self-supervised learning
AI 理解论文
溯源树
样例
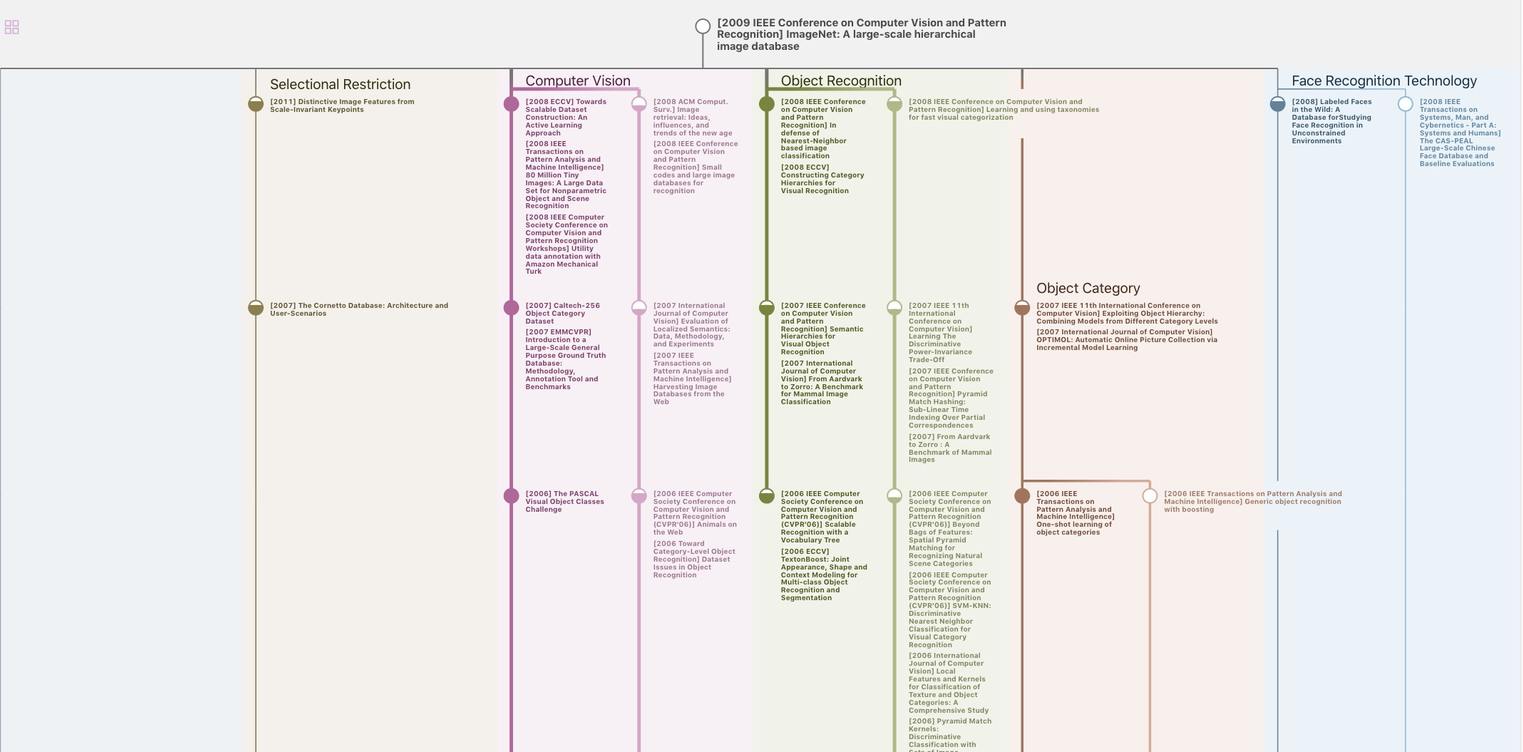
生成溯源树,研究论文发展脉络
Chat Paper
正在生成论文摘要